Quantum-Compatible Variational Segmentation for Image-to-Image Wildfire Detection Using Satellite Data.
IEEE International Geoscience and Remote Sensing Symposium (IGARSS)(2022)
摘要
Wildfire occurrences have been increasing for the past decade, leaving devastating traces across the world. In the recent efforts, remote sensing and airborne missions have been utilized to better understand and manage wildfires. This has resulted in an exponential increase in volume of remote sensing data, which has pushed the need for intelligent automation of data extraction for wildfire studies. Machine learning offers accurate automation in detecting such natural anomalies and enable decision-makers to take actions in a timely manner. Recent advances in machine learning algorithms, namely probabilistic generative methods, allow researchers and decision-makers to step beyond detection and study "what-if" scenarios for wildfire occurrences. Additionally, they offer better imitations to the stochastic behavior of nature, and wildfire events. However, optimizing the performance of these probabilistic generative models is a computationally expensive process, specially using digital computers. On the other hand, quantum computers have recently shown a promise to reduce computationally costly training of such models and provide performance improvements. There is a body of research investigating the potential for improved machine learning methods in which key operations are performed on a quantum computer. In this study, we propose a probabilistic image-to-image segmentation approach combining a very well-known segmentation method, U-NET, with a Conditional Variational Auto-Encoder (CVAE) to not only detect wildfires but also describe the stochasticity of the phenomenon and be capable of running "what-if" scenarios. Our proposed model is compatible with training on quantum computers, which results in a quantum-assisted image-to-image segmentation approach and can be used to benchmark the potential benefit of quantum computing over the classical one.
更多查看译文
关键词
detection,quantum-compatible,image-to-image
AI 理解论文
溯源树
样例
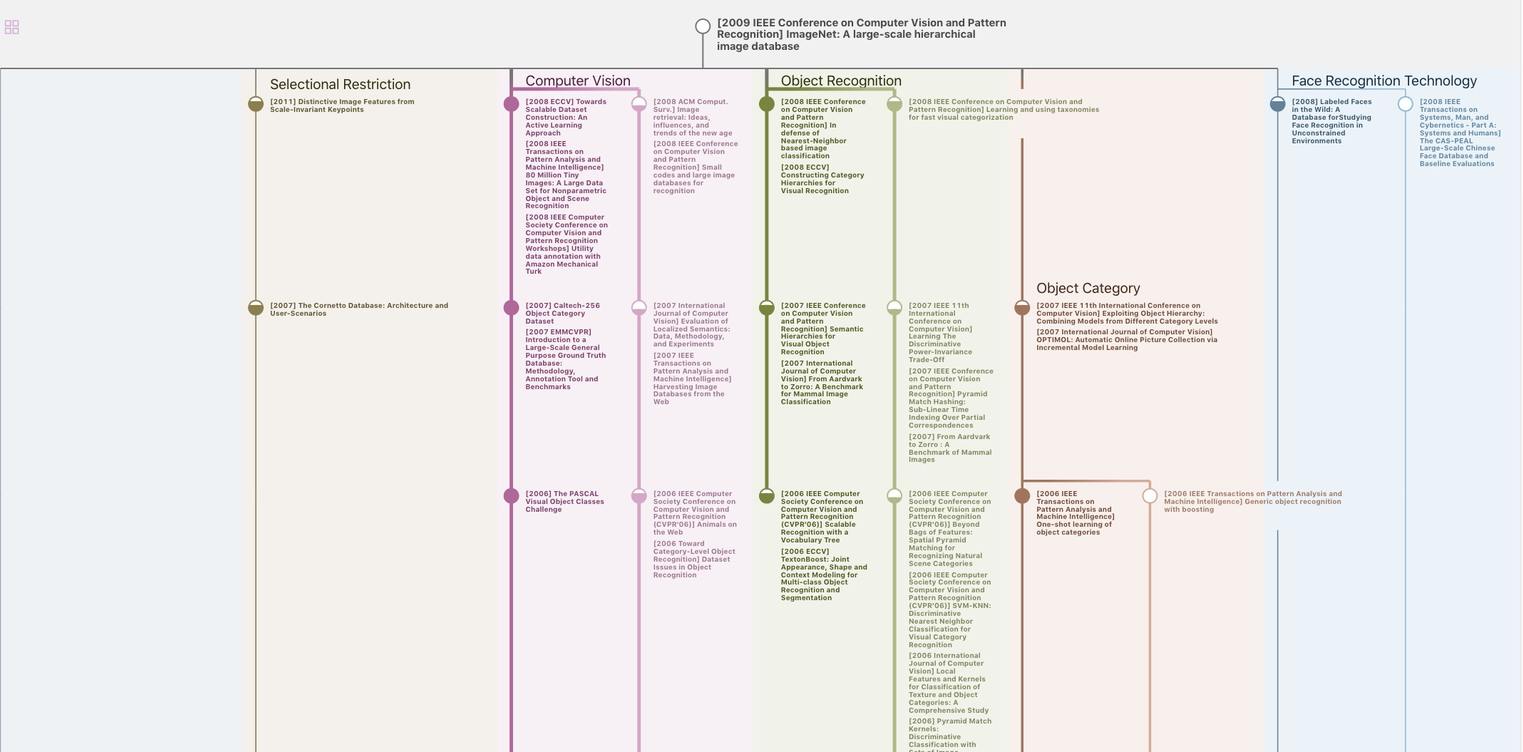
生成溯源树,研究论文发展脉络
Chat Paper
正在生成论文摘要