Composite Anomaly Detection via Hierarchical Dynamic Search.
International Symposium on Information Theory (ISIT)(2022)
摘要
Anomaly detection among a large number of processes arises in many applications ranging from dynamic spectrum access to cybersecurity. In such problems one can often obtain noisy observations aggregated from a chosen subset of processes that conforms to a tree structure. The distribution of these observations, based on which the presence of anomalies is detected, may be only partially known. This gives rise to the need for a search strategy designed to account for both the sample complexity and the detection accuracy, as well as cope with statistical models that are known only up to some missing parameters. In this work we propose a sequential search strategy using two variations of the Generalized Log Likelihood Ratio statistic. Our proposed Hierarchical Dynamic Search (HDS) strategy is shown to be order-optimal with respect to the size of the search space and asymptotically optimal with respect to the detection accuracy. An explicit upper bound on the error probability of HDS is established for the finite sample regime. Extensive experiments are conducted, demonstrating the performance gains of HDS over existing methods.
更多查看译文
关键词
sequential search strategy,finite sample regime,composite anomaly detection,dynamic spectrum access,cybersecurity,tree structure,hierarchical dynamic search strategy,generalized log likelihood ratio statistics,HDS strategy,error probability
AI 理解论文
溯源树
样例
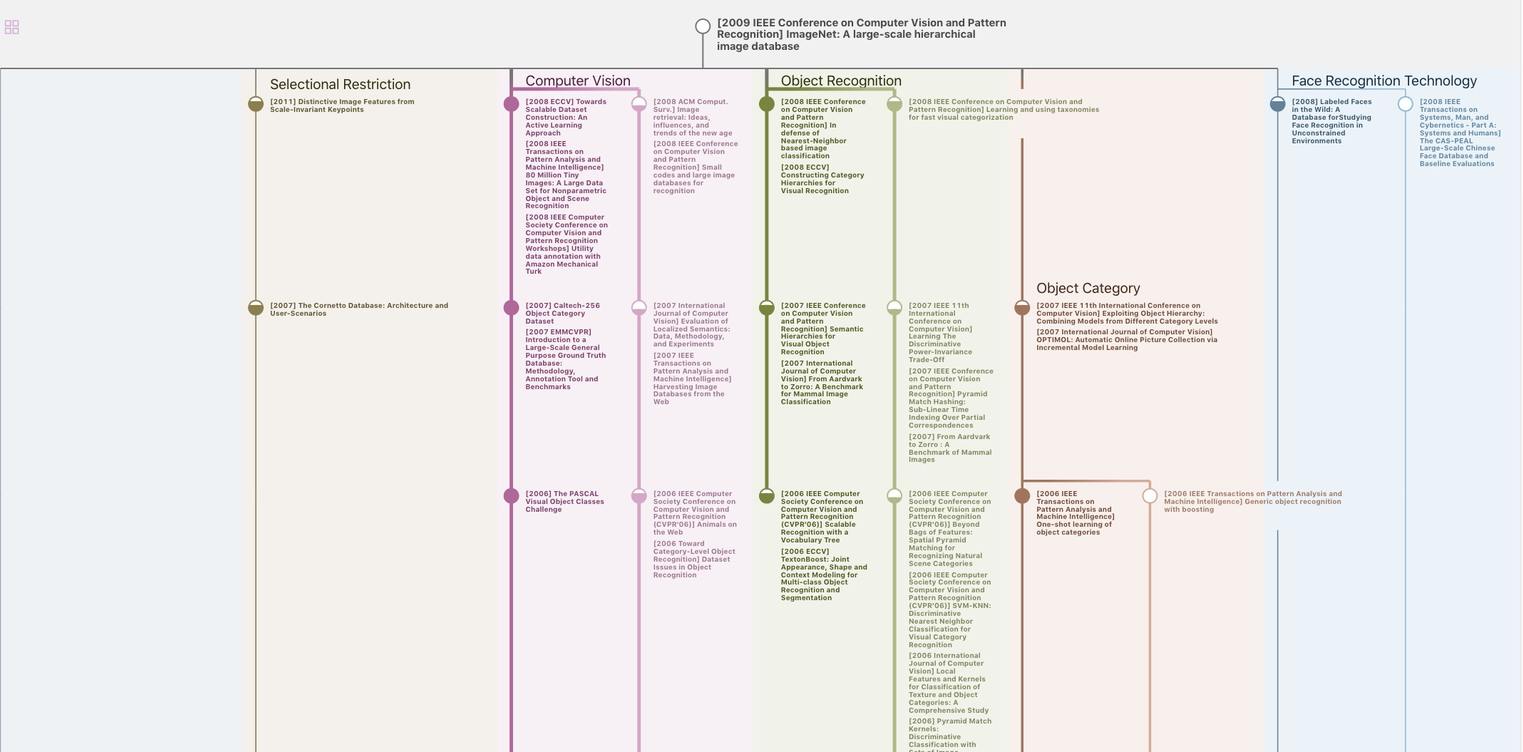
生成溯源树,研究论文发展脉络
Chat Paper
正在生成论文摘要