ACRM: Integrating Adaptive Convolution with Recalibration Mechanism for Link Prediction.
Knowledge Science, Engineering and Management (KSEM)(2022)
Abstract
Link prediction is one of the important tasks of knowledge graphs. Recently, some convolutional models inherited from computer vision have shown many advantages over other methods. The convolution-based method is capable of exploiting the full interactions between entities and relations. However, the feature maps between channels generated from the convolution step are independent, and each channel operating on the local receptacle cannot use context information outside the local receptacle. Therefore, we propose a combined ACRM model based on adaptive convolution and recalibration mechanism. This idea is inherited from ConvR model. We evaluated our proposed model on standard benchmark datasets and achieved improved results compared to baseline models for the link prediction task. Especially those datasets with no inverse relation test leakage. The experimental results show that the recalibration mechanism has improved the limitation of feature maps in convolutional neural models. In addition, we also show the convergence of the loss value during training and demonstrate the model's ability to predict the head and tail entities on common evaluation metrics.
MoreTranslated text
Key words
Knowledge graph embedding,Link prediction,Representation learning,Convolutional neural network,Recalibration mechanism
AI Read Science
Must-Reading Tree
Example
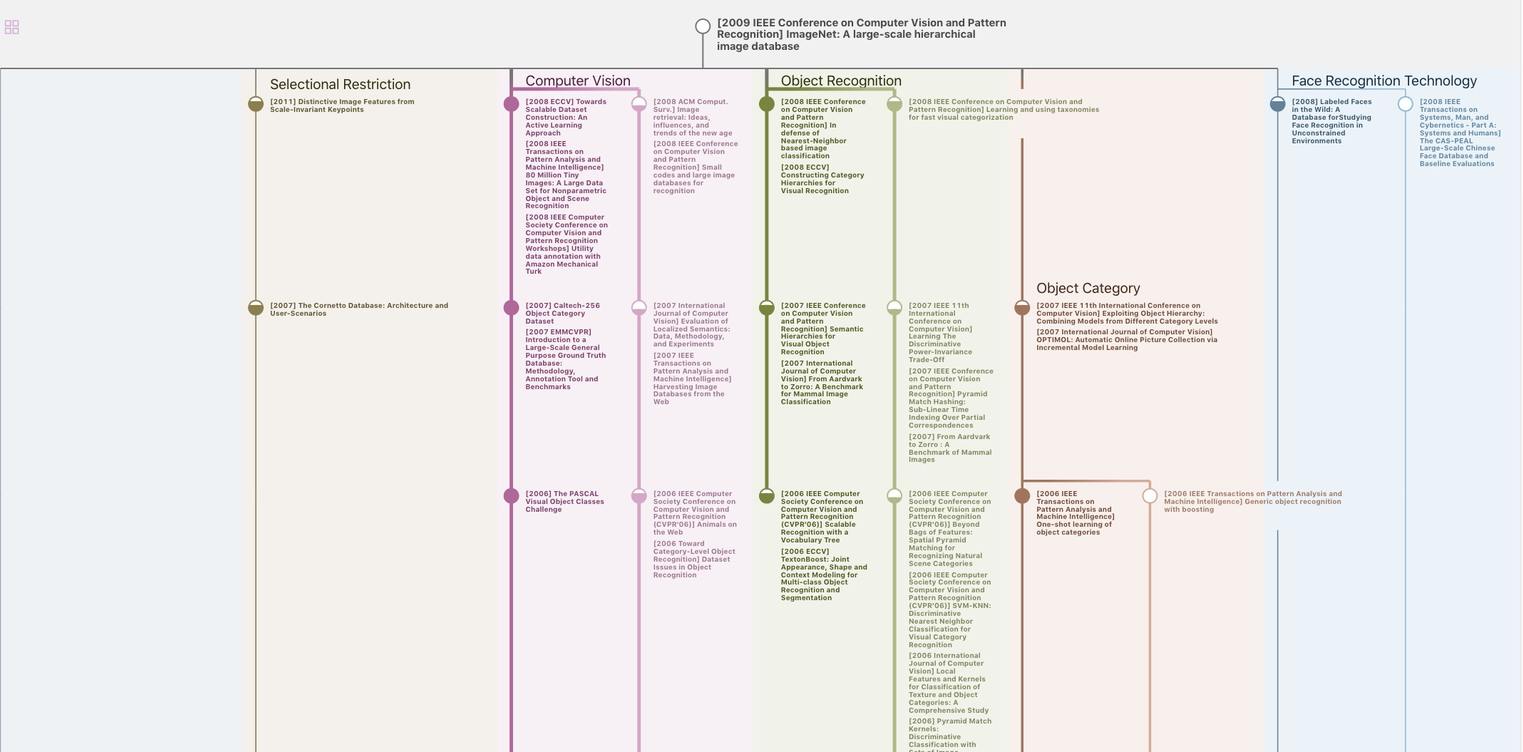
Generate MRT to find the research sequence of this paper
Chat Paper
Summary is being generated by the instructions you defined