Prediction of Road-level Energy Consumption of Battery Electric Vehicles
International Conference on Intelligent Transportation Systems (ITSC)(2022)
摘要
Predicting the energy consumption of electric vehicles (EVs) plays an essential role in many EV planning and operation problems, such as route planning, eco-driving guidance, and charging facility design. This study analyzes the correlations of EV energy consumption with vehicle-related and environmental features from the one-year (Nov. 2017 to Nov. 2018) real-world trajectory data collected in Ann Arbor, Michigan, USA. The result suggests that the importance of these features is different for different road types. Since the road conditions and other external factors are transient and usually absent in the planning phase, we develop the problem for estimating the prediction intervals (PIs) for road-level EV energy consumption, which can consider the uncertainties. Several machine learning algorithms, such as Gradient Boosted Regression Tree (GBRT) and Extreme Gradient Boosting (XGBoost), are applied to estimate the PIs. The performances are evaluated by the percentage of observations that fall into PIs (coverage percentages) and the width of PIs (interval width). We find that GBRT provides coverage percentages over 90% and interval widths around 0.2 kWh/km for each level of roads under the confidence level of 85 %, which simultaneously satisfies a high prediction accuracy and a narrow prediction interval.
更多查看译文
关键词
Electric vehicles, energy consumption estimation, correlated features, uncertainties, prediction intervals
AI 理解论文
溯源树
样例
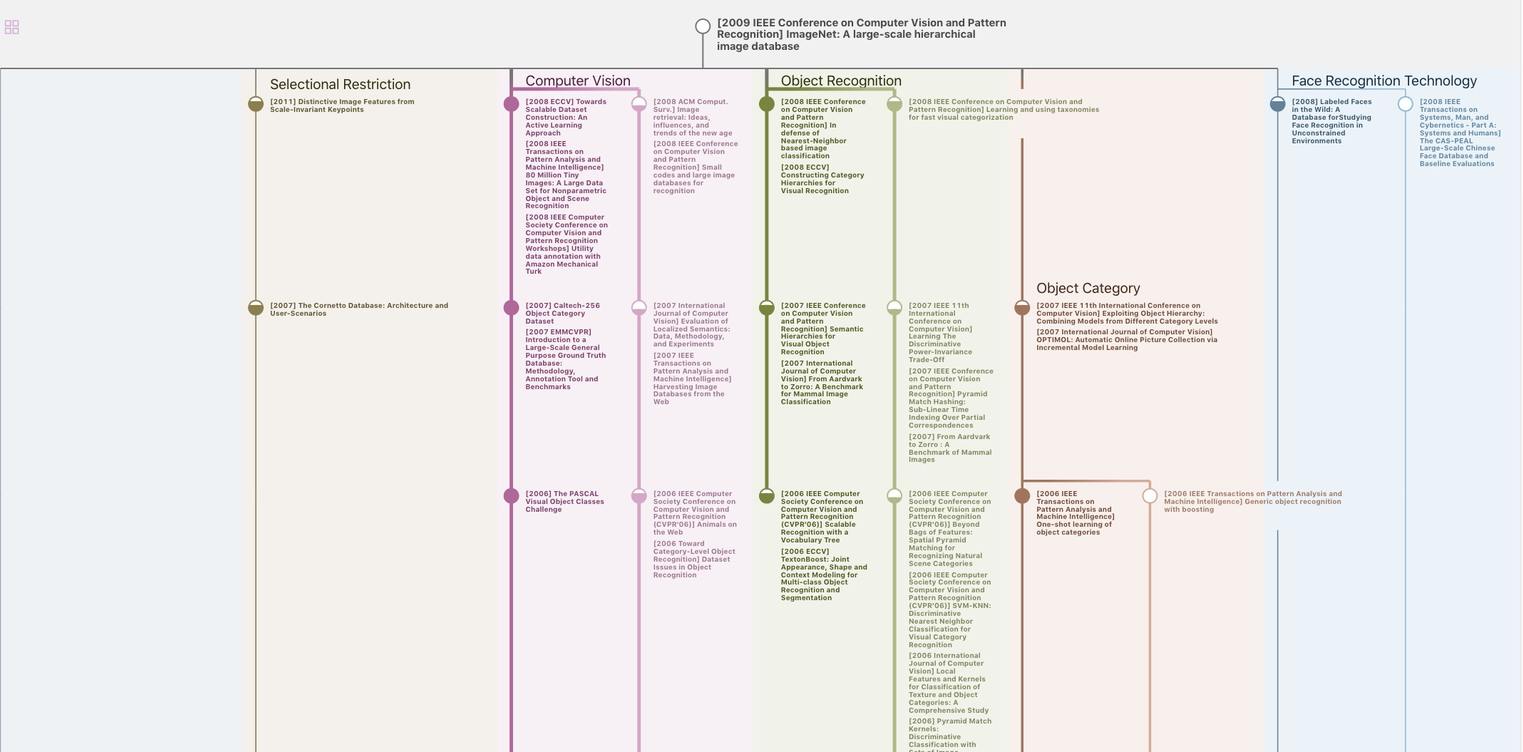
生成溯源树,研究论文发展脉络
Chat Paper
正在生成论文摘要