Gan-based SAR to Optical Image Translation in Fire-Disturbed Regions.
IEEE International Geoscience and Remote Sensing Symposium (IGARSS)(2022)
摘要
Climate change by anthropogenic warming leads to increases in dry fuels and promotes forest fires. Multispectral images' quality is easily affected by poor atmospheric conditions. SAR satellite sensors can penetrate through clouds and image day and night. However, the burned area mapping methods widely used for optical data are not feasible to be applied for SAR data owing to the differences in imaging mechanisms. Recent advances in deep image translation can fill this gap by using Generative Adversarial Networks (GAN). In this research, we apply a GAN-based model for SAR to optical image translation over fire-disturbed regions. Specifically, Sentinel-1 SAR images are translated into Sentinel-2 images using the ResNet-based Pix2Pix model, which is trained on 281 large fire events and tested on the other 23 events in Canada. The generated images preserve the spectral characteristics well and show high similarity to the real images with Structure Similarity Index Measure (SSIM) over 0.59.
更多查看译文
关键词
optical image translation,sar,gan-based,fire-disturbed
AI 理解论文
溯源树
样例
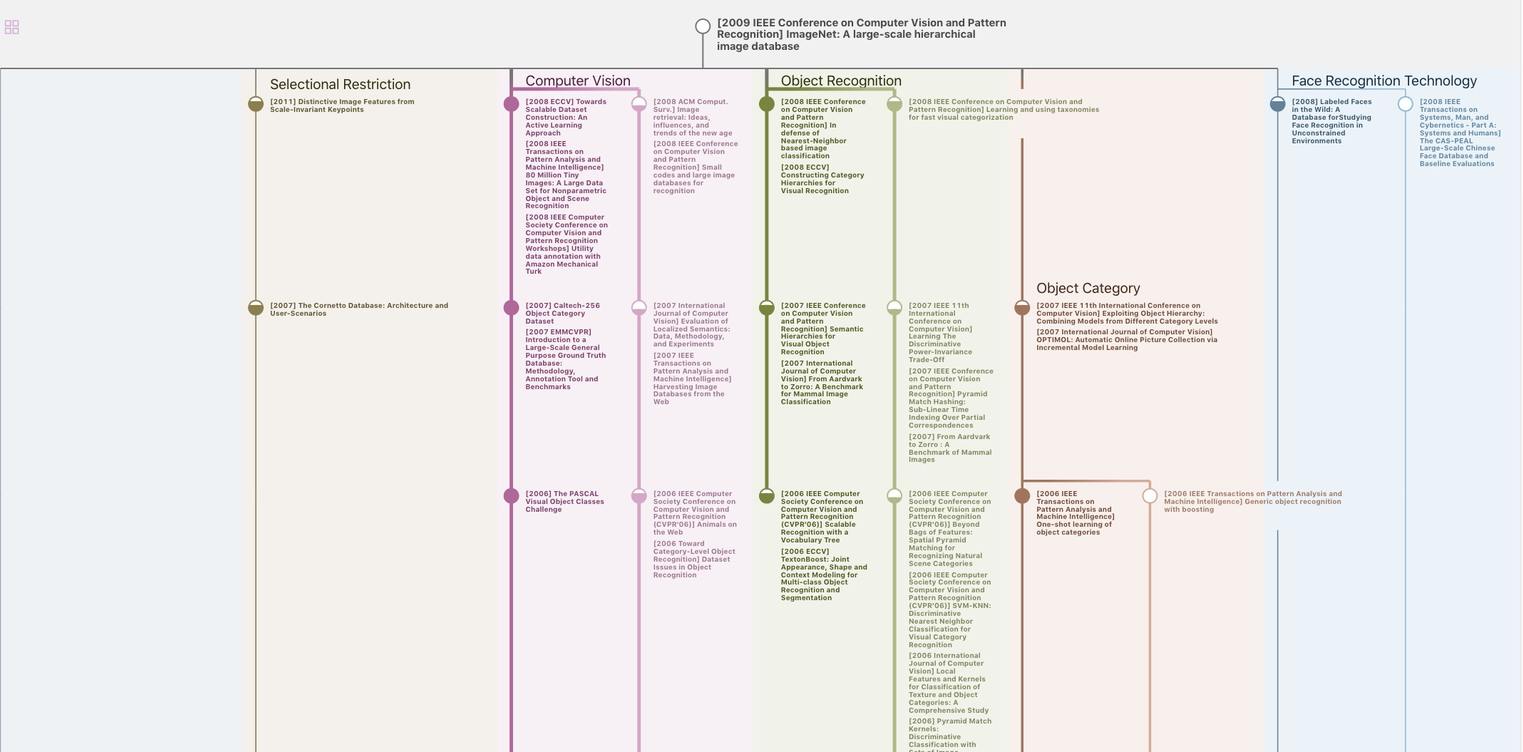
生成溯源树,研究论文发展脉络
Chat Paper
正在生成论文摘要