Multimodal Deep Learning and Fast Retrieval for Recommendation.
International Syposium on Methodologies for Intelligent Systems (ISMIS)(2022)
摘要
We propose a retrieval architecture in the context of recommender systems for e-commerce applications, based on a multi-modal representation of the items of interest (textual description and images of the products), paired with a locality-sensitive hashing (LSH) indexing scheme for the fast retrieval of the potential recommendations. In particular, we learn a latent multimodal representation of the items through the use of CLIP architecture, combining text and images in a contrastive way. The item embeddings thus generated are then searched by means of different types of LSH. We report on the experiments we performed on two real-world datasets from e-commerce sites, containing both images and textual descriptions of the products.
更多查看译文
关键词
Multimodal embeddings,Recommender systems,Locality sensitive hashing
AI 理解论文
溯源树
样例
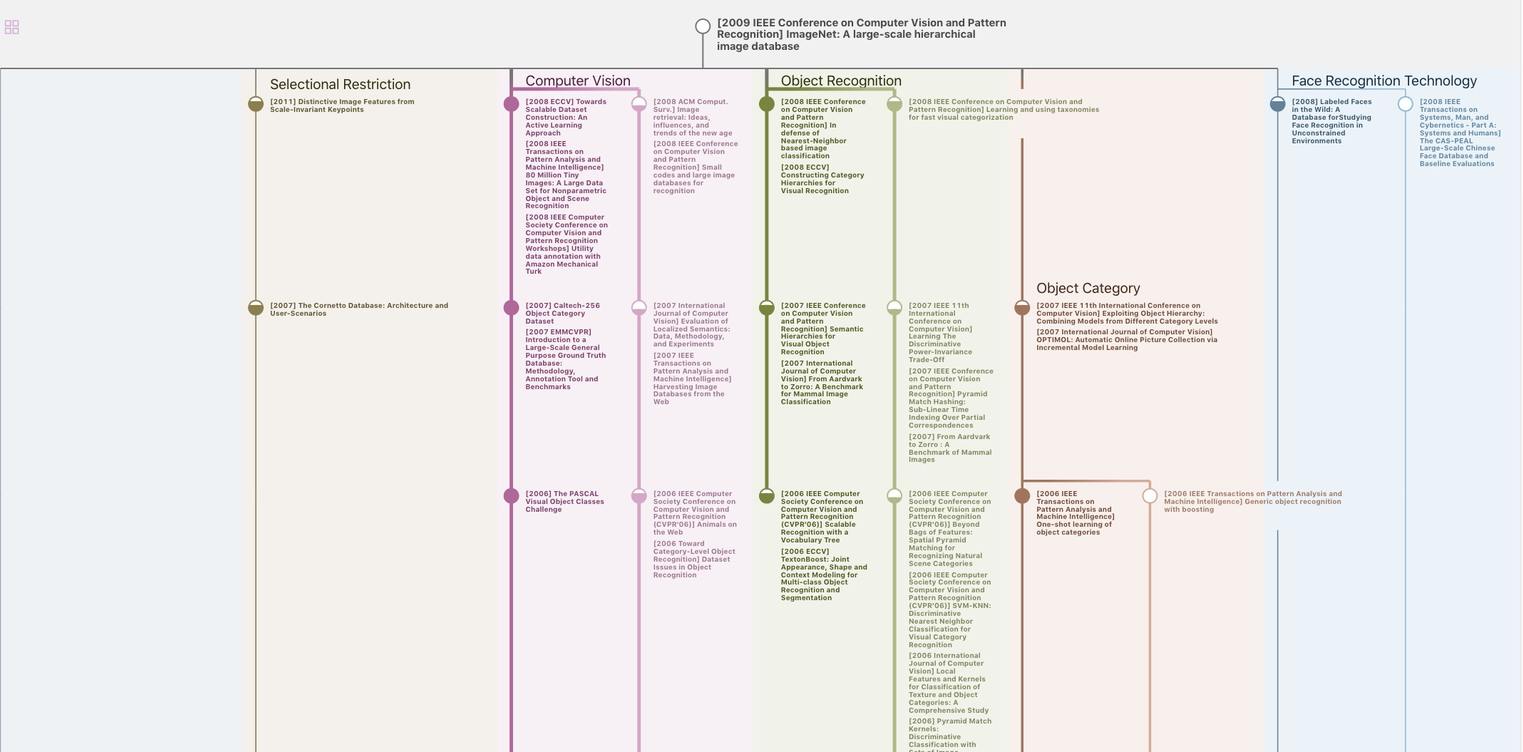
生成溯源树,研究论文发展脉络
Chat Paper
正在生成论文摘要