Alleviating Mode Collapse Problem in Deep Bayesian Active Learning.
Signal Processing and Communications Applications Conference (SIU)(2022)
摘要
Deep Bayesian Active Learning is an Active Learning technique that proves a success in high dimensional datasets. Standard Deep Models require a large amount of data to achieve high performance. On the contrary, Deep Bayesian Neural Networks facilitates high accuracy while utilizing fewer data. In addition, they outperform regular deep models during the selection process of data points since they consider the uncertainty of both model parameters and data points. Nevertheless, Deep Bayesian Active Learning is not robust against the Mode Collapse problem. In other words, it samples data points that are poor in diversity since it only considers the uncertainty of data points. This work proposes a method that combines Deep Bayesian Active Learning with a clustering based approach and alleviates the Mode Collapse problem. The proposed method outperforms the baseline model in terms of accuracy.
更多查看译文
关键词
mode collapse problem,active
AI 理解论文
溯源树
样例
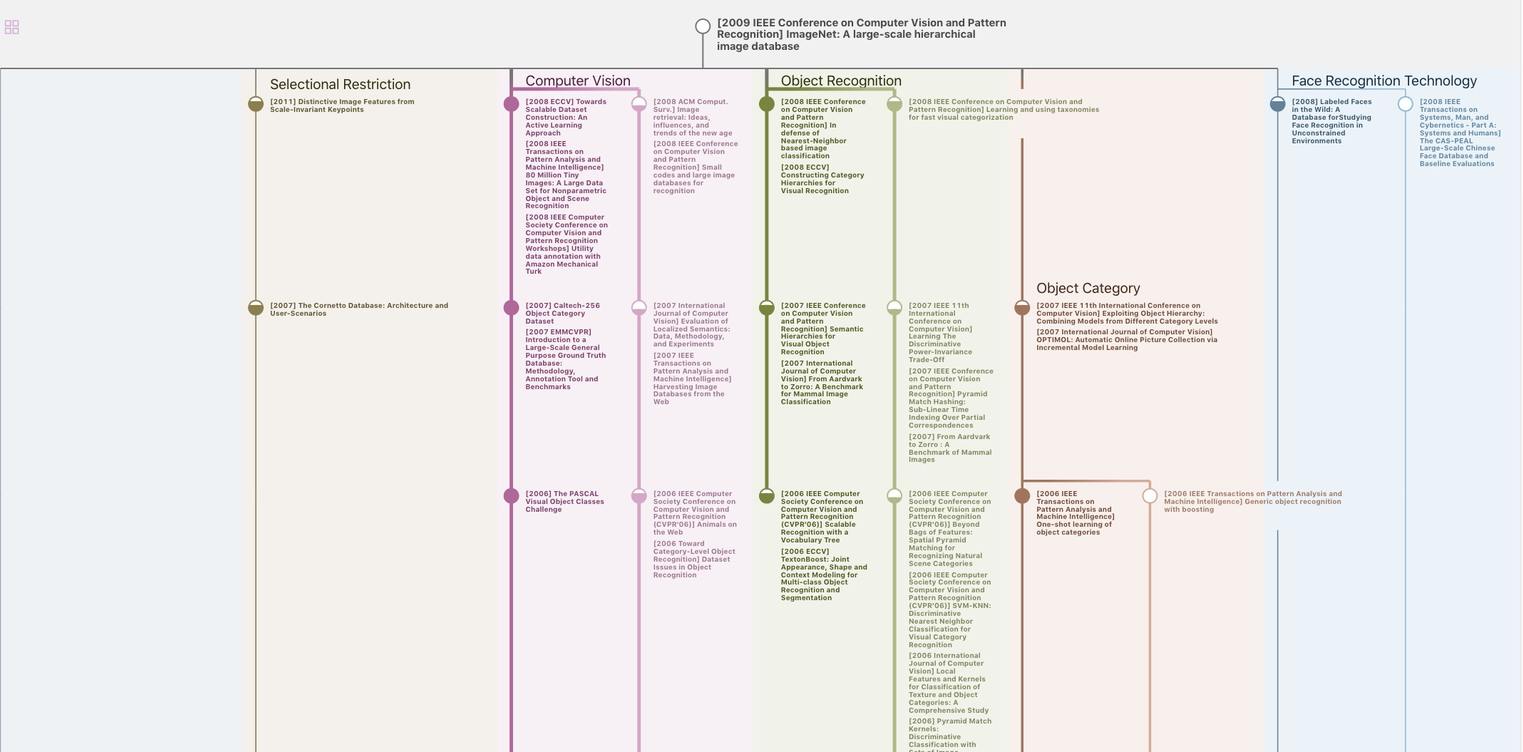
生成溯源树,研究论文发展脉络
Chat Paper
正在生成论文摘要