PG-BCNet : A Neural Network Combined with the PGNet and BCNet for 2-D InSAR Phase Unwrapping
IEEE International Geoscience and Remote Sensing Symposium (IGARSS)(2022)
摘要
A deep convolutional neural network (DCNN) has been widely applied to the 2-D phase unwrapping (PU) in synthetic aperture radar interferometry (InSAR). Our previously-developed PGNet and BCNet outperform the model-based 2-D PU methods. However, the two networks can be further improved. As the PGNet is limited to estimating the phase gradients within ±2π, unwrapped phases can be incorrectly unwrapped sometimes. The BCNet is sensitive to the high-density distribution of the residues caused by a noisy interferogram, resulting in many isolated regions. To solve both issues, we bridge the PGNet and BCNet, studying a new DCNN-based 2-D PU framework (PG-BCNet). The results show that the PG-BCNet is more noise-robust than that of the BCNet and overcomes the limitation of the PGNet that cannot unwrap the phase gradients beyond ±2π.
更多查看译文
关键词
BCNet, Deep convolutional neural network, PGNet, Phase unwrapping, Synthetic aperture radar interferometry
AI 理解论文
溯源树
样例
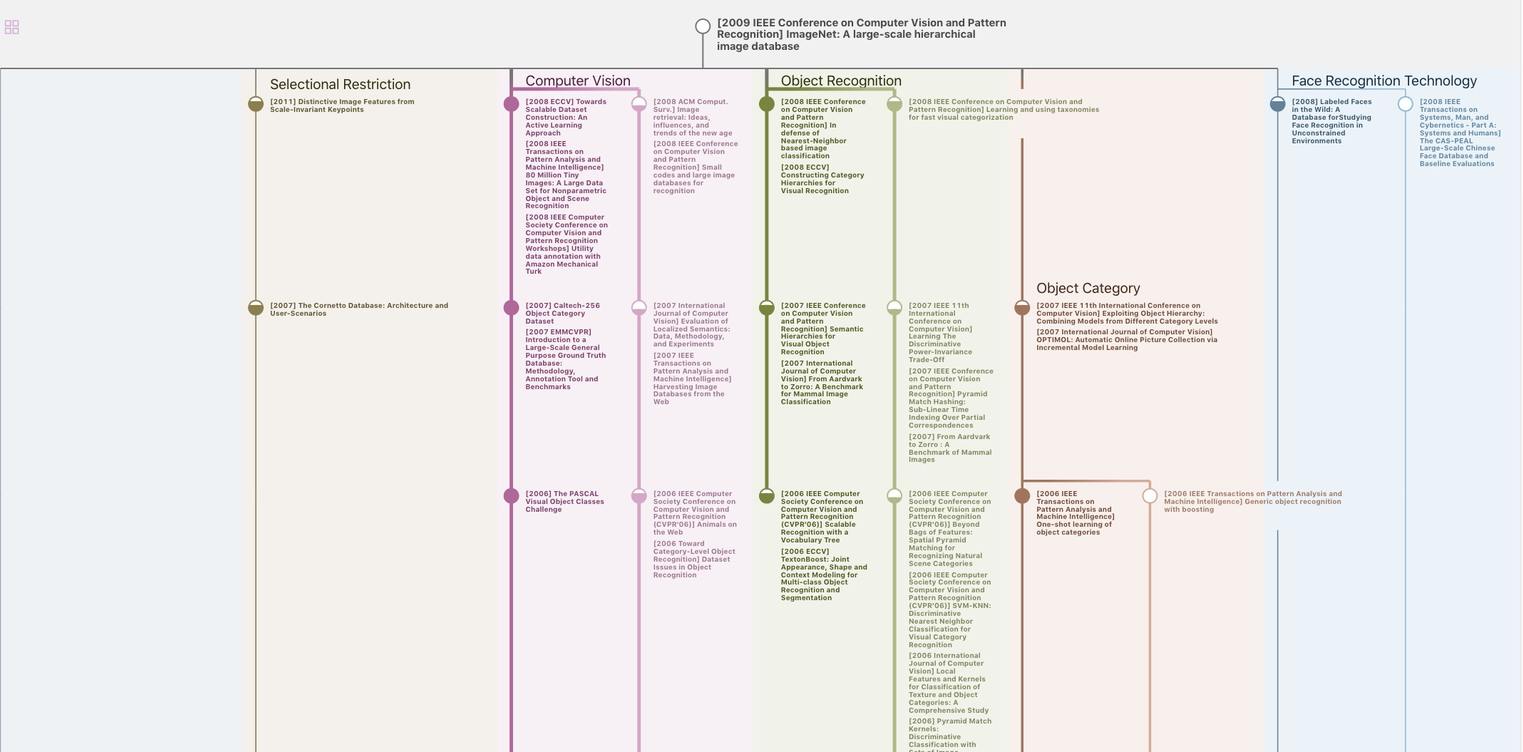
生成溯源树,研究论文发展脉络
Chat Paper
正在生成论文摘要