MCoM: A Semi-Supervised Method for Imbalanced Tabular Security Data.
Database Security (DBSec)(2022)
摘要
MCoM (Mixup Contrastive Mixup) is a new semi-supervised learning methodology that innovates a triplet minty) data augmentation approach to address the imbalanced data problem in tabular security data sets. Tabular data sets in cybersecurity domains are widely known to pose challenges for machine learning because of their heavily imbalanced data (e.g., a small number of labeled attack samples buried in a sea of mostly benign, unlabeled data). Semi-supervised learning leverages a small subset of labeled data and a large subset of unlabeled data to train a learning model. While semi-supervised methods have been well studied in image and language domains, in security domains they remain underutilized, especially on tabular security data sets which pose especially difficult contextual information loss and balance challenges for machine learning. Experiments applying MCoM to collected security data sets show promise for addressing these challenges, achieving state-of-the-art performance compared with other methods.
更多查看译文
关键词
Semi-Supervised Learning,Contrastive Learning,Tabular Data Sets,Security Data Sets
AI 理解论文
溯源树
样例
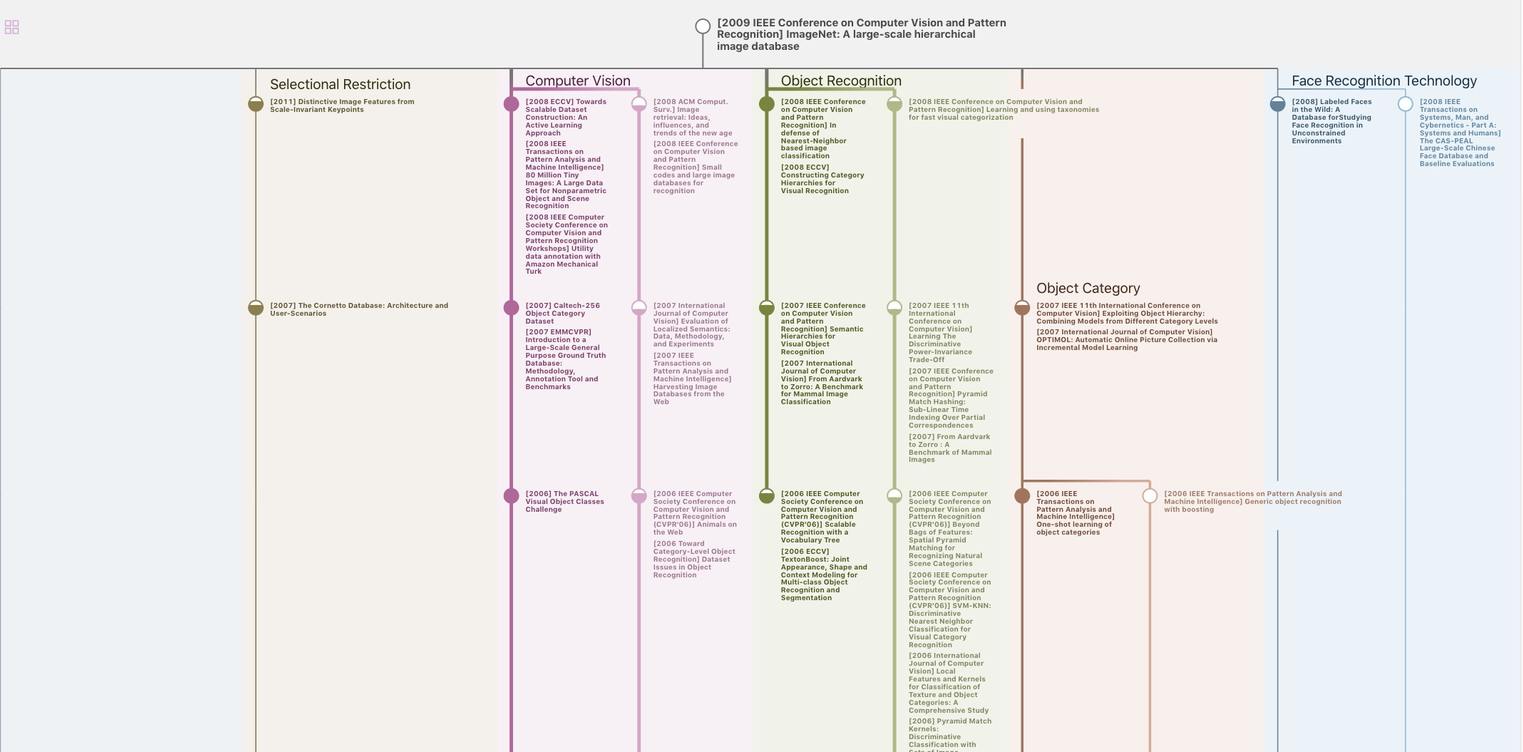
生成溯源树,研究论文发展脉络
Chat Paper
正在生成论文摘要