Training Computational Models of Group Processes without Groundtruth: the Self- vs External Assessment's Dilemma.
International Conference on Multimodal Interaction (ICMI)(2022)
摘要
Supervised learning relies on the availability and reliability of the labels used to train computational models. In research areas such as Affective Computing and Social Signal Processing, such labels are usually extracted from multiple self- and/or external assessments. Labels are, then, either aggregated to produce a single groundtruth label, or all used during training, potentially resulting in degrading performance of the models. Defining a “true” label is, however, complex. Labels can be gathered at different times, with different tools, and may contain biases. Furthermore, multiple assessments are usually available for a same sample with potential contradictions. Thus, it is crucial to devise strategies that can take advantage of both self- and external assessments to train computational models without a reliable groundtruth. In this study, we designed and tested 3 of such strategies with the aim of mitigating the biases and making the models more robust to uncertain labels. Results show that the strategy based on weighting the loss during training according to a measure of disagreement improved the performances of the baseline, hence, underlining the potential of such an approach.
更多查看译文
AI 理解论文
溯源树
样例
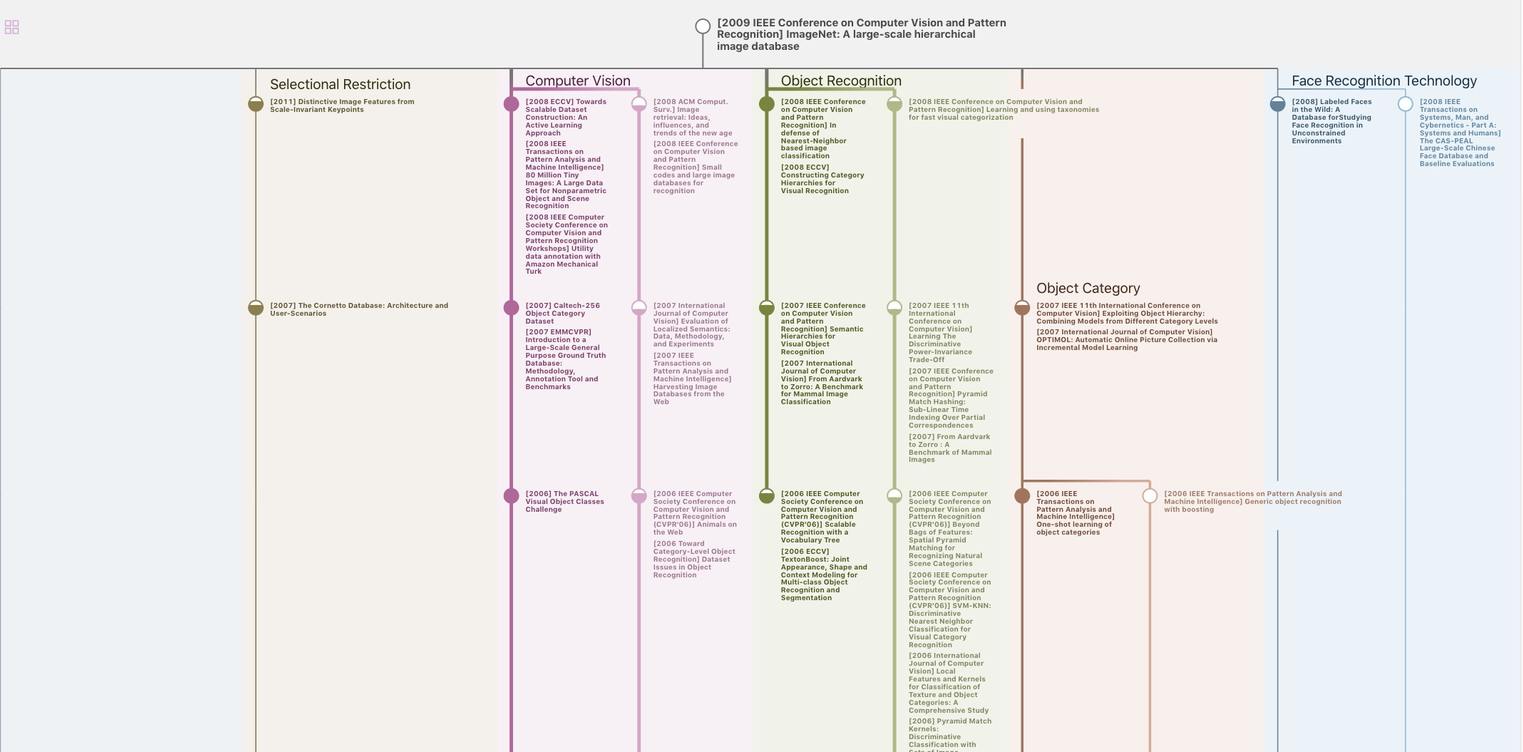
生成溯源树,研究论文发展脉络
Chat Paper
正在生成论文摘要