Explainability Analysis of CNN in Detection of Volcanic Deformation Signal.
IEEE International Geoscience and Remote Sensing Symposium (IGARSS)(2022)
摘要
With improvement in the processing of synthetic aperture radar interferometry (InSAR) data, the detection of long-term volcanic deformations becomes possible. While deep learning (DL) models are considered black-box models, challenging to debug, the advances in explainable AI (XAI) help understand the model and how it makes decisions. In this paper, the model is trained on synthetic InSAR velocity maps to detect slow, sustained deformations. XAI tools, including Grad-CAM and t-SNE, are utilized for understanding and improving the trained model. Grad-CAM helps identify the slope-induced signal and salt lake patterns responsible for the model's misclassifications. T-SNE feature representation visualizations are used to estimate data sets and model class separation ability. Additionally, a sensitivity analysis shows the model performance with different intensity deformation data and uncovers the minimal detectable deformations of 1 cm cumulative deformation over five years.
更多查看译文
关键词
cnn,detection,deformation
AI 理解论文
溯源树
样例
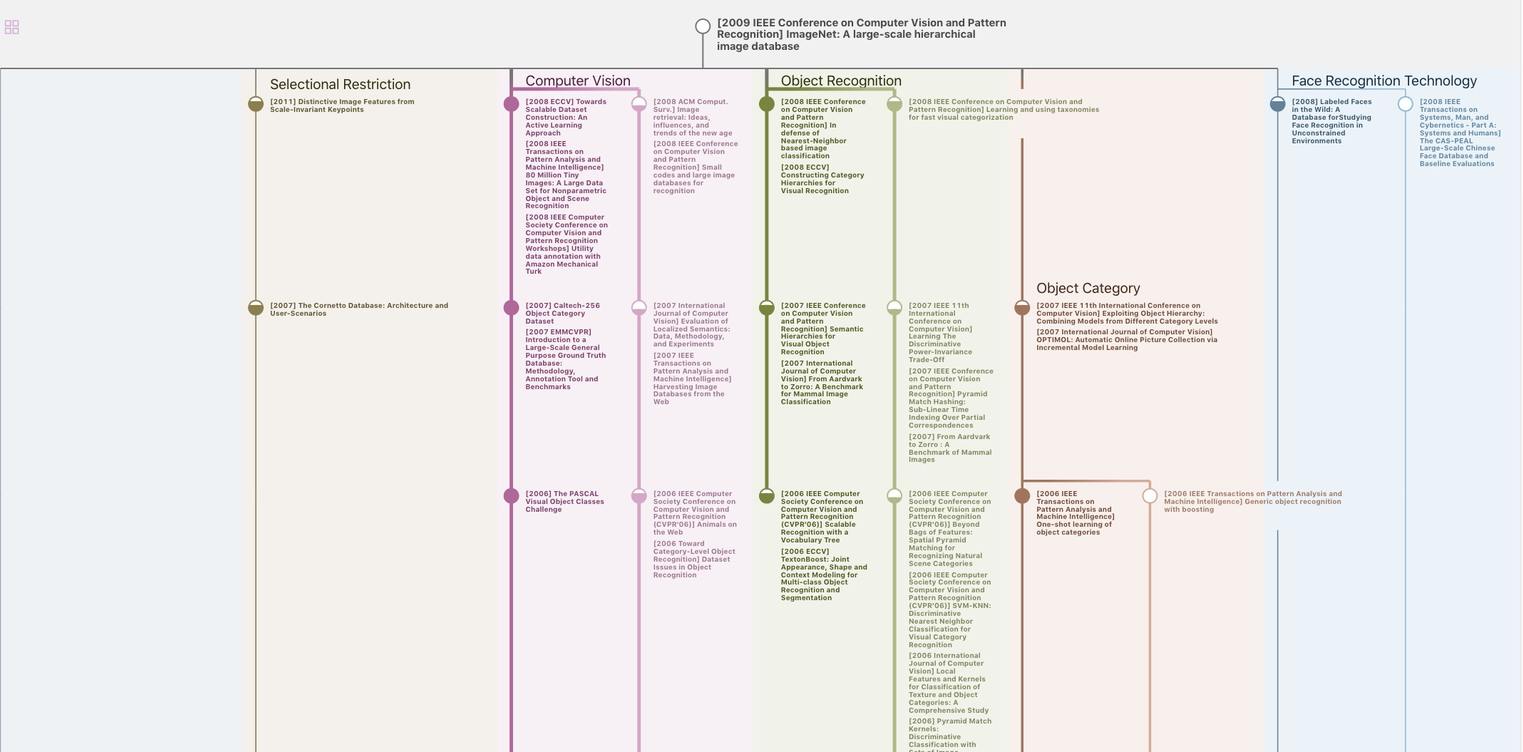
生成溯源树,研究论文发展脉络
Chat Paper
正在生成论文摘要