A general approach to fast online training of modern datasets on real neuromorphic systems without backpropagation
International Conference on Neuromorphic Systems (ICONS)(2022)
Abstract
We present parameter-multiplexed gradient descent (PMGD), a perturbative gradient descent framework designed to easily train emergent neuromorphic hardware platforms. We show its applicability to both analog and digital systems. We demonstrate how to use it to train networks with modern machine learning datasets, including Fashion-MNIST and CIFAR-10. Assuming realistic timescales and hardware parameters, our results indicate that PMGD could train a network on emerging hardware platforms orders of magnitude faster than the wall-clock time of training via backpropagation on a standard GPU/CPU.
MoreTranslated text
Key words
machine learning, neural networks, neuromorphic computing, emerging hardware
AI Read Science
Must-Reading Tree
Example
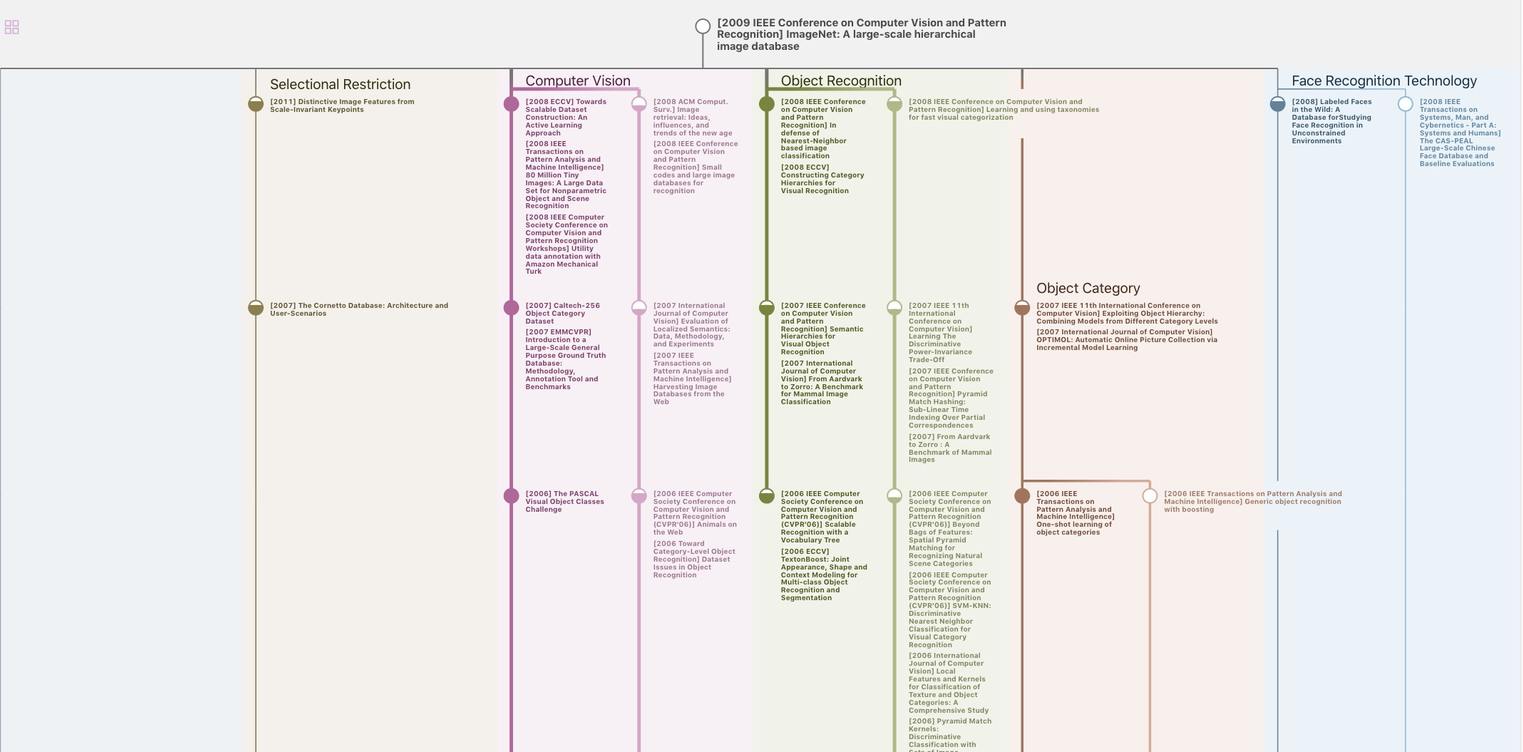
Generate MRT to find the research sequence of this paper
Chat Paper
Summary is being generated by the instructions you defined