Achievable Error Exponents for Almost Fixed-Length Binary Classification.
International Symposium on Information Theory (ISIT)(2022)
摘要
We revisit the binary classification problem where the generating distribution under each hypothesis is unknown and propose a two-phase test, where each phase is a fixed-length test and the second-phase proceeds only if a reject option is decided in the first phase. We derive the achievable error exponents of both type-I and type-II error probabilities. Furthermore, we illustrate our results via numerical examples and show that the performance close to sequential test can be achieved with the much simpler and less complex almost fixed-length test. Our results generalize the design and analysis of the almost fixed-length test for binary hypothesis testing (Lalitha and Javidi, ISIT 2016) to the more practical setting of binary classification.
更多查看译文
关键词
Error Exponent,Classification,Two-phase test,Neyman-Pearson,Bayesian
AI 理解论文
溯源树
样例
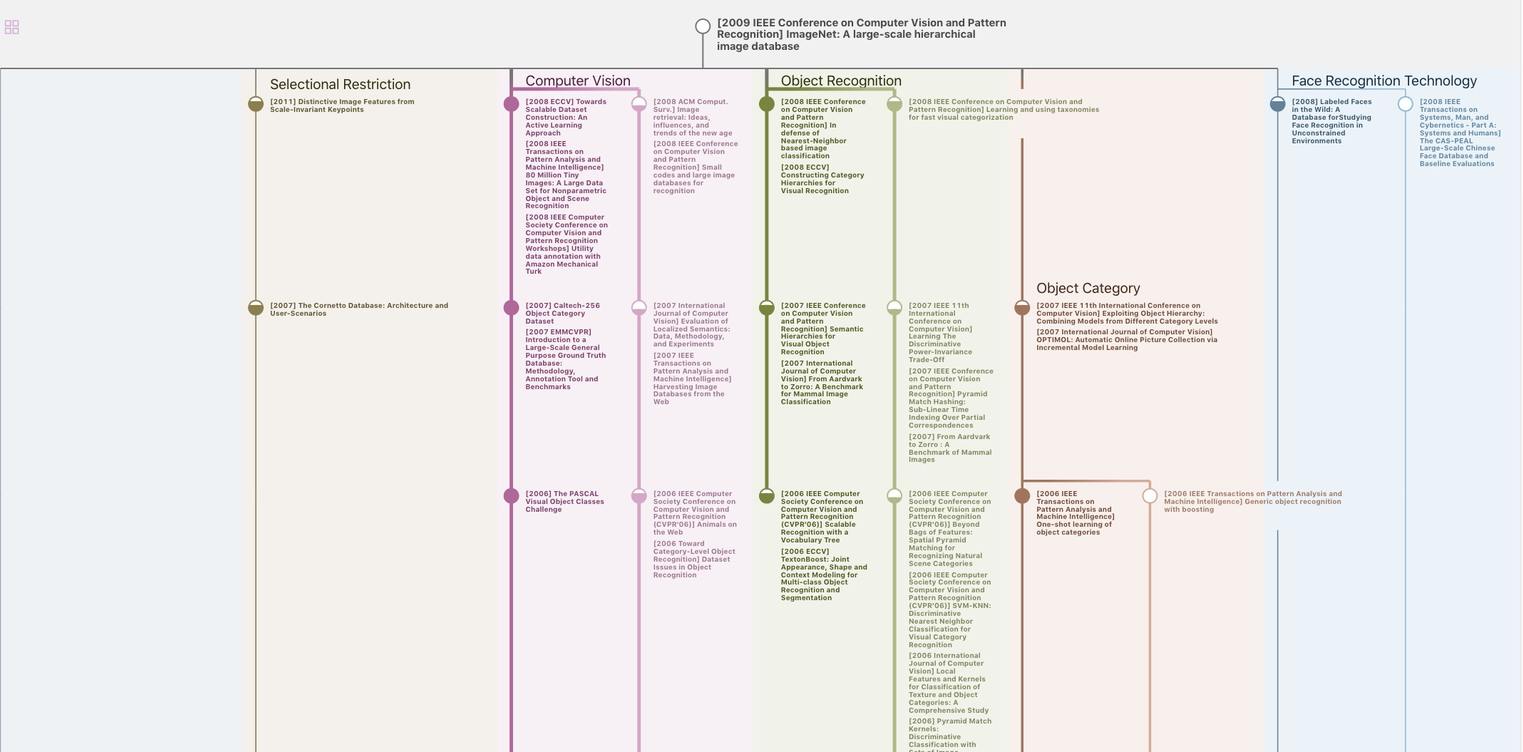
生成溯源树,研究论文发展脉络
Chat Paper
正在生成论文摘要