Supervised Contrastive Learning for Cross-Lingual Transfer Learning.
China National Conference on Chinese Computational Linguistics (CCL)(2022)
Abstract
Multilingual pre-trained representations are not well-aligned by nature, which harms their performance on cross-lingual tasks. Previous methods propose to post-align the multilingual pre-trained representations by multi-view alignment or contrastive learning. However, we argue that both methods are not suitable for the cross-lingual classification objective, and in this paper we propose a simple yet effective method to better align the pre-trained representations. On the basis of cross-lingual data augmentations, we make a minor modification to the canonical contrastive loss, to remove false-negative examples which should not be contrasted. Augmentations with the same class are brought close to the anchor sample, and augmentations with different class are pushed apart. Experiment results on three cross-lingual tasks from XTREME benchmark show our method could improve the transfer performance by a large margin with no additional resource needed. We also provide in-detail analysis and comparison between different post-alignment strategies.
MoreTranslated text
Key words
transfer learning,contrastive learning,cross-lingual
AI Read Science
Must-Reading Tree
Example
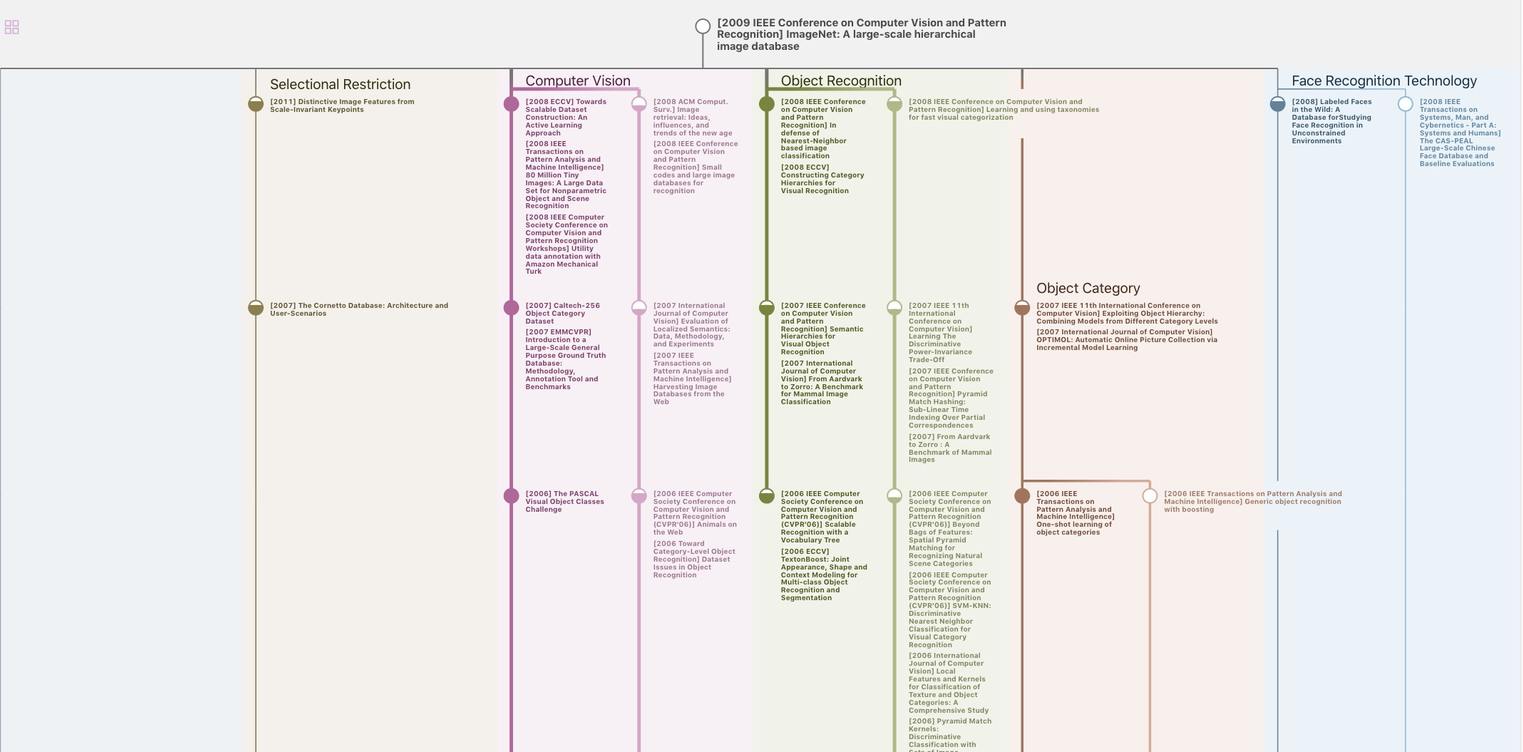
Generate MRT to find the research sequence of this paper
Chat Paper
Summary is being generated by the instructions you defined