On the Performance of Deep Learning Models for Respiratory Sound Classification Trained on Unbalanced Data.
Iberian Conference on Pattern Recognition and Image Analysis (IbPRIA)(2022)
摘要
The detection of abnormal breath sounds with a stethoscope is important for diagnosing respiratory diseases and providing first aid. However, accurate interpretation of breath sounds requires a great deal of experience on the part of the clinician. In the past few years, a number of deep learning models have been proposed to automate lung classification tasks in physical examination. Unfortunately, acquiring accurately annotated data for this problem is not straightforward and important issues arise, as the available examples of abnormal and normal sounds usually differ substantially. This work provides a comprehensive analysis of deep learning models making use of different class balancing methods during training, considering multiple network architectures and audio input features. The results show that good performance is achievable when applying random oversampling and a convolutional neural network operating over Mel-frequency cepstral coefficient (MFCC) representations.
更多查看译文
关键词
Deep learning,Class imbalance,Respiratory diseases
AI 理解论文
溯源树
样例
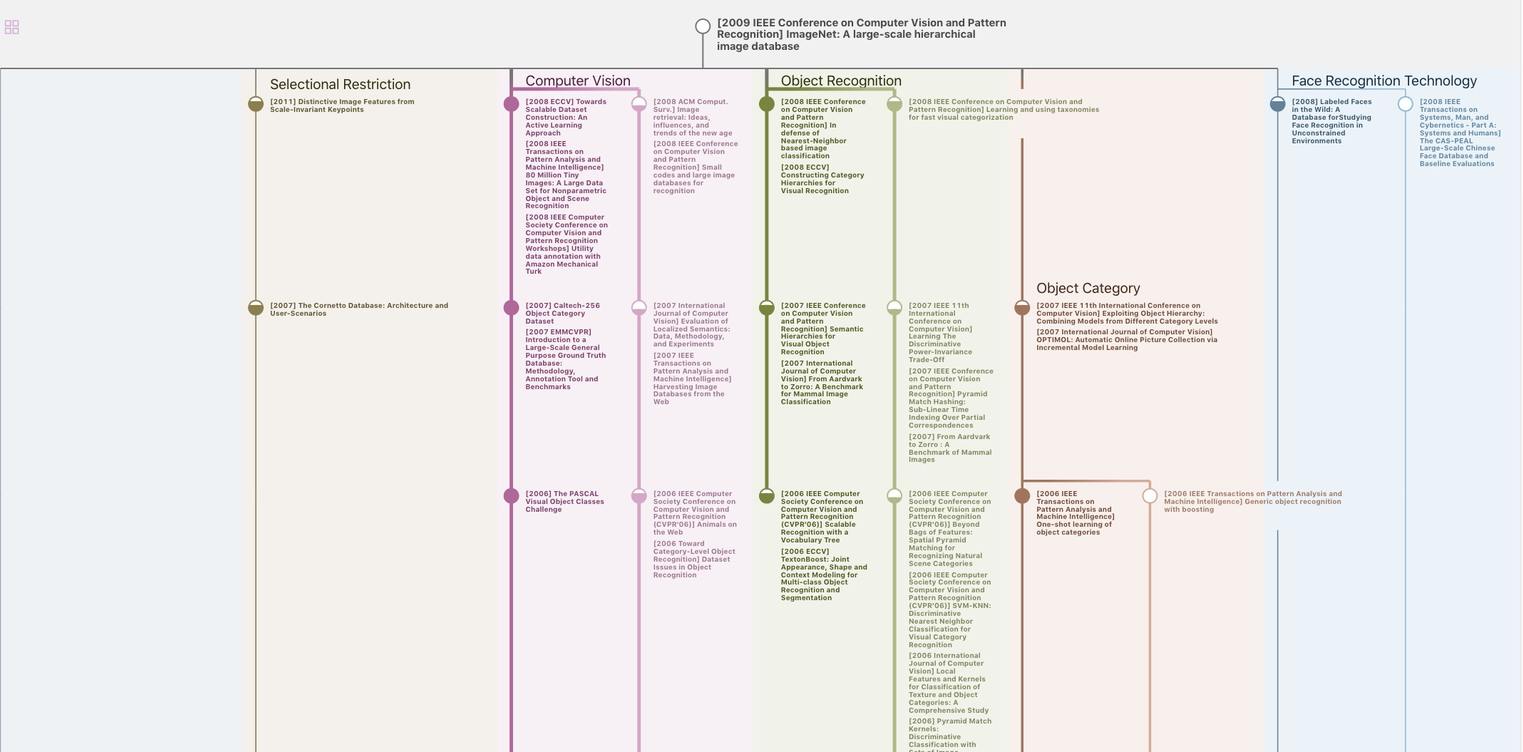
生成溯源树,研究论文发展脉络
Chat Paper
正在生成论文摘要