Modeling and generating synthetic anomalies for energy and power time series.
Energy-Efficient Computing and Networking (e-Energy)(2022)
摘要
With the development of the smart grid, the number of recorded energy and power times series increases noticeably. This increase allows for the automation of smart grid applications such as load forecasting and load management. This automation, however, requires data that only represents the typical behavior of the system. To ensure that such data is available, detecting the anomalies often present in recorded data is important. As a result, anomaly detection methods are a recent research topic. However, their development is often limited by undefined anomaly characteristics and a lack of labeled anomalous data. To overcome this challenge, we propose a method that generates synthetic anomalies based on real-world anomalies that can be inserted into energy and power time series. For this, we analyze real energy and power time series to identify four types of commonly occurring anomalies. Given the identified anomaly types, we formally model each type and use these models to insert synthetic anomalies of each type into arbitrary energy or power time series. We show that our method is not only capable of generating synthetic anomalies with real-world properties, but also beneficial for training supervised anomaly detection methods.
更多查看译文
AI 理解论文
溯源树
样例
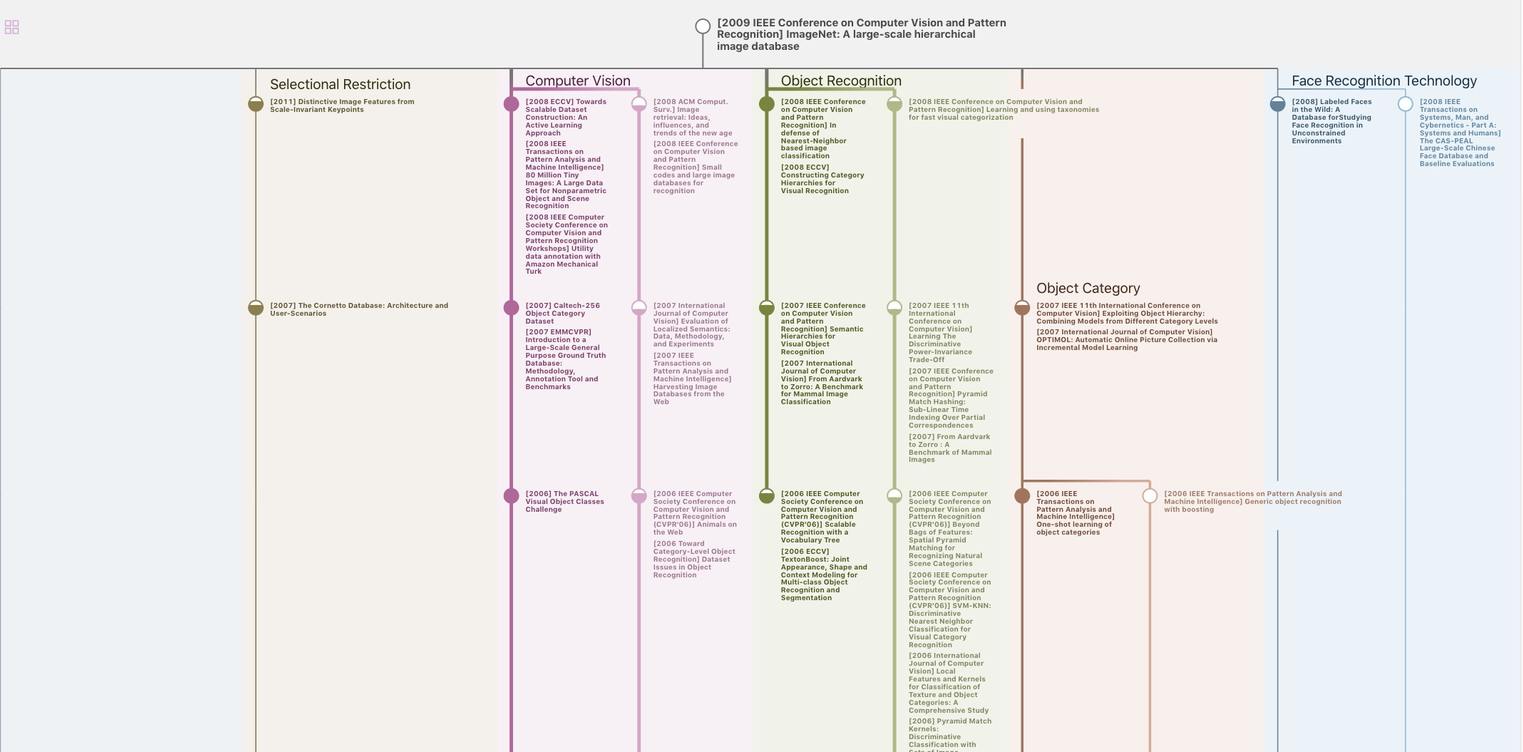
生成溯源树,研究论文发展脉络
Chat Paper
正在生成论文摘要