Approximate and Interactive Processing of Aggregate Queries on Knowledge Graphs: A Demonstration.
International Conference on Information and Knowledge Management (CIKM)(2022)
摘要
This paper demonstrates AGQ [26] - our system for approximate and interactive processing of ag gregate q ueries on knowledge graphs (KGs), e.g., " what is the average price of cars produced in Germany? " One can support aggregate queries based on factoid queries, e.g., " find all cars produced in Germany ", by applying an aggregate operation on factoid queries' answers. However, this straightforward method is problematic since both the accuracy and efficiency of factoid query processing would impact the performance of aggregate queries. Moreover, returning a one-time, exact result might add computation overhead and hinder users' engagement and interactivity. To this end, we design a system, called AGQ which employs a "sampling-estimation" model to answer aggregate queries over KGs. This is the first work to provide an approximate aggregate result with effective and interactive accuracy guarantees, and without relying on factoid queries. Our demonstration highlights (1) a novel semantic-aware sampling to collect a high-quality random sample through a random walk based on KG embedding, followed by our unbiased (or, consistent) estimators for COUNT, SUM, AVG to compute the approximate aggregate results using the random sample, with a confidence interval-based accuracy guarantee. (2) AGQ supports interactive improvements of accuracy, complex queries with filter, GROUP-BY, MAX/MIN, and different graph shapes, e.g., chain, cycle, star, flower. (3) Its GUI helps users compare simple and complex aggregate queries, intermediate results as the queries progress, confidence intervals, relative errors, and various schemas for different valid answers in a user-friendly and interactive manner. Additionally, our system permits users to input queries in natural languages, keywords, or to select from a set of example graph queries.
更多查看译文
关键词
Knowledge graph, Approximate aggregate query, Random walk
AI 理解论文
溯源树
样例
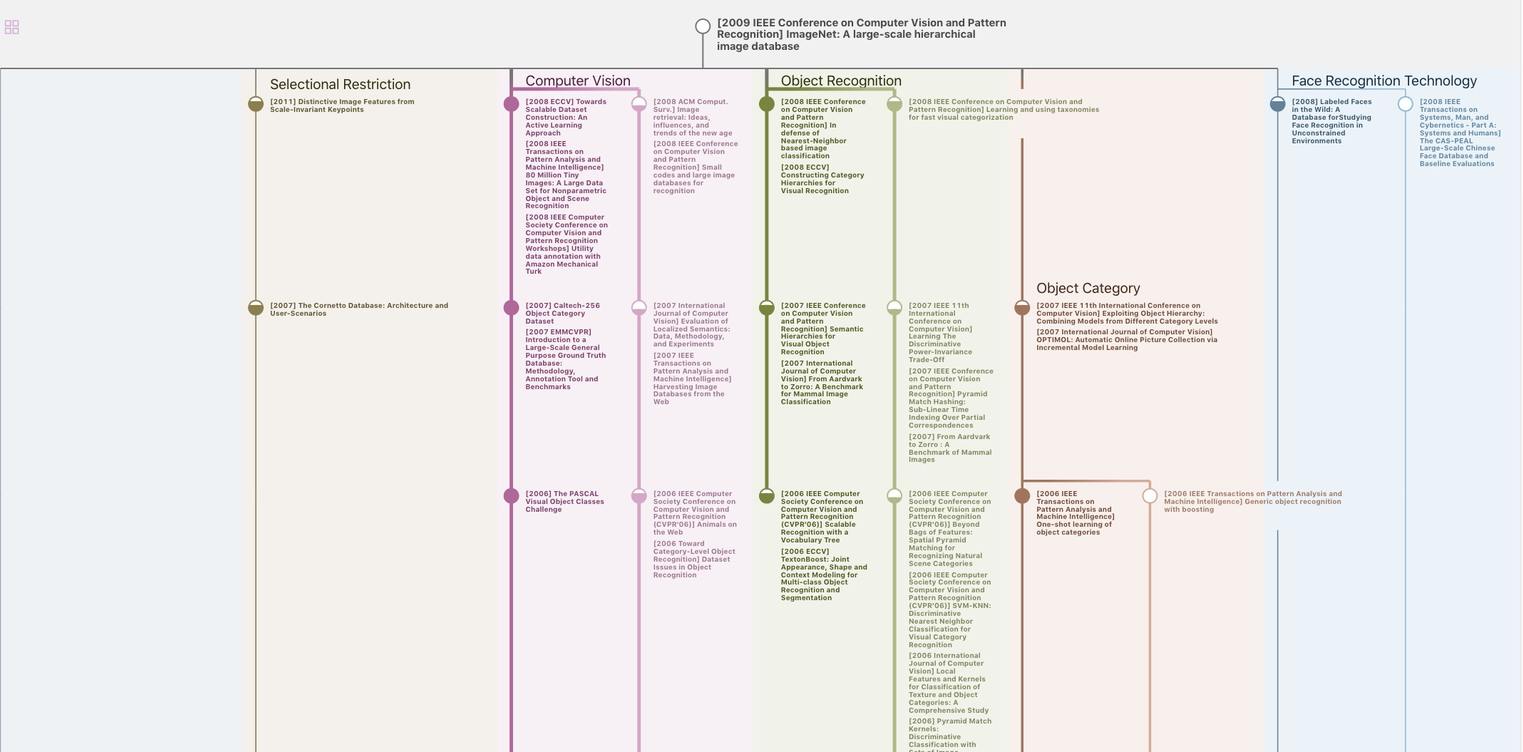
生成溯源树,研究论文发展脉络
Chat Paper
正在生成论文摘要