ED-AnoNet: Elastic Distortion-Based Unsupervised Network for OCT Image Anomaly Detection.
Lecture Notes in Computer Science(2022)
Abstract
The use of anomaly detection methods based on the deep convolutional neural network has shown its success on optical coherence tomography (OCT) images. However, these methods only train normal samples from healthy subjects, which are insensitive to abnormalities caused by small lesions, and are prone to missed diagnoses. To alleviate this shortcoming, this paper proposes an unsupervised anomaly detection network called ED-AnoNet, which is based on elastic distortion (ED), to improve OCT images anomaly detection. Different distortion fields are generated by adjusting the parameters of ED to simulate the damage of the hierarchical structure of the retina by the lesions. Subsequently, the normal OCT images are used to resample the distortion field to generate the pseudo abnormal OCT (Pseudo-abnorOCT) images and use them to train ED-AnoNet. On the spatial feature map, the spatial similarity retention loss function is used to increase the similarity between normal OCT and reconstructed images. Meanwhile, the spatial difference retention loss function is used to reduce the similarity between normal OCT and Pseudo-abnorOCT images. ED-AnoNet is promoted to extract more expressive feature maps, in which the anomaly detection performance can be improved. Results on public datasets show that the proposed method outperforms current state-of-the-art methods in terms of the area under the curve significantly.
MoreTranslated text
AI Read Science
Must-Reading Tree
Example
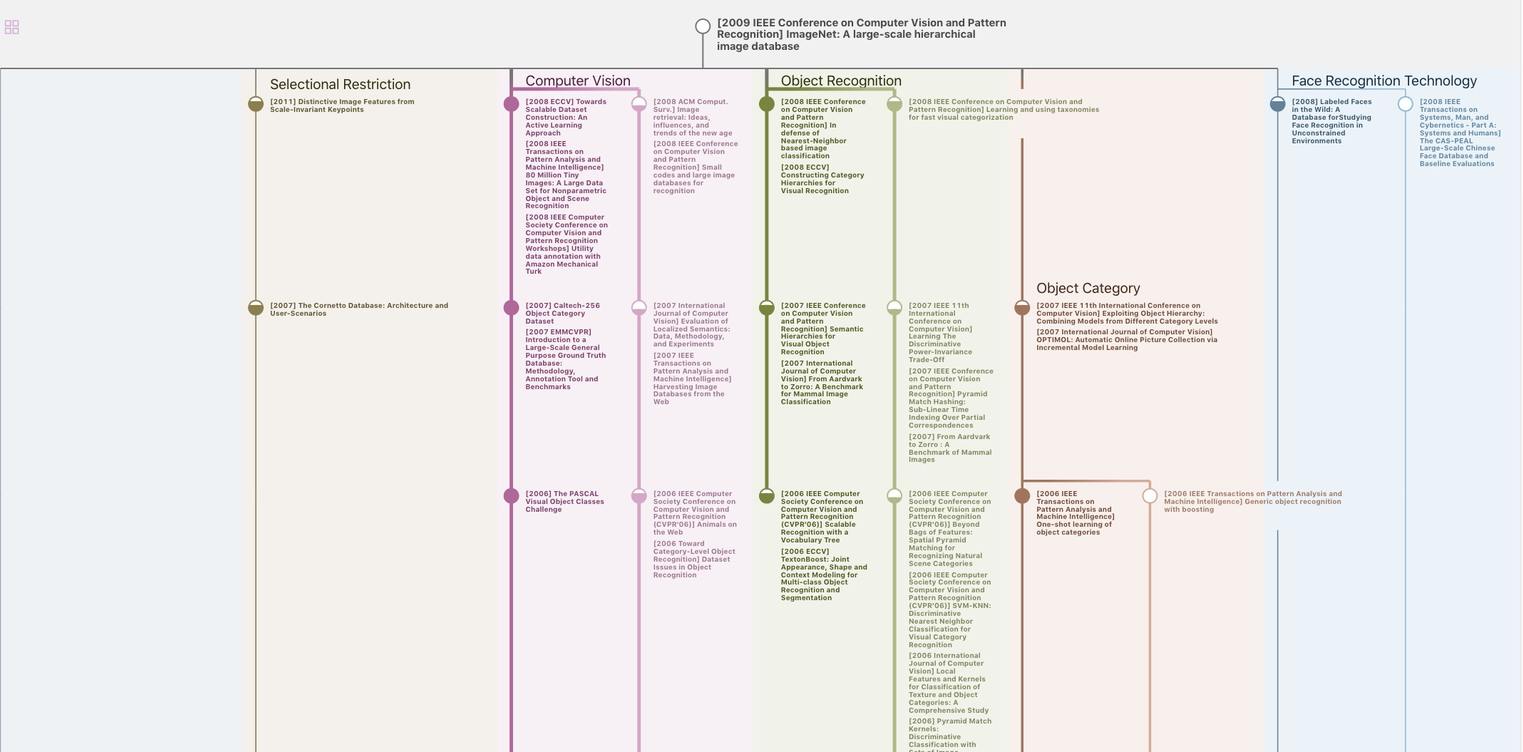
Generate MRT to find the research sequence of this paper
Chat Paper
Summary is being generated by the instructions you defined