Built-Up Area Extraction From GF-3 Image based on an Improved Transformer Model.
IEEE International Geoscience and Remote Sensing Symposium (IGARSS)(2022)
Abstract
With the development of urbanisation in China, the urban areas are expanding rapidly, but there is a huge regional disparity between the east, central and western regions. The urban development in the western region lags far behind that in the eastern and central regions. In the western region of China, due to the large number of mountains and SAR backscatter mechanism, there are a lot of overlays in the image, resulting in high false alarms in built-up areas segmentation. In order to solve the problem, this paper proposed a new built-up area extraction model based on the Transformer. Different from the segmentation method based on convolutional neural network, the self-attention mechanism of the Transformer was introduced to effectively capture the image context information and reduce the impact of mountain overlays on the extraction of built-up areas. The multi-layer Transformer encoder and the multilayer perceptron (MLP) decoder were used to fuse feature maps of different scales for the sake of enhancing the ability to extract architectural features. With the purpose of improving the generalization ability, various data augmentation methods were used during training, such as random noise, random blur, and random distortion. In this paper, about 32000 samples, including some mountainous areas and around China, were used for training, which are from 27 scenes of GF-3 10m SAR images covering different areas in China. The method proposed in this paper reached a mIoU of 0.8130 and a Kappa coefficient of 0.9423, which significantly reduced false alarms in mountainous areas. Taking the study area of Lanzhou City, Gansu Province of China as an example, the result is basically consistent with the classification map of World Cover. It shows that the proposed method has a good ability to extract the distribution information of built-up areas.
MoreTranslated text
Key words
Built-up area,SAR,GF-3,Transformer,Deep learning
AI Read Science
Must-Reading Tree
Example
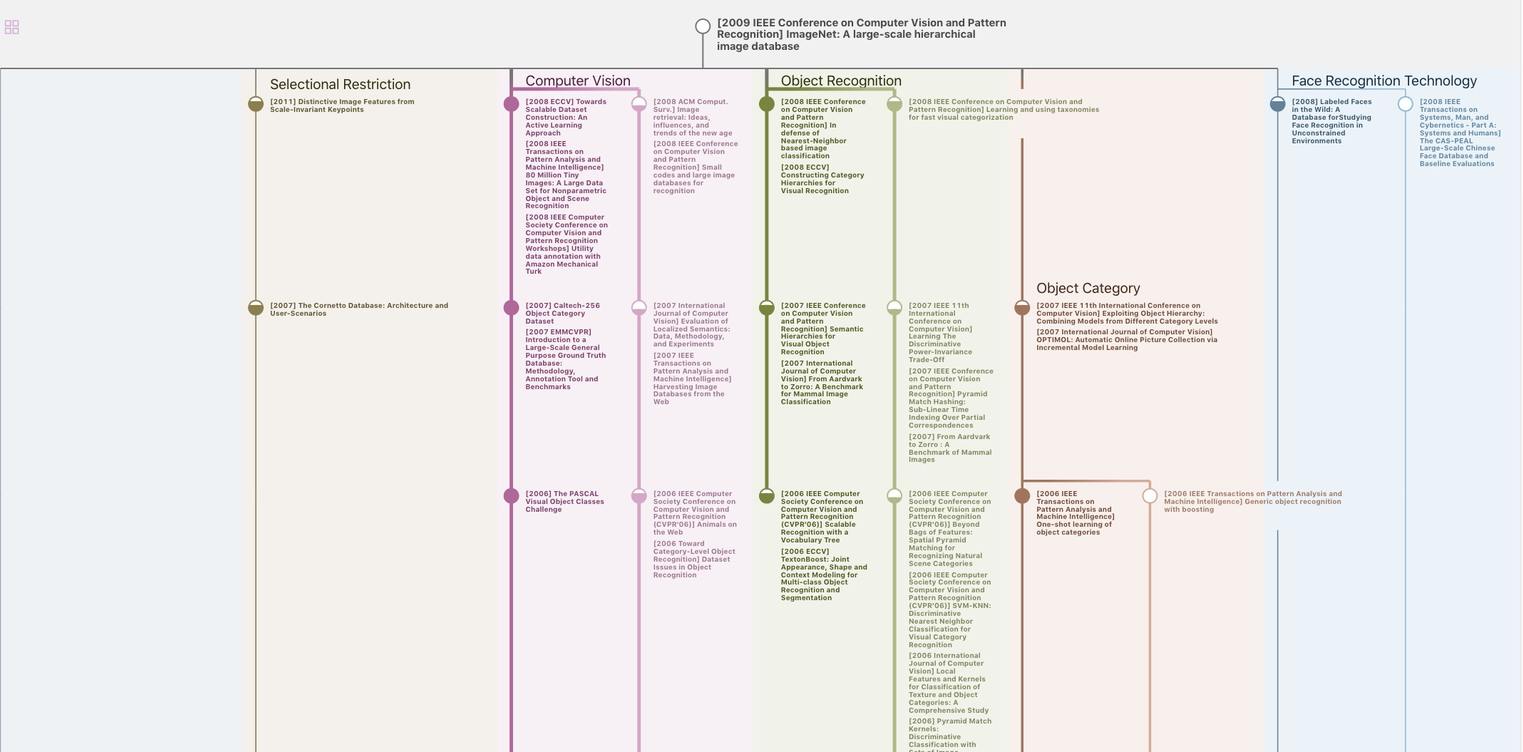
Generate MRT to find the research sequence of this paper
Chat Paper
Summary is being generated by the instructions you defined