Hyperspectral Band Selection Via Sparse Principal Component Analysis and Adaptive Multiple Graph Learning.
IEEE International Geoscience and Remote Sensing Symposium (IGARSS)(2022)
摘要
For hyperspectral image, it is a challenging task to select informative and distinctive bands due to the lack of labeled samples and massive redundancy. To address this issue, we propose a new unsupervised band selection method via Sparse Principal Component Analysis and Adaptive Multiple Graph Learning (SPCA-AMGL). Based on PCA, it proposes a Sparse PCA with L-2,L-1 norm sparse constraint, which can effectively select the bands with high information and low correlation. In addition, an adaptive multiple graph learning is used for manifold-preserving, which ensures that the bands containing abundant spatial structure information are preserved. Specifically, it constructs multiple initial similarity graphs with different distance metrics, and then learns an adaptive graph from them. In this way, it overcomes the shortcoming of insufficient intrinsic structure of data learned from a single graph. Experimental result on Indian Pines data set proves the effectiveness and advancement of SPCA-AMGL. The source code is available at: https://github.com/ZWX0823/SPCA-AMGL.
更多查看译文
关键词
selection,graph
AI 理解论文
溯源树
样例
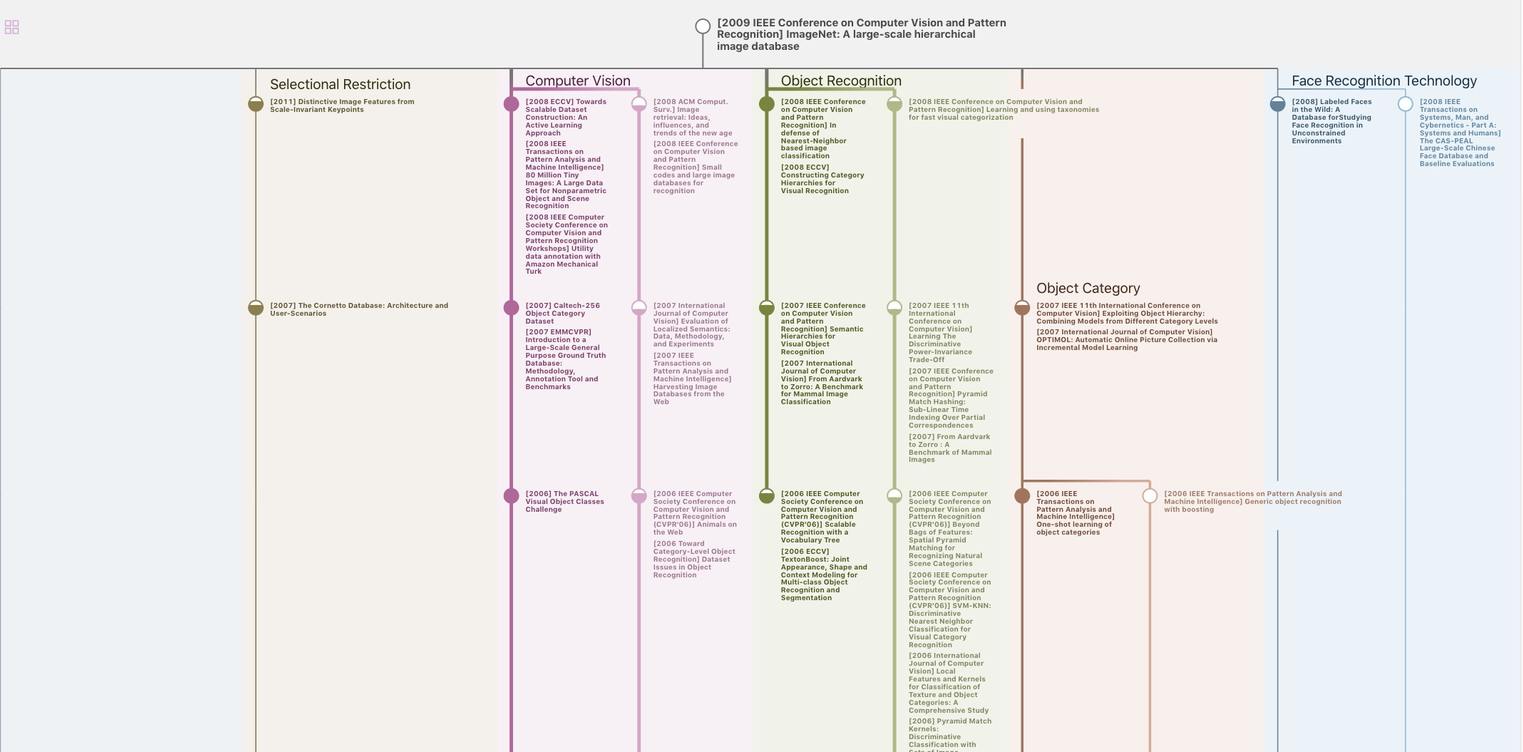
生成溯源树,研究论文发展脉络
Chat Paper
正在生成论文摘要