Towards interpreting deep learning models for industry 4.0 with gated mixture of experts
European Signal Processing Conference (EUSIPCO)(2022)
摘要
In this work, we propose to use the Gated Mixture of Experts to interpret a deep learning model trained on industrial data. Unlike monolithic deep learning models, gated modular neural networks enable to decompose parts of the models in a way that may potentially be interpreted by domain experts or users. We first propose to transform a model that gives state-of-the-art performances on a standard industrial benchmark according to this paradigm. The model aims at predicting the remaining useful life of an asset in the field of prognostics and health management for industry 4.0. Then, we experimentally validate that the performances of the transformed model are not degraded and that the resulting model segments and clusters the data streams according to an emerging concept that reflects previously published analyses by experts on this dataset, even though such a concept has never been introduced at training time. This work thus confirms the interpretable properties of the Gated Mixture of Experts in a new domain. We further study some potential weaknesses of this paradigm, in particular the excessive variability of the resulting decomposition across experiments, and we propose to modify the loss with a new knowledge-based constraint term that encodes a known prior distribution of latent concepts in the data. We show that this term enables greater control over the Gated Mixture of Experts that results in a decomposition of significantly better quality on our benchmark.
更多查看译文
关键词
Interpretability,Gated mixture of experts,Prog-nostic,Deep learning,LSTM,CMAPSS
AI 理解论文
溯源树
样例
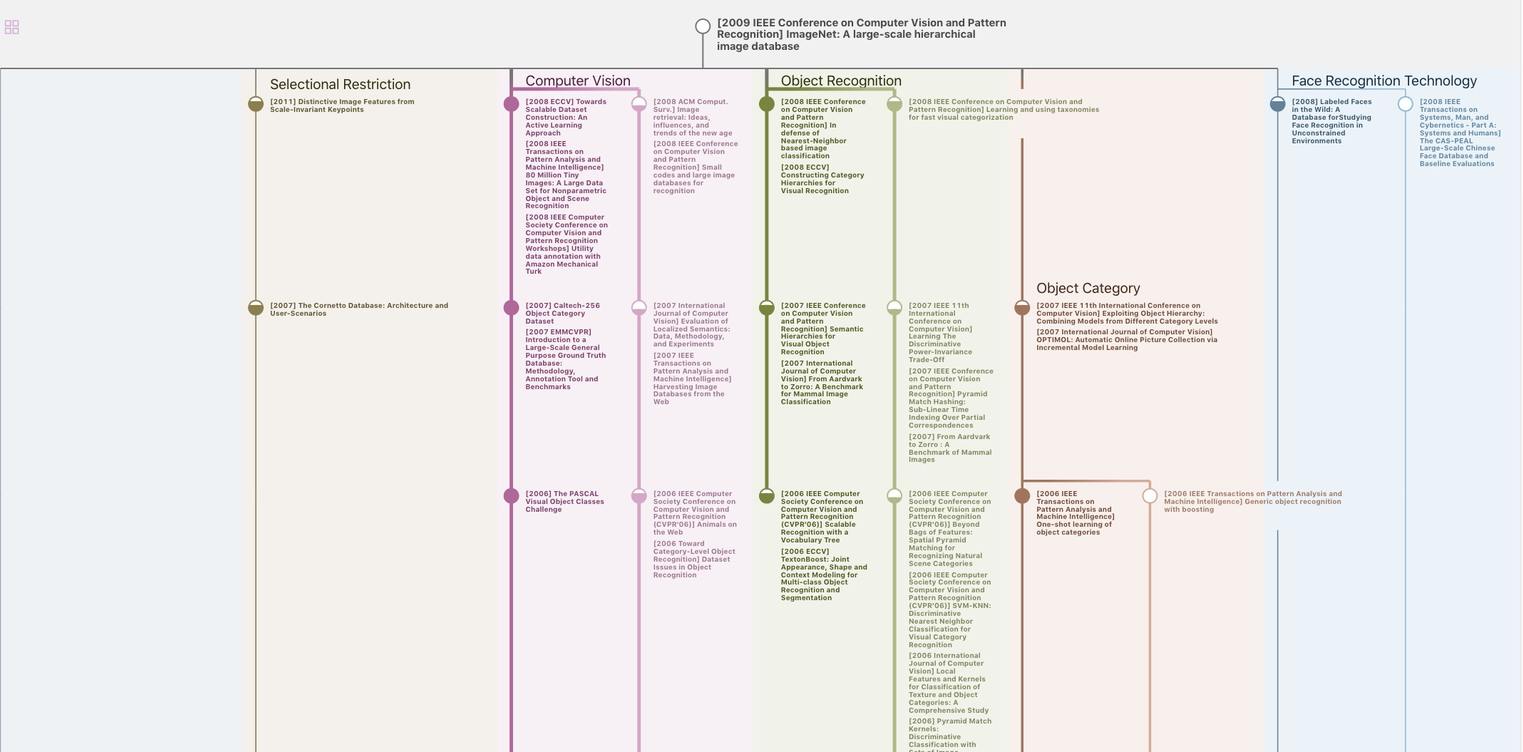
生成溯源树,研究论文发展脉络
Chat Paper
正在生成论文摘要