Assessing Buildings Damage from Multi-Temporal Sar Images Fusion using Semantic Change Detection
IEEE International Geoscience and Remote Sensing Symposium (IGARSS)(2022)
摘要
A prompt and accurate assessment of buildings' damage is critical for disaster management and emergency response. With the development of high-resolution synthetic aperture radar (SAR) and deep-learning methods, more efficient damage assessment techniques based on building-units are possible. This paper proposes a new building damage assessment method using high-resolution SAR images based on semantic change detection. It utilizes a Siamese-based module for damage change detection together with an attention mechanism-based module for semantic segmentation of the damage maps. To evaluate the proposed model, a new damage assessment dataset is constructed from the SAR imagery originated from the battle of Aleppo, Syria, for model training and testing. The experiments performed on this dataset show an overall accuracy of 88.3%. The proposed method effectively identifies the damaged areas of the buildings and grade the damage condition.
更多查看译文
关键词
buildings damage,detection,multi-temporal
AI 理解论文
溯源树
样例
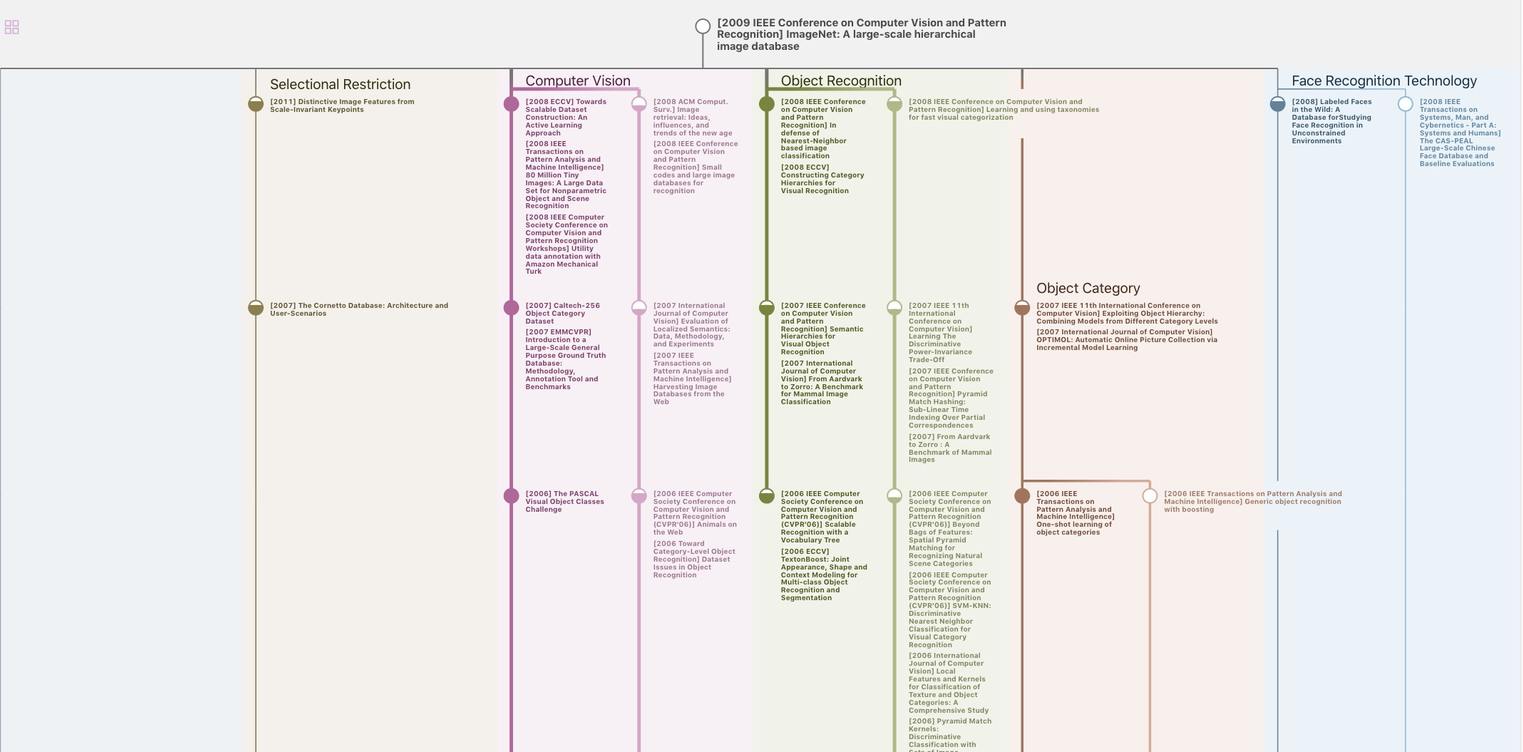
生成溯源树,研究论文发展脉络
Chat Paper
正在生成论文摘要