An Interpretable Deep Classifier for Counterfactual Generation
3RD ACM INTERNATIONAL CONFERENCE ON AI IN FINANCE, ICAIF 2022(2022)
Abstract
Counterfactual explanation has been the core of interpretable machine learning, which requires a trained model to be able to not only infer but also justify its inference. This problem is crucial in many fields, such as fintech and the healthcare industry, where accurate decisions and their justifications are equally important. Many studies have leveraged the power of deep generative models for counterfactual generation. However, most focus on vision data and leave the latent space unsupervised. In this paper, we propose a new and general framework that uses a supervised extension to the Variational Auto-Encoder (VAE) with Normalizing Flow (NF) for simultaneous classification and counterfactual generation. We show experiments on two tabular financial data-sets, Lending Club (LCD) and Give Me Some Credit (GMC), which show that the model can achieve a state-of-art level prediction accuracy while also producing meaningful counterfactual examples to interpret and justify the classifier's decision.
MoreTranslated text
Key words
supervised autoencoders,intepretable deep learning,explainability,counterfactual methods
AI Read Science
Must-Reading Tree
Example
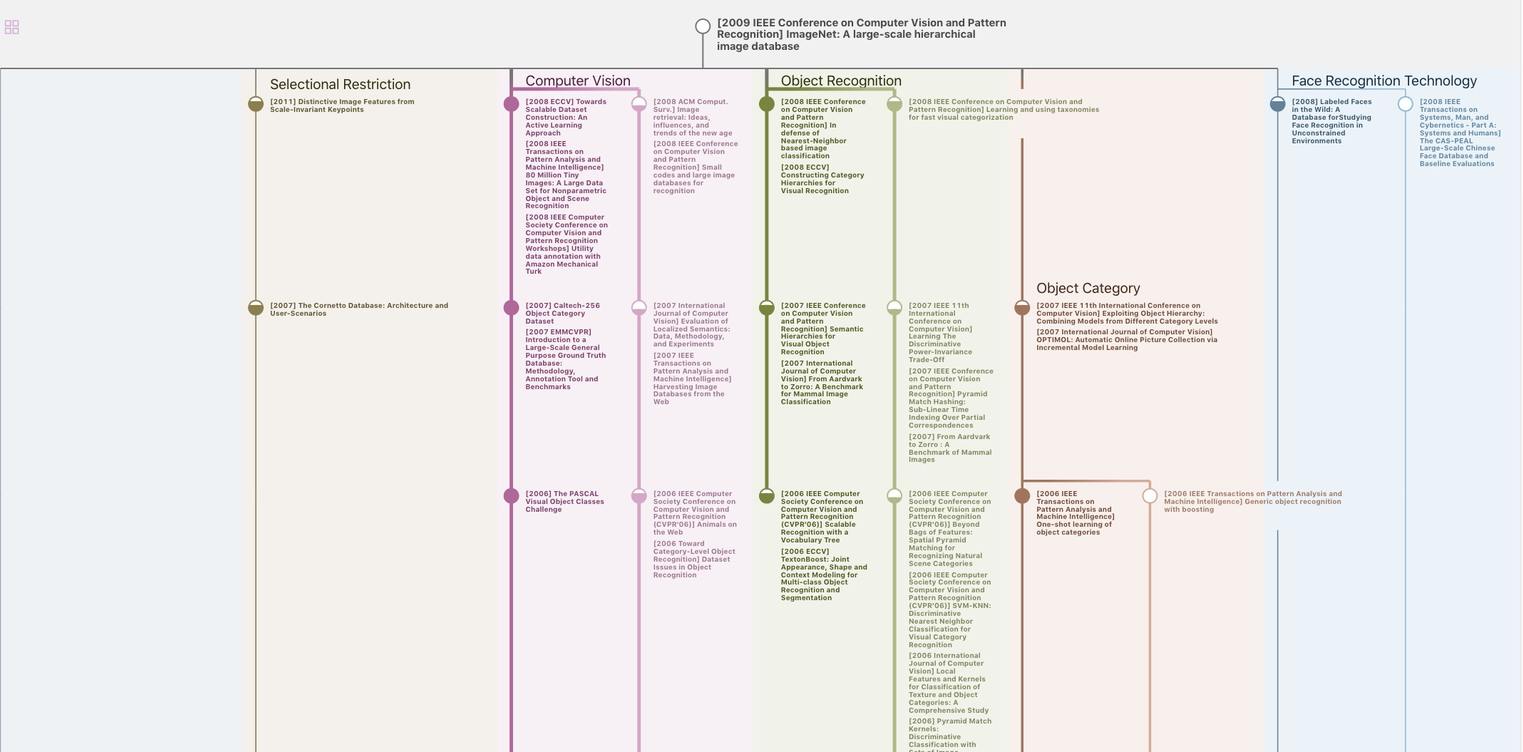
Generate MRT to find the research sequence of this paper
Chat Paper
Summary is being generated by the instructions you defined