Secure Federated Learning for IoT using DRL-based Trust Mechanism.
International Conference on Wireless Communications and Mobile Computing (IWCMC)(2022)
摘要
Federated learning (FL) has evolved to leverage a distributed dataset from numerous IoT devices to improve the performance of a Machine Learning (ML) model while preserving the privacy of device data. Client devices train a global model jointly and share local model updates with a central entity or a server. However, FL is vulnerable to a variety of adversarial attacks that target its security and privacy and lead to compromising the main FL task. In particular, devices can contribute unreliable local model updates due to poisoning attack, or unintentionally due to their limited resources. Therefore, identifying trustworthy and reliable devices to participate in FL task is a key security challenge. In this paper, we propose a reputation management mechanism based on Deep Reinforcement Learning (DRL) in order to optimize the selection and evaluation of reliable devices and improve the accuracy of the FL model. The experimental results show that the proposed DRL-based reputation management scheme can enhance the FL accuracy by 20% while requiring fewer training iterations when compared to conventional reputation-based methods.
更多查看译文
关键词
Federated Learning, Poisoning Attack, Reputation Management, Deep Reinforcement Learning
AI 理解论文
溯源树
样例
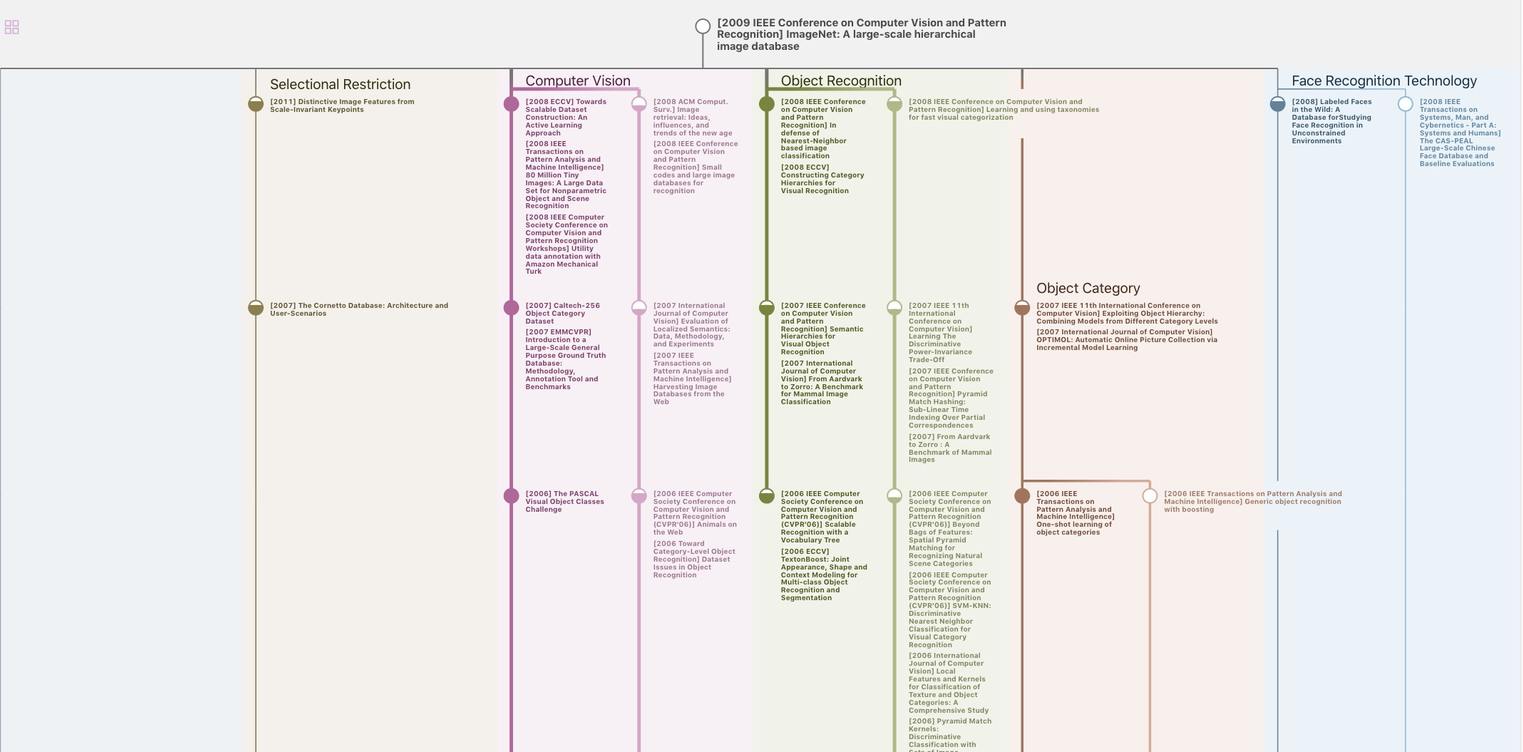
生成溯源树,研究论文发展脉络
Chat Paper
正在生成论文摘要