Convergent Distributed Actor-Critic Algorithm Based on Gradient Temporal Difference.
European Signal Processing Conference (EUSIPCO)(2022)
摘要
In this paper a new distributed off-policy Actor-Critic algorithm for reinforcement learning is proposed. It is composed of the Gradient Temporal Difference GTD(1) algorithm at the Critic stage, and a complementary consensus-based exact policy gradient algorithm at the Actor stage, derived from the global objective in the form of a sum of weighted local state-value functions. Weak convergence of the algorithm to the invariant set of a corresponding attached ODE is demonstrated under mild conditions. An experimental verification of the algorithm properties is presented, showing that the algorithm can represent an efficient tool for practice, enabling parallel execution and fusion of local exploration spaces.
更多查看译文
关键词
gradient temporal difference,actor-critic
AI 理解论文
溯源树
样例
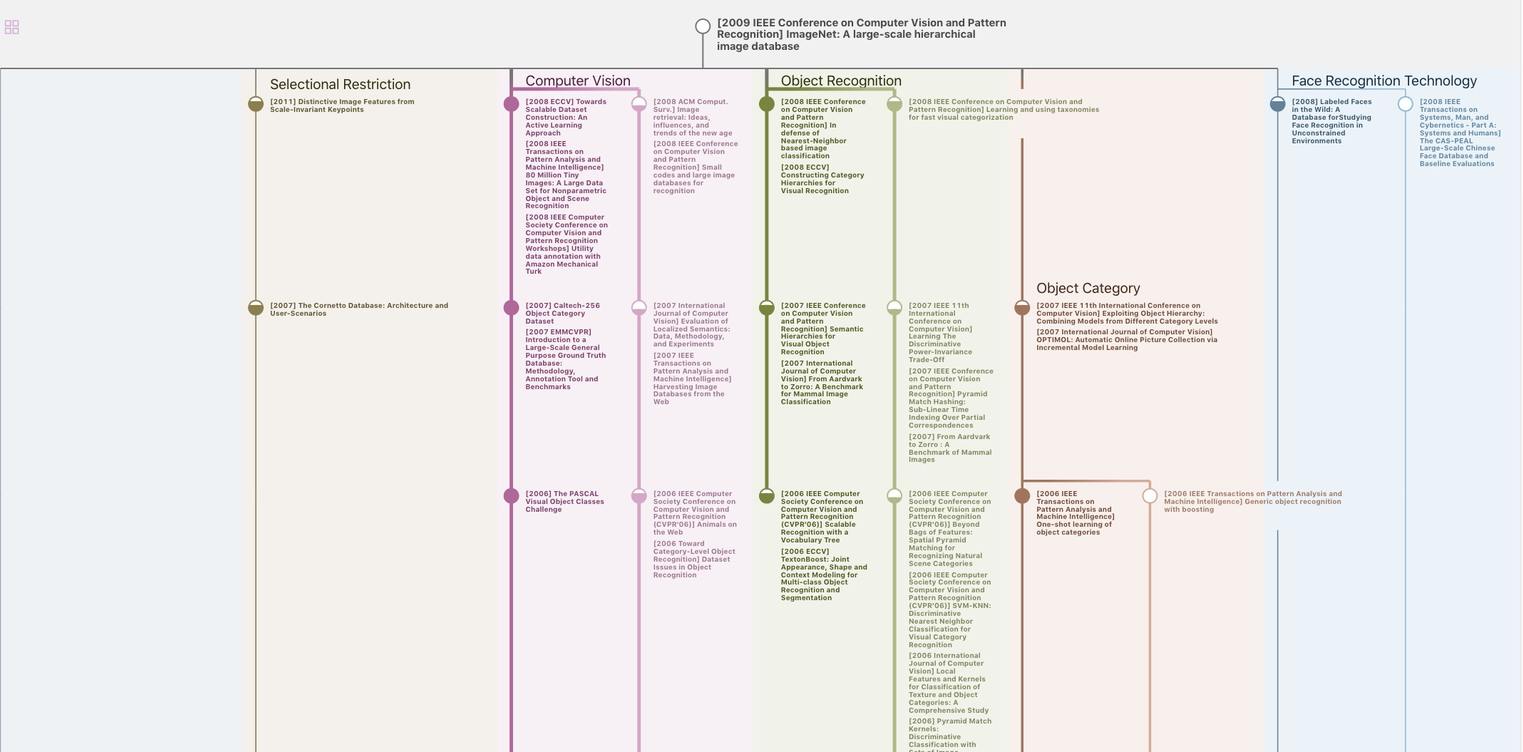
生成溯源树,研究论文发展脉络
Chat Paper
正在生成论文摘要