A Spatial Interpolation Using Clustering Adaptive Inverse Distance Weighting Algorithm with Linear Regression.
Knowledge Science, Engineering and Management (KSEM)(2022)
摘要
The Inverse Distance Weighting (IDW) spatial interpolation algorithm is one of the important methods that are used to estimate the values of specific locations based on an observation dataset of samples. However, it is difficult to obtain highly accurate estimates due to some weaknesses of the IDW algorithm. To address the problems of the IDW algorithm, in this paper, we propose a new spatial interpolation method by integrating the IDW algorithm with the clustering algorithms, adaptive mechanism and weighted linear regression model, namely CAIDWR. (1) By employing the clustering algorithms, the CAIDWR method eliminates abnormal values for cleaning a sample set and clusters the similar attribute values of samples for spatial interpolation. (2) This method adaptively evaluates the distance-decay parameter by calculating the local neighborhood density of a predicted point and using a fuzzy membership function. (3) It adaptively optimizes the interpolation results by using the trend of the cleaned sample set with the weighted linear regression strategy. The CAIDWR method is applied to a system of soil testing and formulated fertilization for intelligent agriculture, we compare our CAIDWR method with the Ordinary Kriging (OK) algorithm and four IDW-like algorithms over a dataset of soil samples, and the results show that our CAIDWR method outperforms the five algorithms significantly.
更多查看译文
关键词
Inverse distance weighting,Spatial clustering,Distance-decay parameter,Weighted linear regression,K nearest neighbor
AI 理解论文
溯源树
样例
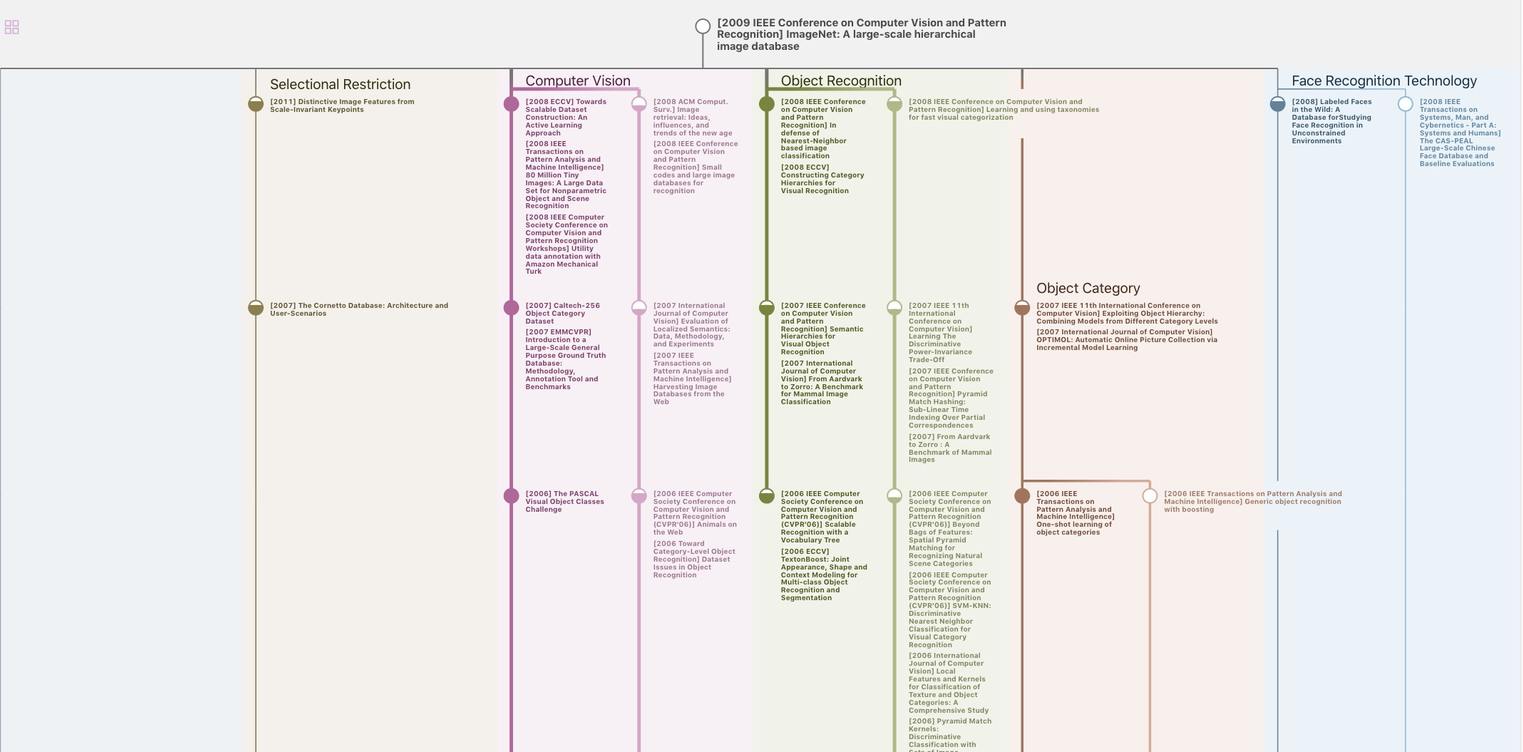
生成溯源树,研究论文发展脉络
Chat Paper
正在生成论文摘要