Deep Reinforcement Learning toward Robust Multi-echelon Supply Chain Inventory Optimization.
IEEE Conference on Automation Science and Engineering (CASE)(2022)
摘要
Multi-echelon supply chains (SC) are highly complex systems with many inherent uncertainties, including customer demands and transportation time from one location to another. In addition to the complex SC structures, inventory decisions made at different stages affect each other. Maintaining global-level optimal inventory levels along the entire supply chain that is robust to changing business needs remains challenging. In this work, we have developed a simulation environment, GymSC, for reinforcement learning of multi-echelon SC inventory policies. Using a series of model-free deep reinforcement learning algorithms, we trained dynamic SC policies that have significantly improved performance when compared with popularly used heuristics. The robustness of the learned policy is demonstrated by its adaptability to a previously unseen environment with non-stationary customer demands. The presented work showcases the effectiveness and robustness of deep reinforcement learning in solving important practical SC optimization problems. In addition, we present this configurable simulation environment as a platform for testing existing and new algorithms to develop robust inventory policies with a goal to encourage further integration of deep reinforcement learning into the SC management problems as a promising alternative for addressing inventory optimization with uncertainties.
更多查看译文
关键词
deep reinforcement learning,supply,optimization,multi-echelon
AI 理解论文
溯源树
样例
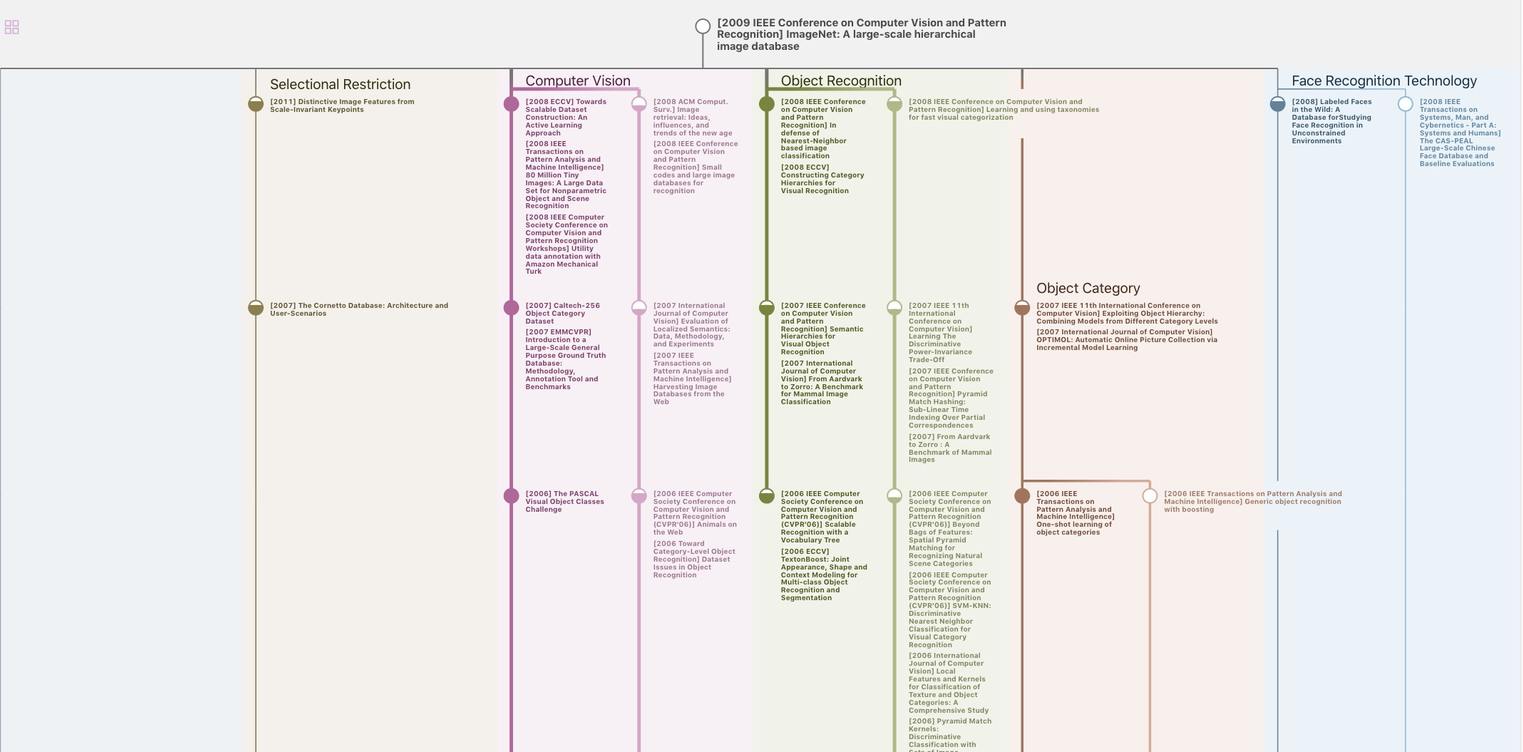
生成溯源树,研究论文发展脉络
Chat Paper
正在生成论文摘要