Automatic selection of latent variables in variational auto-encoders.
European Signal Processing Conference (EUSIPCO)(2022)
摘要
Variational auto-encoders (VAEs) are powerful generative neural networks based on latent variables. They aim to capture the distribution of a dataset, by building an informative space composed of a reduced number of variables. However, the size of this latent space is both sensitive and difficult to adjust. Thus, most state-of-the-art architectures experience either disentanglement issues, or, at the opposite, posterior collapse. Both phenomena impair the interpretability of the latent variables. In this paper, we propose a variant of the VAE which is able to automatically determine the informative components of the latent space. It consists in augmenting the vanilla VAE with auxiliary variables and defining a hierarchical model which favors that only a subset of the latent variables are used for the encoding. We refer to it as NGVAE. We compare its performance with other auto-encoder based architectures.
更多查看译文
关键词
latent variables,automatic selection,auto-encoders
AI 理解论文
溯源树
样例
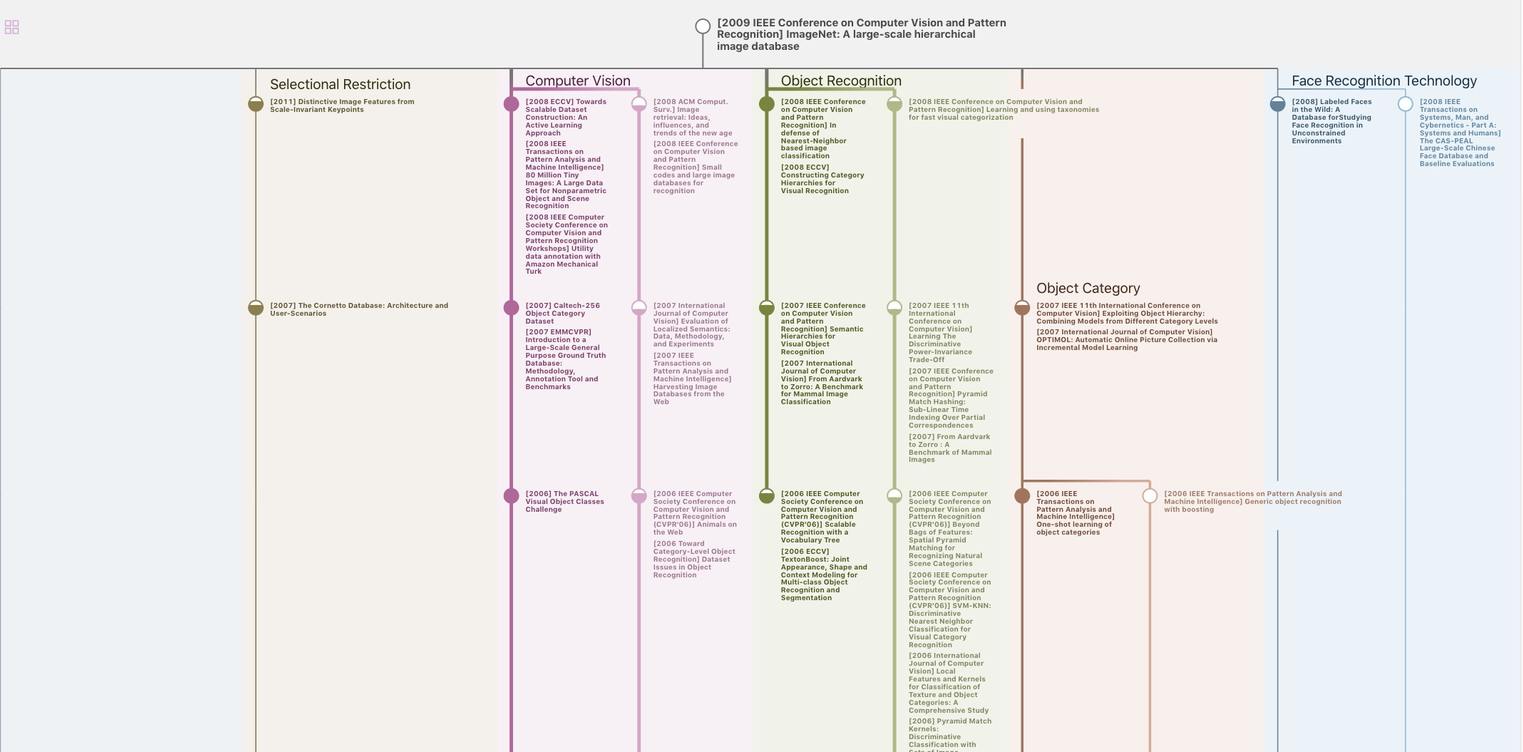
生成溯源树,研究论文发展脉络
Chat Paper
正在生成论文摘要