Multiple imputation based on Bayesian principal component analysis and bootstrap.
Asian Control Conference (ASCC)(2022)
Abstract
In this paper, a multiple imputation algorithm based on Bayesian principal component analysis (BPCA) and bootstrap is proposed for data filling in time series. Firstly, we need to sample n times with replacement through the bootstrap method. Then, the Bayesian method combined with principal component analysis (PCA) and the Expectation Maximization (EM) algorithm is utilized to estimate the model parameters and missing points for each data subset. Finally, the final filling value can be obtained by comprehensive analysis of these groups of filling values. To verify the effectiveness of the proposed BPCAMI method, four data sets are employed in this paper. Experimental results show that the imputation effect of the proposed method is better than that of the existing methods.
MoreTranslated text
Key words
missing data,multiple imputation,Bayesian principal component analysis,bootstrap
AI Read Science
Must-Reading Tree
Example
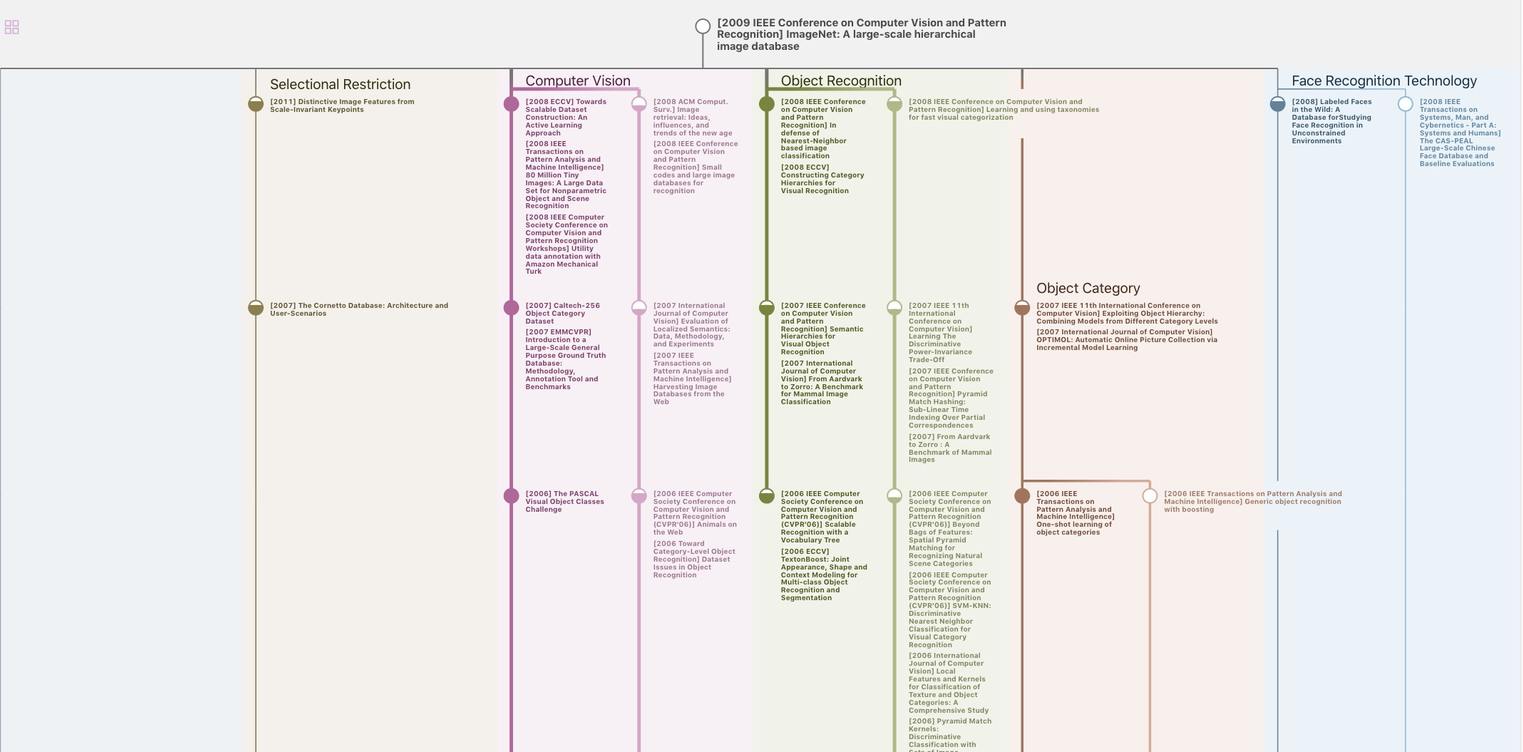
Generate MRT to find the research sequence of this paper
Chat Paper
Summary is being generated by the instructions you defined