CLINER: Clinical Interrogation Named Entity Recognition.
Knowledge Science, Engineering and Management (KSEM)(2022)
摘要
The automatic generation of electronic medical record (EMR) data aims to create EMRs from raw medical text (e.g., doctor-patient interrogation dialog text) without human efforts. A critical problem is how to accurately locate the medical entities mentioned in the doctor-patient interrogation text, as well as identify the state of each clinical entity (e.g., whether a patient genuinely suffers from the mentioned disease). Such precisely extracted medical entities and their states can facilitate clinicians to trace the whole interrogation process for medical decision-making. In this work, we annotate and release an online clinical dialog NER dataset that contains 72 types of clinical items and 3 types of states. Existing conventional named entity recognition (NER) methods only take a candidate entity's surrounding context information into consideration. However, identifying the state of a clinical entity mentioned in a doctor-patient dialog turn requires the information across the whole dialog rather than only the current turn. To bridge the gap, we further propose CLINER, a CLinical Interrogation NER model, which exploits both fine-grained and coarse-grained information for each dialog turn to facilitate the extraction of entities and their corresponding states. Extensive experiments on the medical dialog information extraction (MIE) task and clinical interrogation named entity recognition task show that our approach shows significant performance improvement (3.72 on NER F1 and 6.12 on MIE F1) over the state-of-art on both tasks.
更多查看译文
关键词
Clinical named entity recognition,Information extraction,Coarse-grained and fine-grained context,Historical pattern memory,BERT
AI 理解论文
溯源树
样例
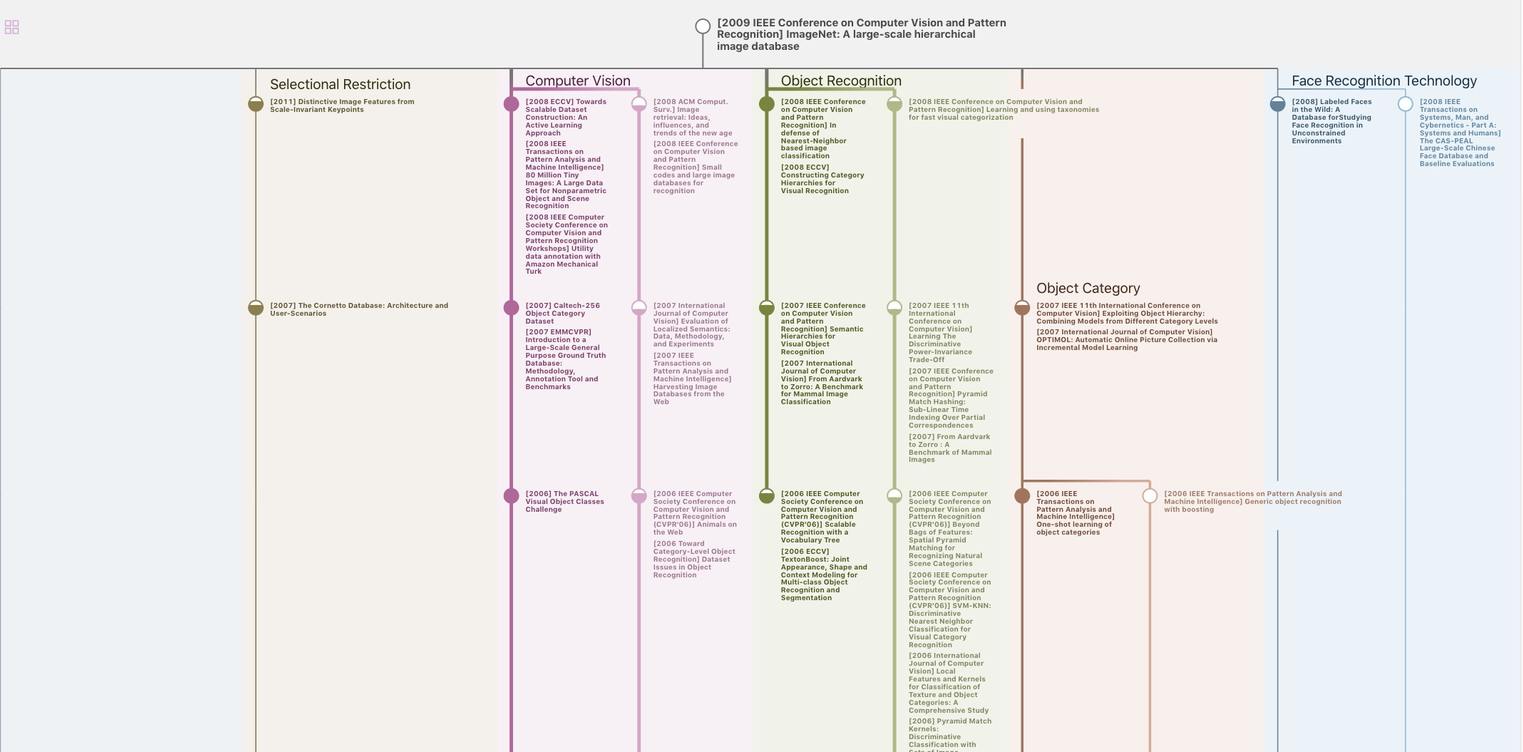
生成溯源树,研究论文发展脉络
Chat Paper
正在生成论文摘要