Improving Style Randomization Via Domain-specific Feature Reweighting for Domain Generalization.
2022 30th European Signal Processing Conference (EUSIPCO)(2022)
Abstract
Despite the steady progress of neural networks, their applicability to the real world is limited because they often fail to generalize to unseen domains. To overcome this challenge, recent studies have proposed various methods for improving out-of-distribution generalizations. However, these methods require complex architectures or additional learning strategies that involve non-trivial efforts. On the other hand, style randomization, a feature-level augmentation strategy, can increase the networks' generalization capability simply by diversifying the source domains. In this paper, we focus on improving the internal process of style randomization to produce more diverse samples that help networks learn domain-invariant representation. To this end, we propose a novel feature-level augmentation strategy that generates diverse samples for contents as well as styles. Our method can be implemented very simply but outperforms all compared methods in experiments on the DomainBed benchmark.
MoreTranslated text
Key words
Domain Generalization
AI Read Science
Must-Reading Tree
Example
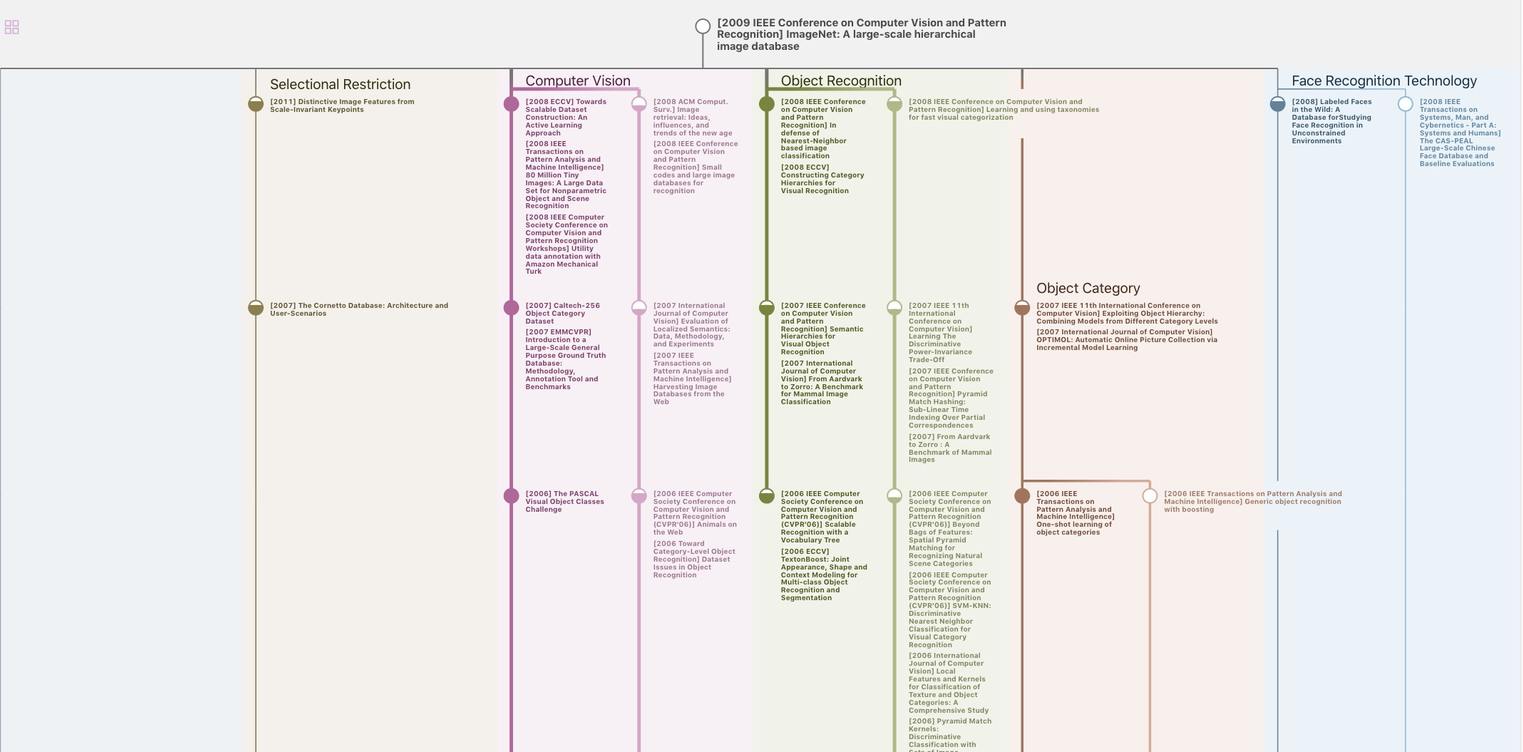
Generate MRT to find the research sequence of this paper
Chat Paper
Summary is being generated by the instructions you defined