Generating Counterfactual Explanations For Causal Inference in Breast Cancer Treatment Response.
IEEE Conference on Automation Science and Engineering (CASE)(2022)
Abstract
Imaging phenotypes extracted via radiomics of magnetic resonance imaging has shown great potential at predicting the treatment response in breast cancer patients after administering neoadjuvant systemic therapy (NST). Existing machine learning models are, however, limited in providing an expert-level interpretation of these models, particularly interpretability towards generating causal inference. Causal relationships between imaging phenotypes, clinical information, molecular features, and the treatment response may be useful in guiding the treatment strategies, management plans, and gaining acceptance in medical communities. In this work, we leverage the concept of counterfactual explanations to extract causal relationships between various imaging phenotypes, clinical information, molecular features, and the treatment response after NST. We implement the methodology on a publicly available breast cancer dataset and demonstrate the causal relationships generated from counterfactual explanations. We also compare and contrast our results with traditional explanations, such as LIME and Shapley.
MoreTranslated text
Key words
radiomics,counterfactual explanations,magnetic resonance imaging,machine learning
AI Read Science
Must-Reading Tree
Example
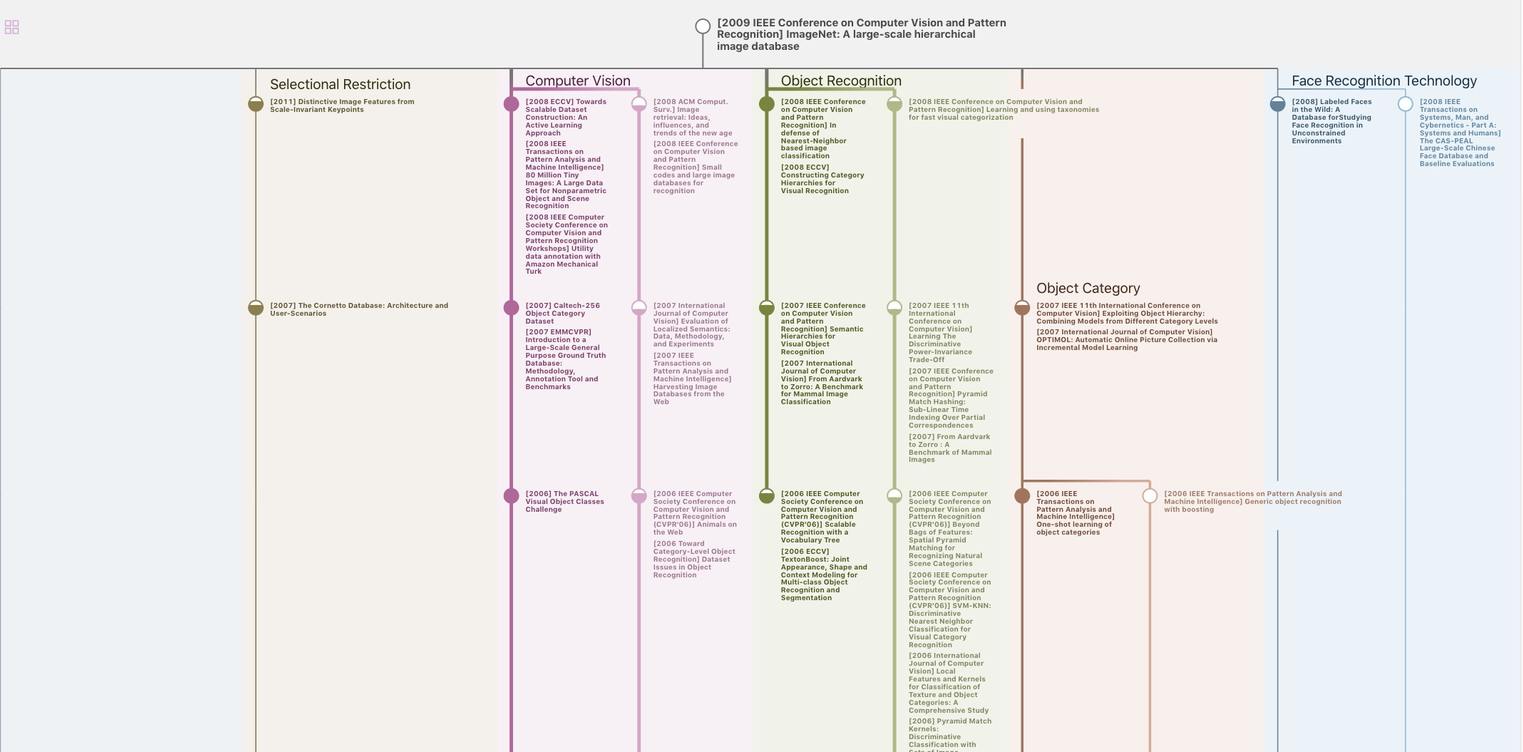
Generate MRT to find the research sequence of this paper
Chat Paper
Summary is being generated by the instructions you defined