A Visual Analytics Approach to Understanding Gradient Boosting Tree via Click Prediction on Ads.
Cooperative Design, Visualization, and Engineering (CDVE)(2022)
Abstract
As an iterative algorithm consisting of multiple decision trees, gradient boosting decision tree (GBDT) is widely used in problems such as classification and regression prediction. The ensemble decision trees of the algorithm obtain predictive effect by automatically filtering and combining new feature vectors, which contributes to discovering effective feature combinations. However, gradient boosting tree (GBT) is a tedious model, especially the boosting tree approach. It is difficult to interpret the principle of the model due to the characteristic of each tree of the model with weights and the unique structural properties of each decision tree, which is a challenge in many fields that require high interpretation such as financial risk control. In this paper, we design an interactive visual analytic system to solve this problem, to explain the structure and prediction process of the gradient boosting tree model, and to help experts in related fields to perform efficient analysis. We have designed a graphical representation of the feature information and a visual model of the boosting tree to show the basic mechanism of the GBT algorithm in a comprehensive way. The case study is conducted on the dataset of Kaggle competition to prove the effectiveness of the system.
MoreTranslated text
Key words
gradient boosting tree,click prediction,visual analytics approach,ads
AI Read Science
Must-Reading Tree
Example
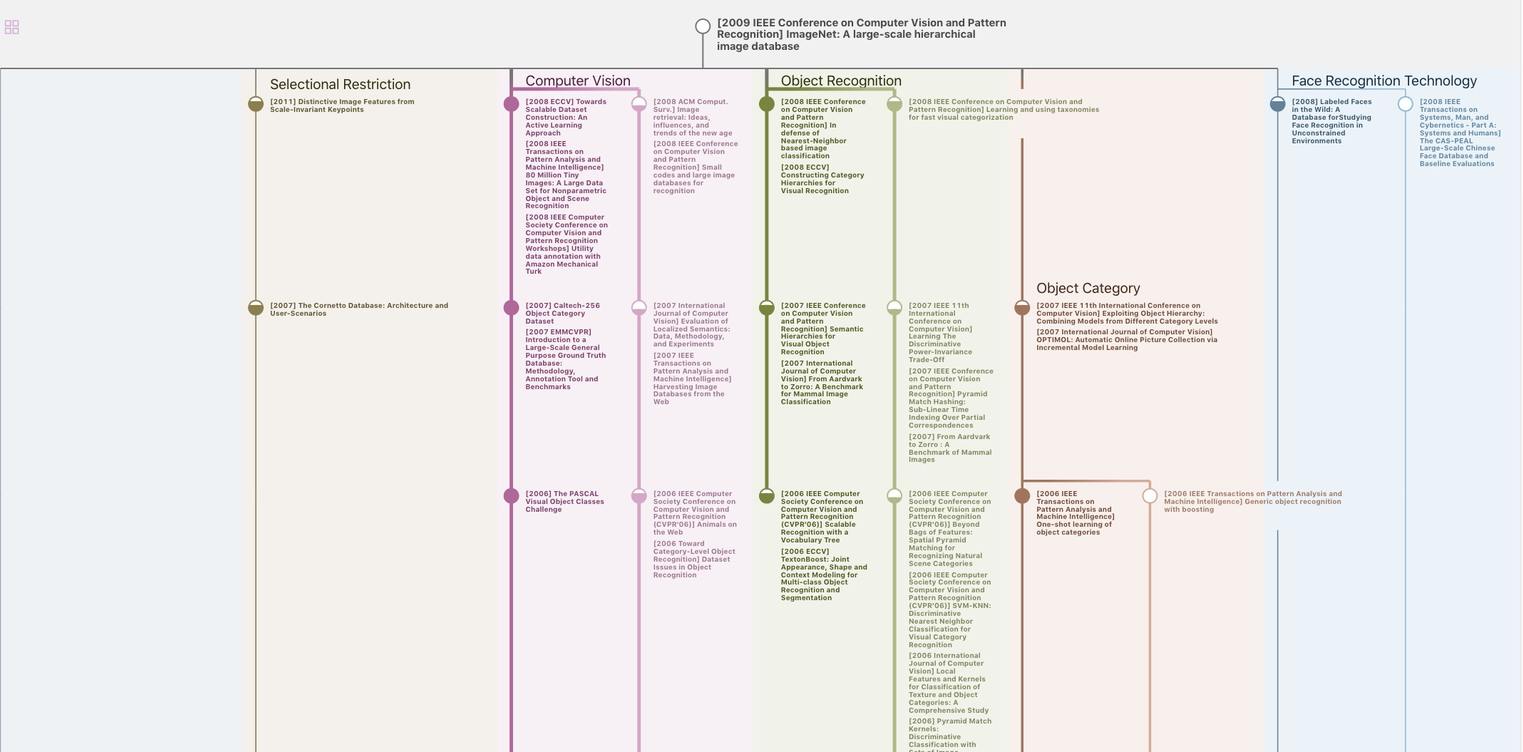
Generate MRT to find the research sequence of this paper
Chat Paper
Summary is being generated by the instructions you defined