Fisher Information Neural Estimation.
European Signal Processing Conference (EUSIPCO)(2022)
摘要
Fisher information is a fundamental quantity in information theory and signal processing. A direct analytical computation of the Fisher information is often infeasible or intractable due to the lack or sophistication of statistical models. In this paper, we propose a Fisher Information Neural Estimator (FINE) which is computationally efficient, highly accurate, and applicable for both cases of deterministic and random parameters. The proposed method solely depends on measured data and does not require knowledge or an estimate of the probability density function and is therefore universally applicable. We validate our approach using some experiments and compare with existing works. Numerical results show the high efficacy and low-computational complexity of the proposed estimation approach.
更多查看译文
关键词
probability density function,deterministic parameters,random parameters,FINE,signal processing,direct analytical computation,information theory,Fisher information neural estimation
AI 理解论文
溯源树
样例
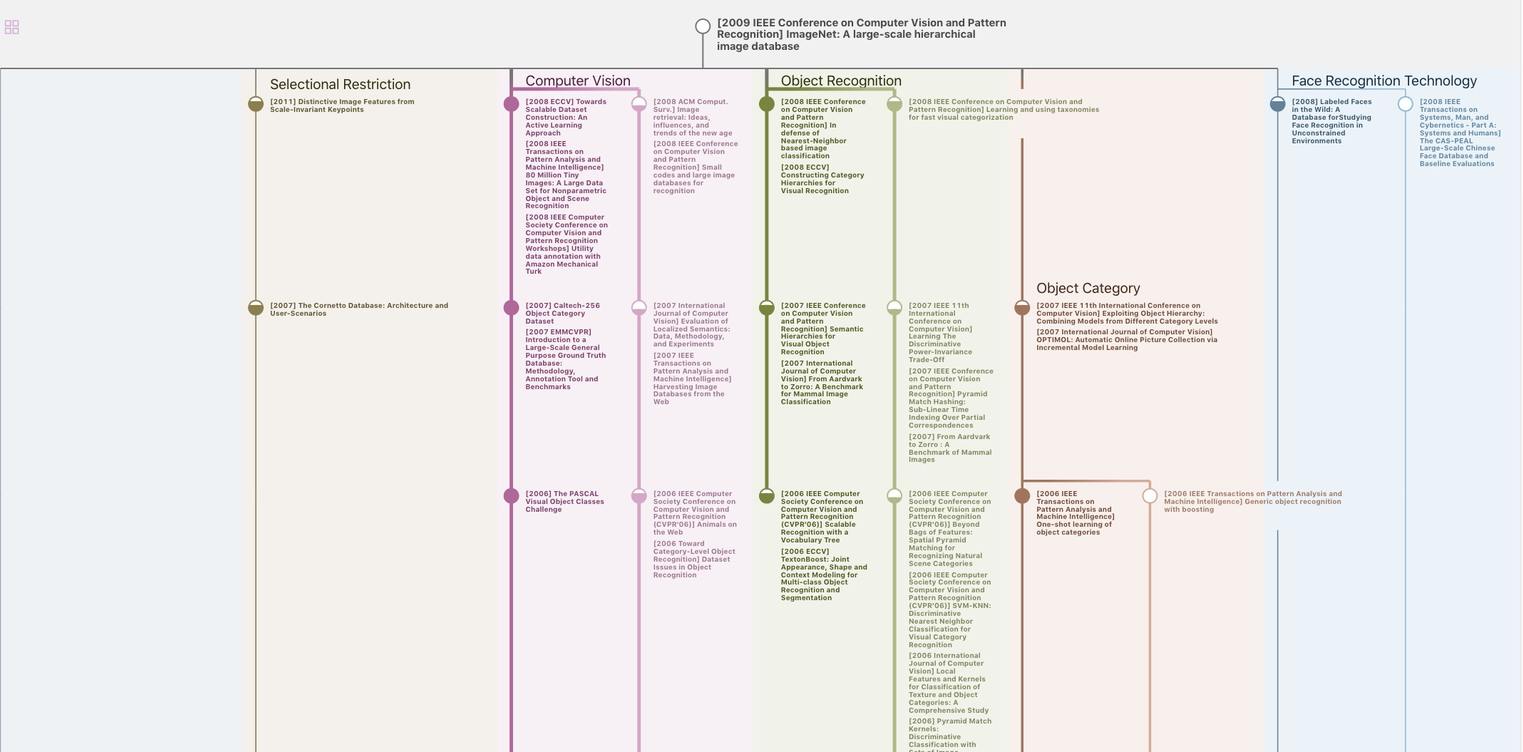
生成溯源树,研究论文发展脉络
Chat Paper
正在生成论文摘要