Hyperparameter Tunning in Simulation-based Optimization for Adaptive Digital-Twin Abstraction Control of Smart Manufacturing System.
SIGSIM Principles of Advanced Discrete Simulation (SIGSIM-PADS)(2022)
摘要
Smart manufacturing utilizes digital twins (DTs) that are virtual forms of their production plants for optimizing decisions. Discrete-event models (DEMs) are frequently used to model the production dynamics of the plants. To accelerate the performance of the discrete-event simulations (DES), adaptive abstraction-level conversion (AAC) approaches were proposed to change specific subcomponents of the DEM with corresponding abstracted queuing models during the runtime based on the steady-state of the DEMs. However, the speedup and accuracy loss of the AAC-based simulations (ABS) are highly influenced by user-specified significance level α (degree of tolerance of statistical invariance between two samples) and the stability of the DEMs. In this paper, we proposed a simulation-based optimization (SBO) that optimizes the problem based on genetic algorithm (GA) while tuning the hyperparameter (α) during runtime to maximize the speedup of ABS under a specified accuracy constraint. For each population, the proposed method distributes the computing budget between the α exploration and fitness evaluation. A discrete-gradient-based method is proposed to estimate each individual’s initial α (close to the final optimum) using previous exploration results of neighboring individuals so that the closeness can reduce the iterative α exploration as GA converges. We also proposed a clean-up method that removes inferior results to improve the α estimation. The proposed method was applied to optimize raw-material releases of a large-scale manufacturing system to prove the concept and evaluate the performance under various situations.
更多查看译文
关键词
smart manufacturing system,optimization,simulation-based,digital-twin
AI 理解论文
溯源树
样例
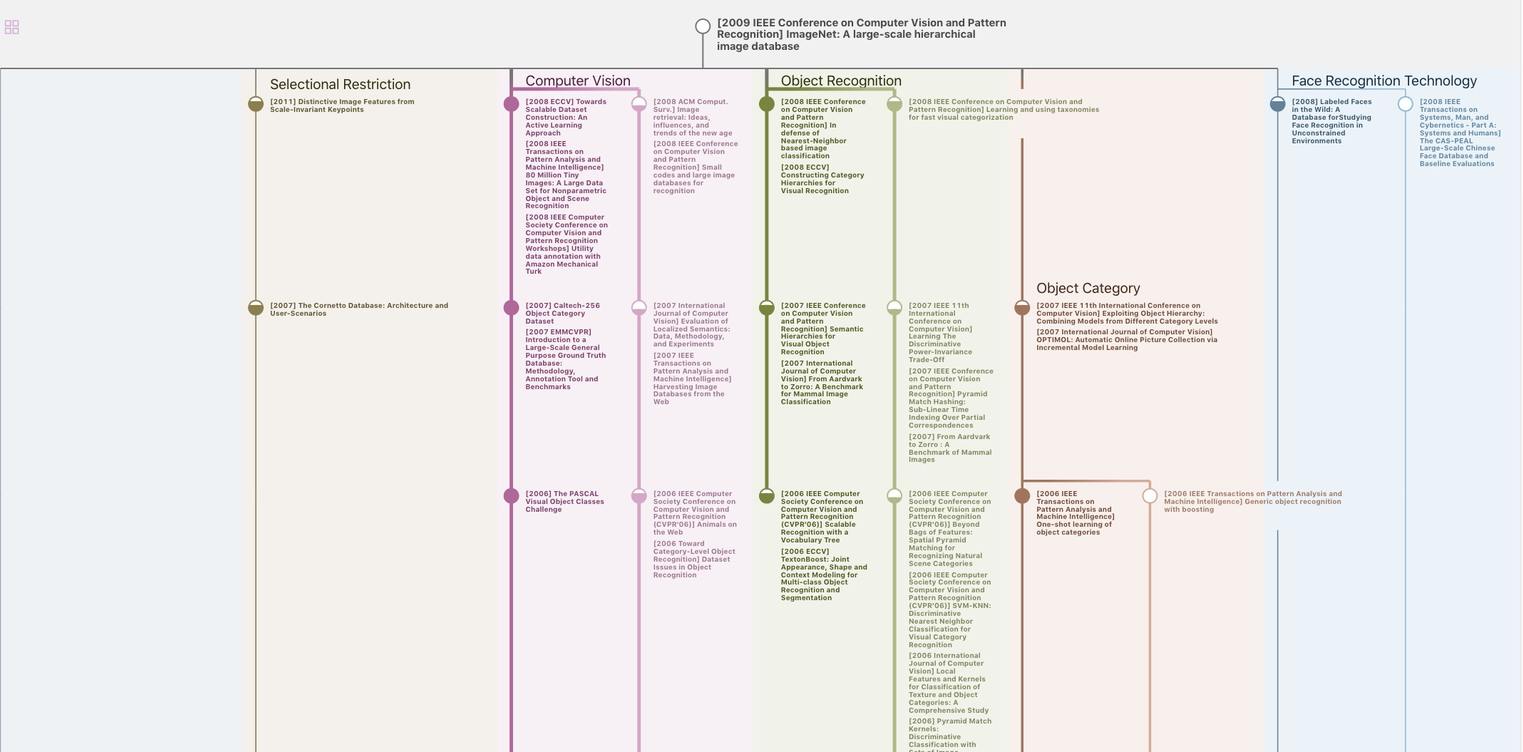
生成溯源树,研究论文发展脉络
Chat Paper
正在生成论文摘要