Lite CNN Models for Real-Time Post-Harvest Grape Disease Detection.
International Conference on Intelligent Environments (IE)(2022)
摘要
Post-harvest fruit grading is a necessary step to avoid disease related loss in quality. In this paper, a hierarchical method is proposed to (1) remove the background and (2) detect images that contains grape diseases(botrytis, oidium, acid rot). Satisfying segmentation performances were obtained by the proposed Lite Unet model with 92.9% IoU score and an average speed of 0.16s/image. A pretrained MobileNet-V2 model obtained 94% F1 score on disease classification. An optimized CNN reached a score of 89% with less than 10 times less parameters. The implementation of both segmentation and classification models on low-powered device would allow for real-time disease detection at the press.
更多查看译文
AI 理解论文
溯源树
样例
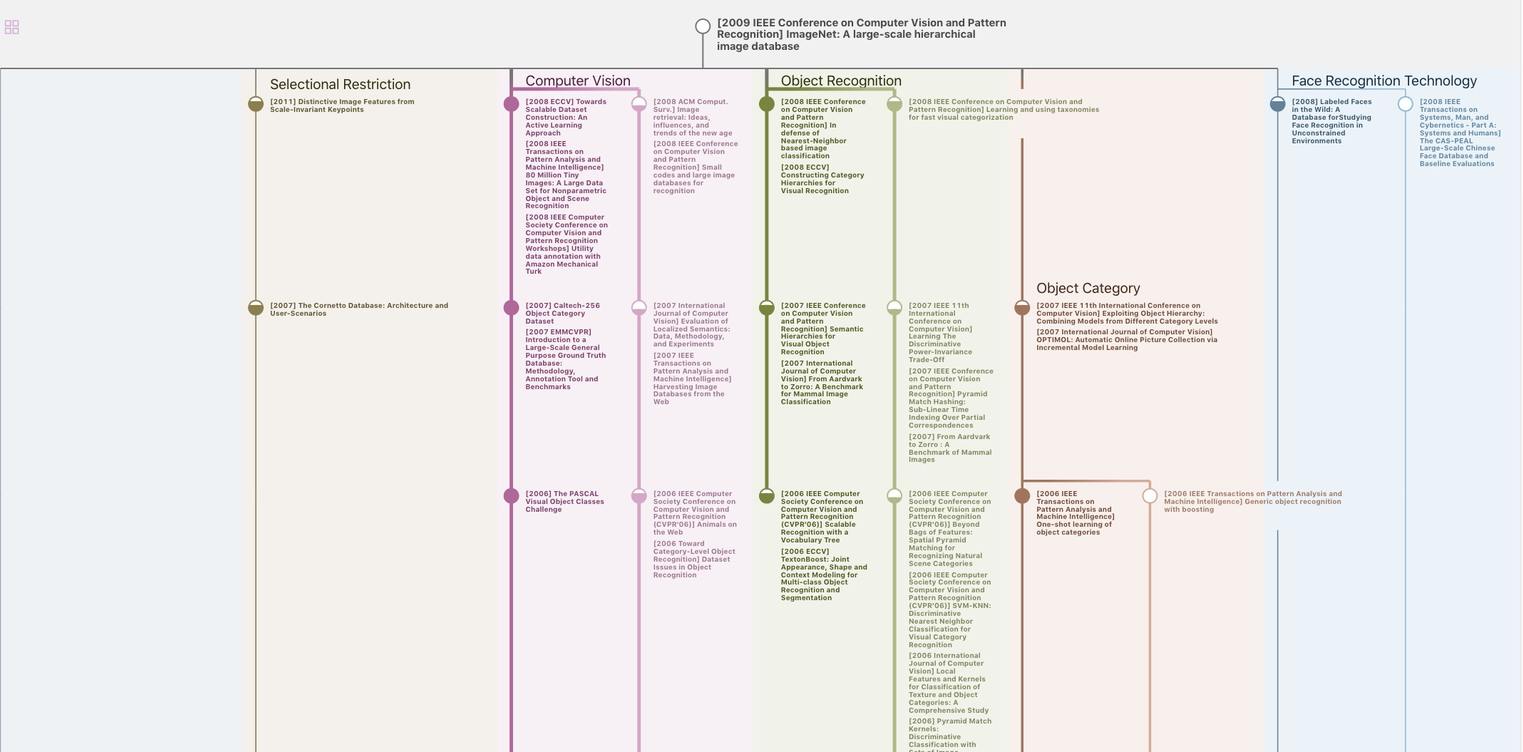
生成溯源树,研究论文发展脉络
Chat Paper
正在生成论文摘要