Sim2Real image translation to improve a synthetic dataset for a bin picking task.
IEEE International Conference on Emerging Technologies and Factory Automation (ETFA)(2022)
摘要
The use of synthetic data is a promising solution to the problem of the availability of real data needed for the development of robotic systems. However, the precision of the systems trained on synthetic data tends to decrease when they are deployed in the real-life scenarios, which happens due to the disparities between the artificial data and the real world. Therefore, efficient methods for Sim2Real translation are much needed for further progress in robotics. In our study, we use Generative Adversarial Networks (GANs) to generate more photorealistic data from the synthetic data created for training deep neural networks to handle a bin picking task. As a result, object detectors trained on the images improved with the GANs with the optimal approach to image translation demonstrate better performance than their counterparts trained on the original synthetic
更多查看译文
关键词
Sim2Real, image translation, synthetic data, CycleGAN, bin picking
AI 理解论文
溯源树
样例
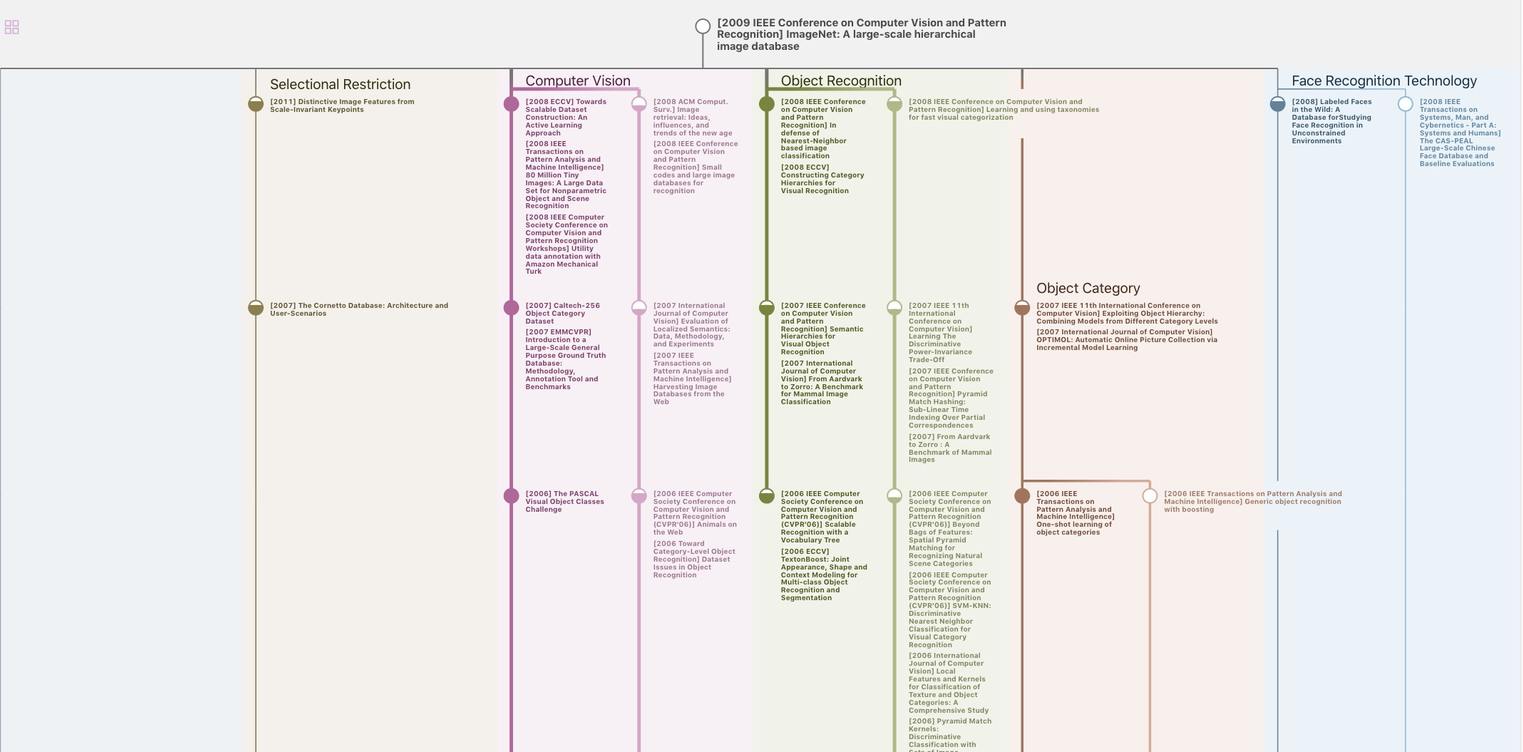
生成溯源树,研究论文发展脉络
Chat Paper
正在生成论文摘要