A Multi-Scale Context Aggregation Enriched MLP-Mixer Model for Oral Cancer Screening from Oral Sub-epithelial Connective Tissues.
European Signal Processing Conference (EUSIPCO)(2022)
摘要
This treatise proposes a dilated convolutional multilayer perceptron (MLP)-mixer model (hereafter referred to as DiCoMLP-Mixer) for oral connective tissue (OCT) grading. The proposed DiCoMLP-Mixer framework comprehends dense multiscale contextual feature representation by enabling exponential receptive field expansion without sacrificing resolution via dilated convolutions. The MLP-mixer backbone in the DiCoMLP-Mixer architecture leverages the attention mechanism of a transformer model for saliency abstraction in oral mucosa histopathological images (OMHIs) by the spatial encoding of OMHI patches. This study focuses on the oral mucosa's sub-epithelium region, which has considerable clinical significance but is understudied in the literature. DiCoMLP-Mixer's exhaustive experimental validation on two OCT layers of the sub-epithelium, namely papillary (L-1) and reticular (L-2) for oral cancer (OC) grading, classifying the three major oral potentially malignant disorders (OPMDs) and OC from healthy OCTs. The ablation study with the existing MLP-mixer model evinces enhanced OC screening performance, while Grad-CAM heatmaps exhibit DiCoMLP-Mixer's consistent clinical saliency for precise OC detection.
更多查看译文
关键词
oral cancer,aggregation,multi-scale,mlp-mixer,sub-epithelial
AI 理解论文
溯源树
样例
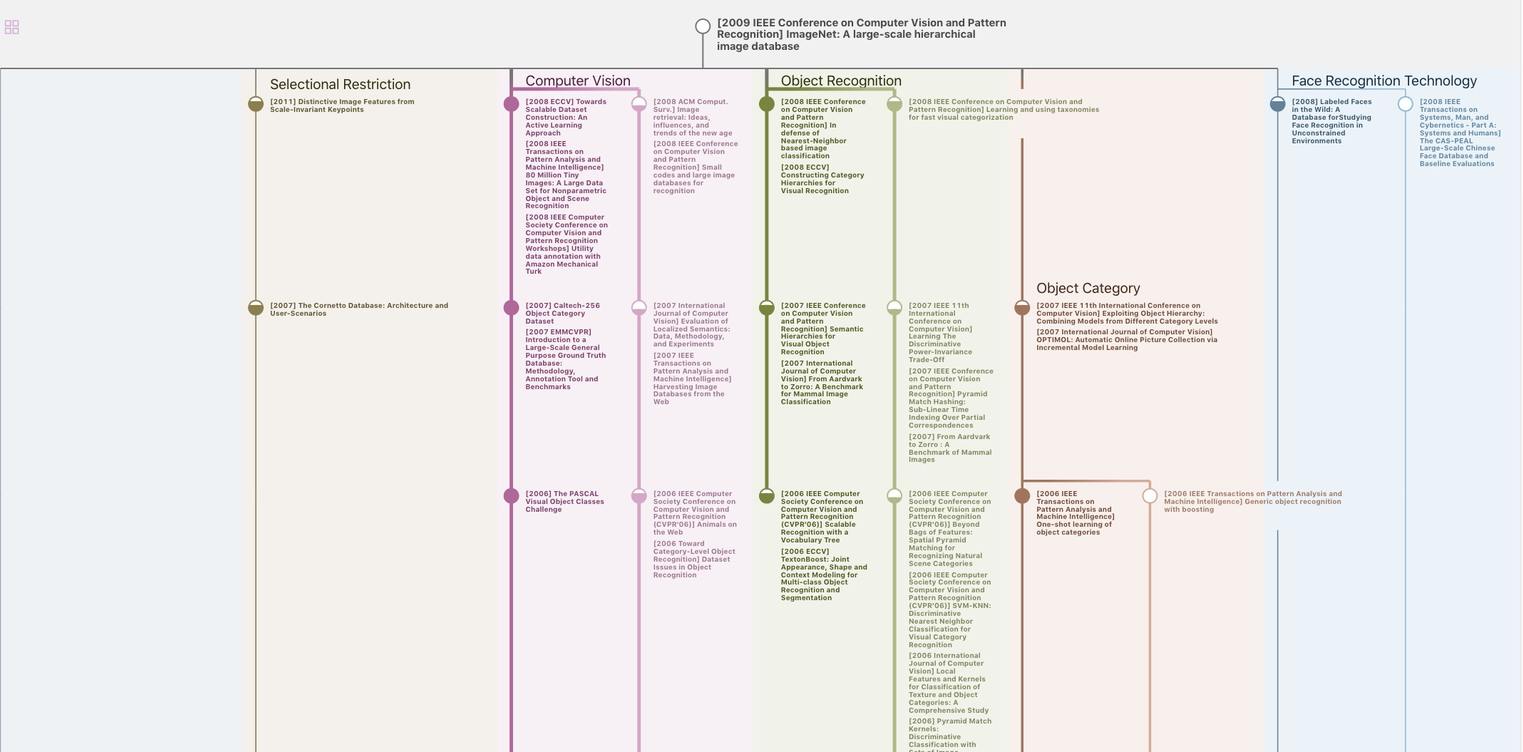
生成溯源树,研究论文发展脉络
Chat Paper
正在生成论文摘要