Complex-Valued Sparse Long Short-Term Memory Unit with Application to Super-Resolving SAR Tomography.
IEEE International Geoscience and Remote Sensing Symposium (IGARSS)(2022)
摘要
To achieve super-resolution synthetic aperture radar (SAR) tomography (TomoSAR), compressive sensing (CS)-based algorithms are usually employed, which are, however, computationally expensive, and thus is not often applied in largescale processing. Recently, deep unfolding techniques have provided a good combination of physical model-based algorithms and the ability of neural networks to learn from data. In this vein, iterative CS-based algorithms can usually be unrolled as neural networks with only 10 to 20 layers. When trained, it shows great computational efficiency for further TomoSAR processing. However, the learning architecture of neural networks built in this approach tends to result in error propagation and information loss, thus degrading the performance. In this paper, we propose to employ complex-valued sparse long short-term memory (CV-SLSTM) units to tackle this problem by incorporating historically updating information into the optimization procedure and preserving full information. Simulations are carried out to validate the performance of the proposed algorithm.
更多查看译文
关键词
SAR tomography, Super-resolution, Complex-valued neural network, deep learning
AI 理解论文
溯源树
样例
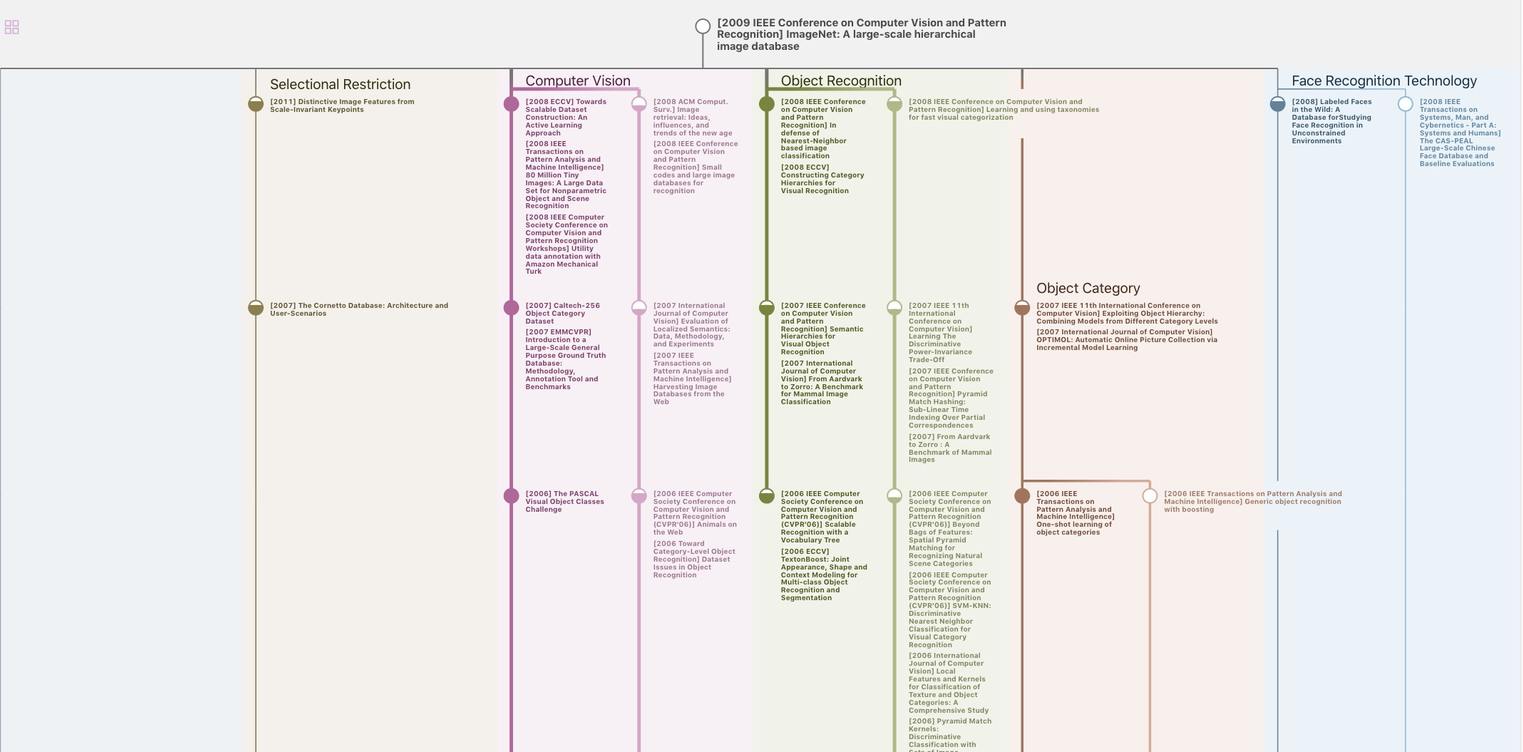
生成溯源树,研究论文发展脉络
Chat Paper
正在生成论文摘要