Two-sided Rank Consistent Ordinal Regression for Interpretable Music Key Recommendation.
International Conference on the Theory of Information Retrieval (ICTIR)(2022)
摘要
Model interpretability has attracted increasing attention in the IR community since it is important to ensure that end-users (decision-makers) correctly understand and consequently trust the functionality of the models. On the other hand, ordinal regression has been widely used in many ranking and prediction tasks, but it could not guarantee the rank consistent predictions for the output labels, which makes the predicted results hard to explain. Take the music key recommendation in karaoke as an example where a user could select a key ranging from -7 to +7 so that the song could meet the user's vocal competence for better performance. If the best key for a user to sing a song is -3, the keys smaller than -3 should be ranked in decreasing order. Similarly, the keys on the positive side should also be ranked in the decreasing order. To address this challenge, we propose a novel Two-sided Rank Consistent Ordinal Regression model. We show that the model is not only able to predict the key for the target song given the user's singing history, but it also has the theoretical guarantees for the two-sided rank-monotonicity. We train the model with a history encoder using the recurrent units and a key decoder using the Transformer. The experimental results on the real-world karaoke dataset demonstrate the effectiveness of our proposed model.
更多查看译文
AI 理解论文
溯源树
样例
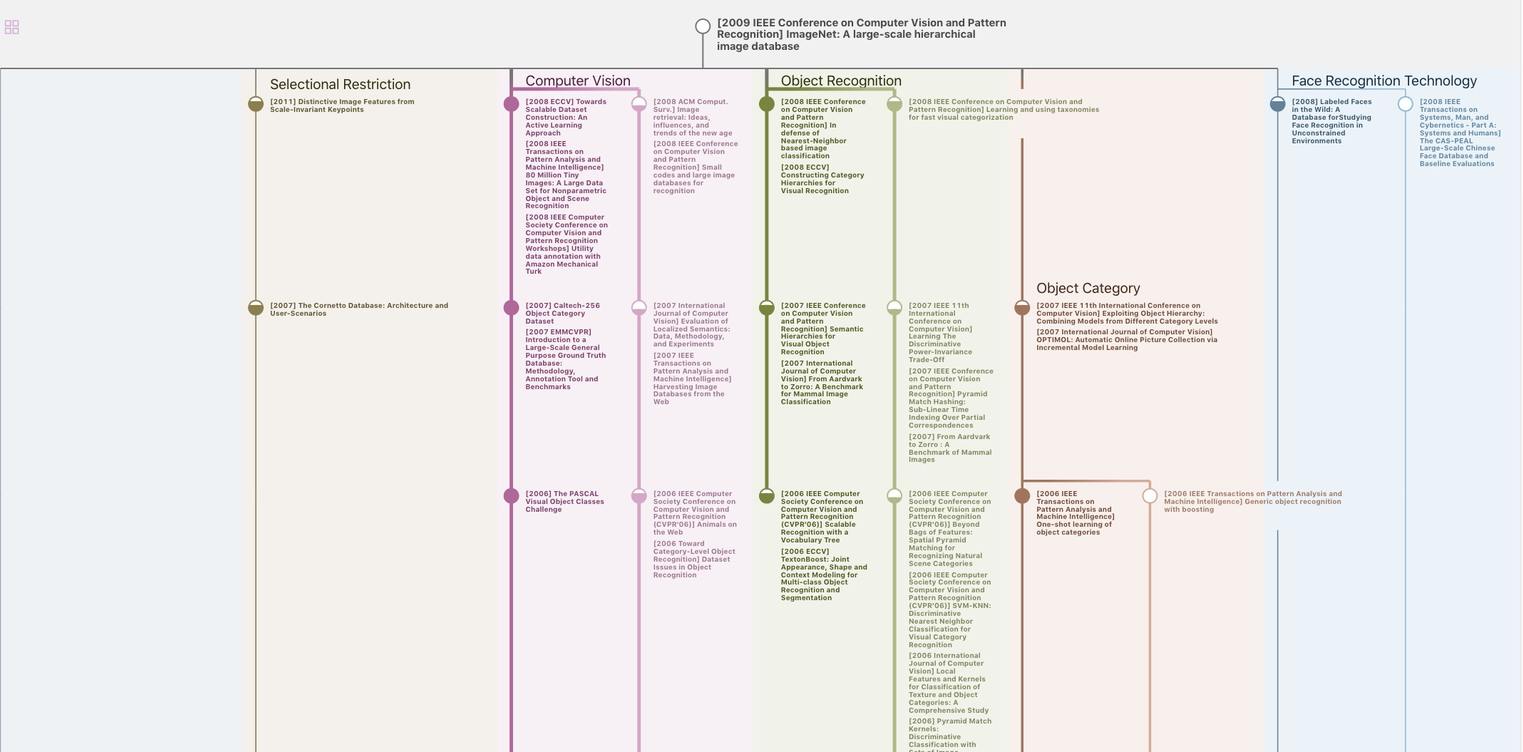
生成溯源树,研究论文发展脉络
Chat Paper
正在生成论文摘要