Empirical Evaluation of Machine Learning Ensembles for Rumor Detection.
International Conference on Human-Computer Interaction (HCI International)(2022)
摘要
Rumor detection is a recent and quite active topic of multi-disciplinary research due to its evident impact on society, which can even result in physical harm to people. Despite its broad definition and scope, most existing research focuses on Twitter, as it makes the problem a bit more tractable from the point of view of information gathering, processing, and further retrieval of social network features. This paper presents an empirical study of novel machine learning ensembles for the rumor classification task on Twitter. As it has been observed that certain neural models perform better for specific veracity labels, we present a study on how the combination of such classifiers in different kinds of ensemble results in a new classifier that has better performance. Using benchmark data, we evaluate three groups of models (two groups of deep neural networks and a control group of classical machine learning methods). In addition, we study the performance of three ensemble strategies: Bagging, Stacking, and Simple Soft Voting. After varying several parameters of the models, such as the number of hidden units and dropout, among others experimental factors, our study shows that the LSTM, Stacked LSTM (S-LSTM), Recurrent Convolutional Neural Networks (RCNN), and Bidirectional Gated Recurrent Unit (Bi-GRU) yields the best results reaching an accuracy of 0.93 and an average precision of 0.97.
更多查看译文
关键词
Rumor detection, Fake news, Ensembles, Machine learning, Deep learning
AI 理解论文
溯源树
样例
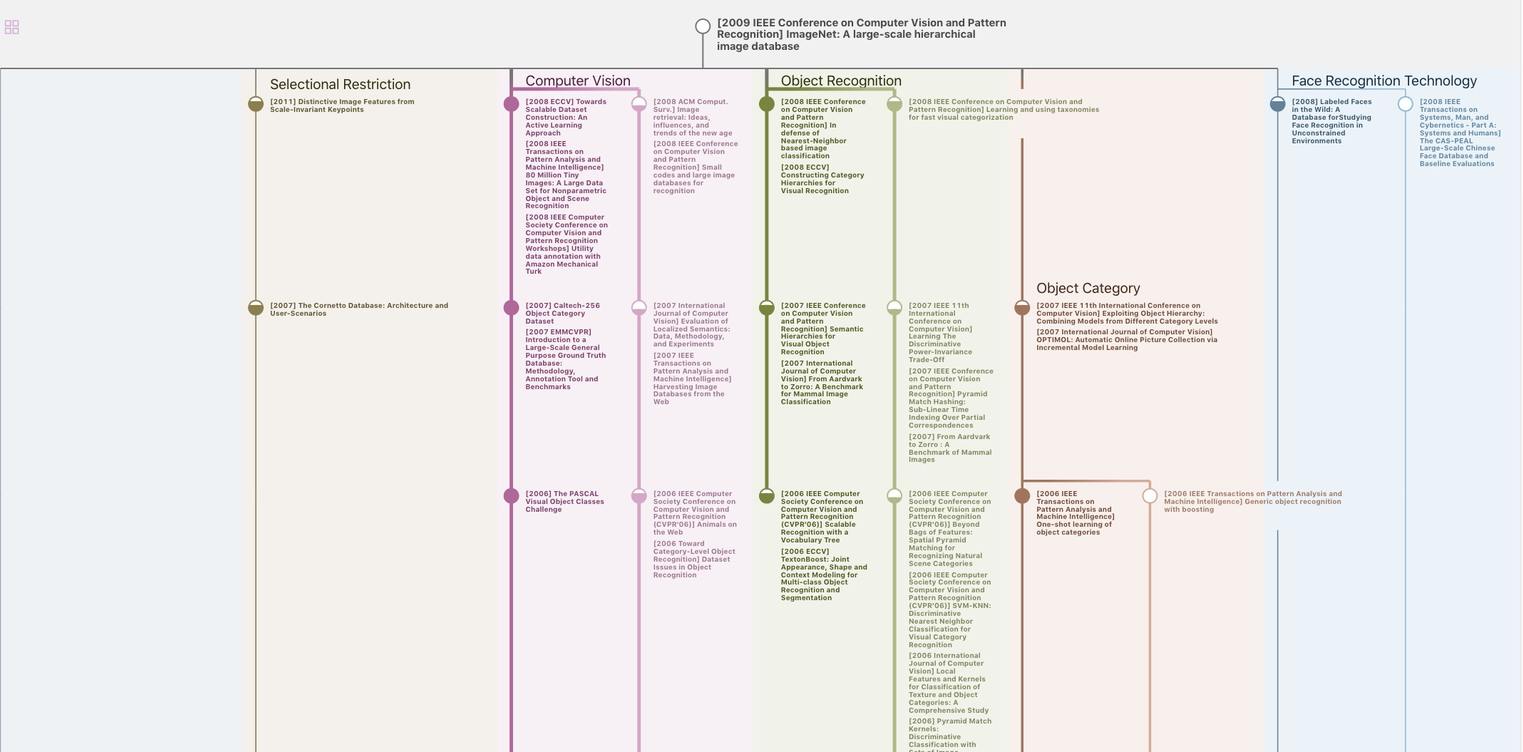
生成溯源树,研究论文发展脉络
Chat Paper
正在生成论文摘要