Toward a decision process of the best machine learning model for multi-stakeholders: a crowdsourcing survey method.
User Modeling, Adaptation, and Personalization (UMAP)(2022)
摘要
Fairness-aware machine learning (ML) technology has been developed to remove discriminatory bias, e.g., bias on race and gender. However, there are trade-offs between the metrics of accuracy and fairness in ML models, and different stakeholders prioritize these metrics differently. Hence, to form an agreement on prioritization, workshop approaches encouraging dialogue among stakeholders have been explored. However, it is practically difficult for multiple stakeholders to have conversations at the same place and time. We examined a method of extracting the prioritization of several stakeholders regarding certain metrics using an online survey. We randomly divided 739 crowdsourced participants into 4 stakeholder groups and asked them to rank 5 randomly selected ML models in terms of their metric prioritization. Through this survey, we calculated the prioritization of metrics of each stakeholder group and whether the information on three other stakeholders affects another stakeholder’s prioritization of metrics. With our method, the prioritization of each stakeholder successfully met the requirements of their role. However, metric prioritization is not affected by information on the other stakeholders. Furthermore, demographics and attitudes towards decision making scenarios affect each stakeholder’s metric prioritization differently.
更多查看译文
AI 理解论文
溯源树
样例
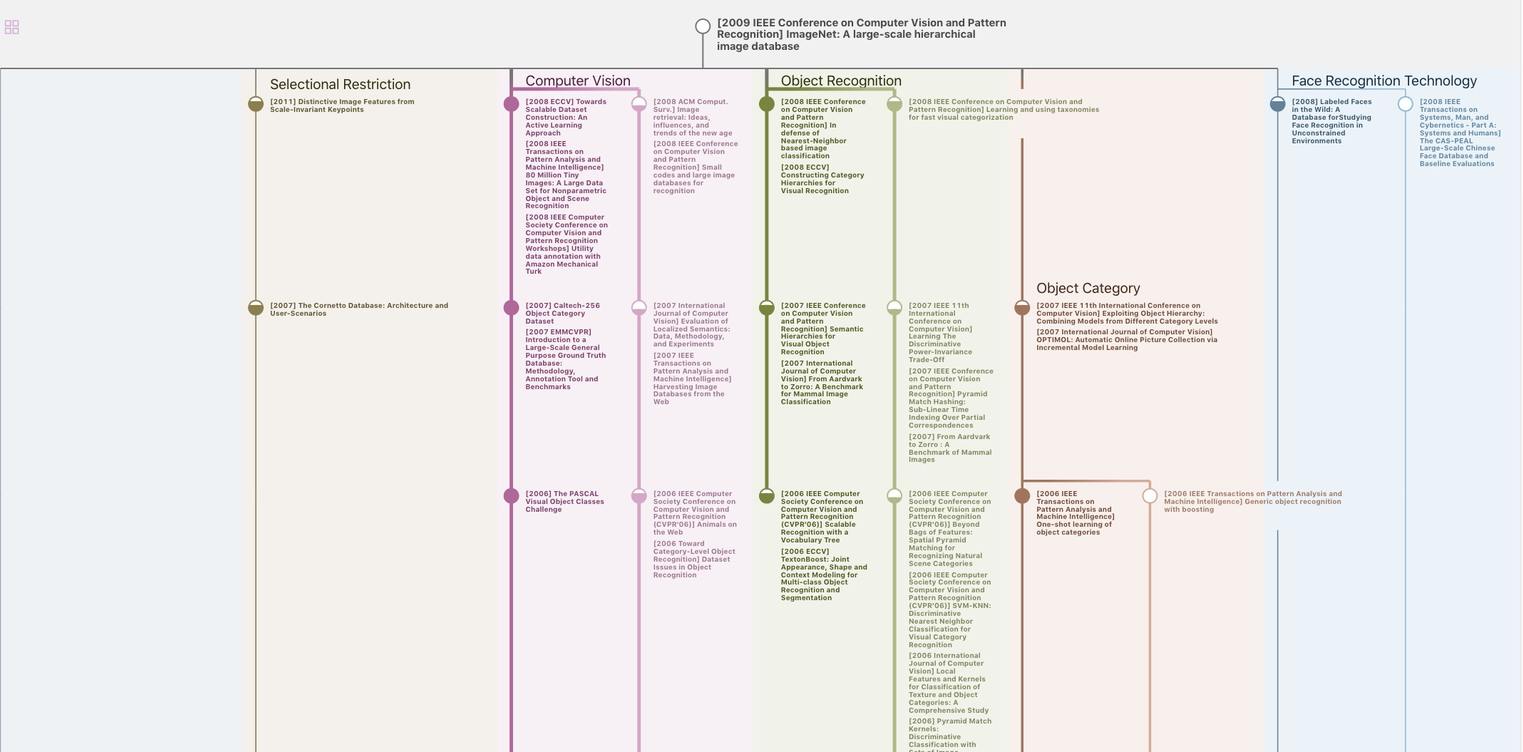
生成溯源树,研究论文发展脉络
Chat Paper
正在生成论文摘要