Semi-supervised Change Point Detection Using Active Learning.
International Conference on Discovery Science (DS)(2022)
摘要
The goal of change point detection (CPD) is to find abrupt changes in the underlying state of a time series. Currently, CPD is typically tackled using fully supervised or completely unsupervised approaches. Supervised methods exploit labels to find change points that are as accurate as possible with respect to these labels, but have the drawback that annotating the data is a time-consuming task. In contrast, unsupervised methods avoid the need for labels by making assumptions about how changes in the underlying statistics of the data correlate with changes in a time series' state. However, these assumptions may be incorrect and hence lead to identifying different change points than a user would annotate. In this paper, we propose an approach in between these two extremes and present AL-CPD, an algorithm that combines active and semi-supervised learning to tackle CPD. AL-CPD asks directed queries to obtain labels from the user and uses them to eliminate incorrectly detected change points and to search for new change points. Using an empirical evaluation on both synthetic and real-world datasets, we show that our algorithm finds more accurate change points compared to existing change point detection methods.
更多查看译文
关键词
active learning,detection,change,semi-supervised
AI 理解论文
溯源树
样例
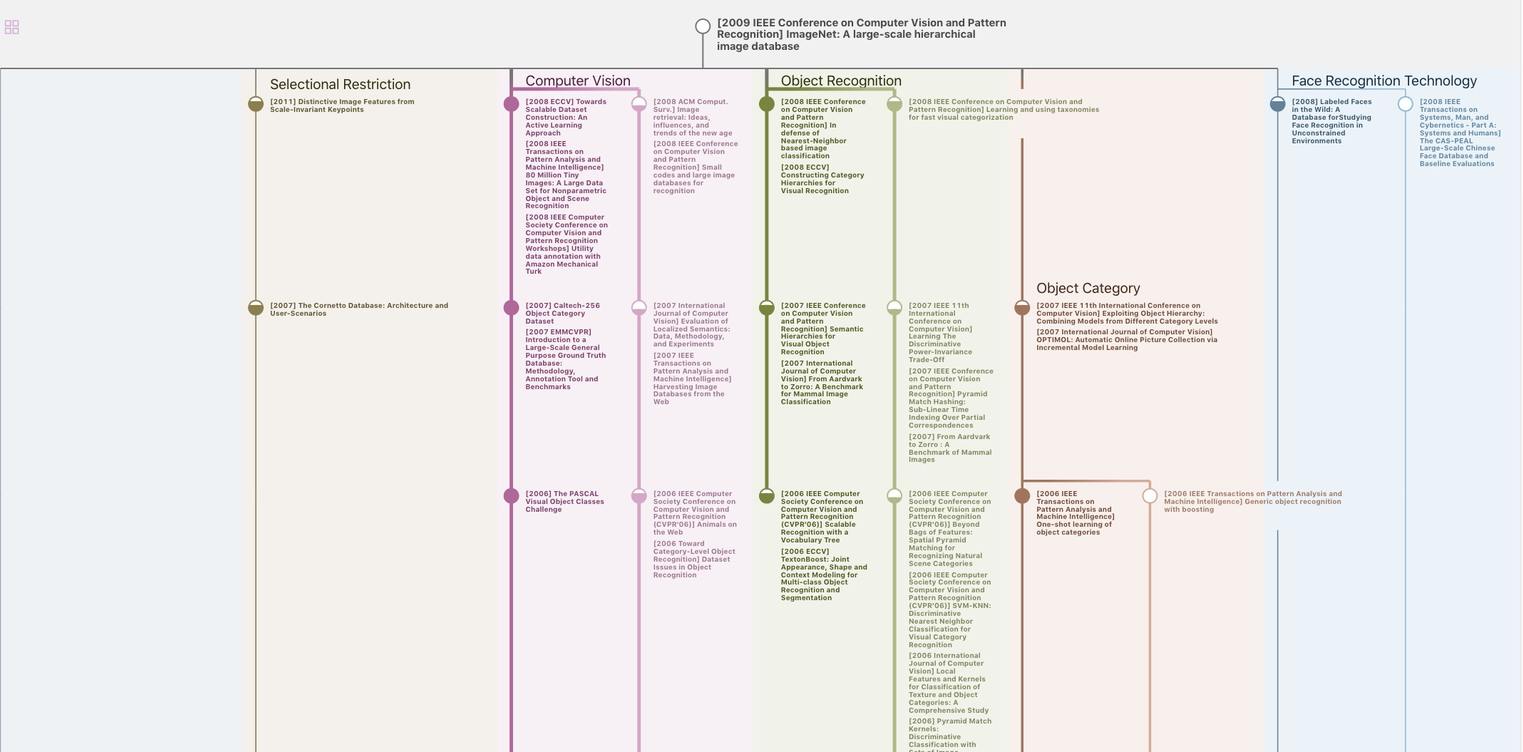
生成溯源树,研究论文发展脉络
Chat Paper
正在生成论文摘要