GM-Attack: Improving the Transferability of Adversarial Attacks.
Knowledge Science, Engineering and Management (KSEM)(2022)
摘要
In the real world, blackbox attacks seem to be widely existed due to the lack of detailed information of models to be attacked. Hence, it is desirable to obtain adversarial examples with high transferability which will facilitate practical adversarial attacks. Instead of adopting traditional input transformation approaches, we propose a mechanism to derive masked images through removing some regions from the initial input images. In this manuscript, the removed regions are spatially uniformly distributed squares. For comparison, several transferable attack methods are adopted as the baselines. Eventually, extensive empirical evaluations are conducted on the standard ImageNet dataset to validate the effectiveness of GM-Attack. As indicated, our GM-Attack can craft more transferable adversarial examples compared with other input transformation methods and attack success rate on Inc-v4 has been improved by 6.5% over state-of-the-art methods.
更多查看译文
关键词
Deep neural networks,Adversarial attack,Adversarial examples,Data augmentation,White-box/black-box attack,Transferability
AI 理解论文
溯源树
样例
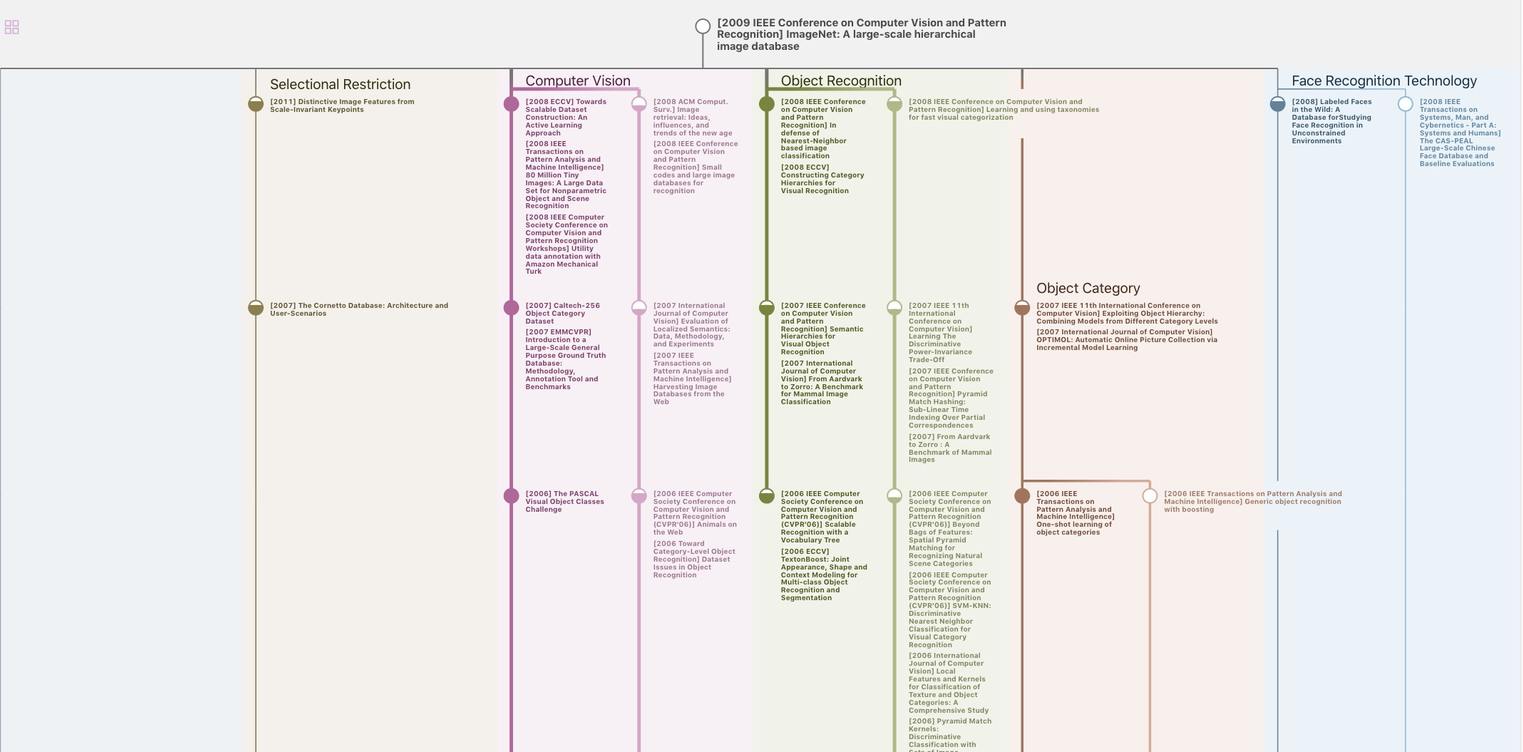
生成溯源树,研究论文发展脉络
Chat Paper
正在生成论文摘要