Bayesian model selection for reducing bloat and overfitting in genetic programming for symbolic regression.
Annual Conference on Genetic and Evolutionary Computation (GECCO)(2022)
摘要
When performing symbolic regression using genetic programming, overfitting and bloat can negatively impact generalizability and interpretability of the resulting equations as well as increase computation times. A Bayesian fitness metric is introduced and its impact on bloat and overfitting during population evolution is studied and compared to common alternatives in the literature. The proposed approach was found to be more robust to noise and data sparsity in numerical experiments, guiding evolution to a level of complexity appropriate to the dataset. Further evolution of the population resulted not in overfitting or bloat, but rather in slight simplifications in model form. The ability to identify an equation of complexity appropriate to the scale of noise in the training data was also demonstrated. In general, the Bayesian model selection algorithm was shown to be an effective means of regularization which resulted in less bloat and overfitting when any amount of noise was present in the training data.
更多查看译文
AI 理解论文
溯源树
样例
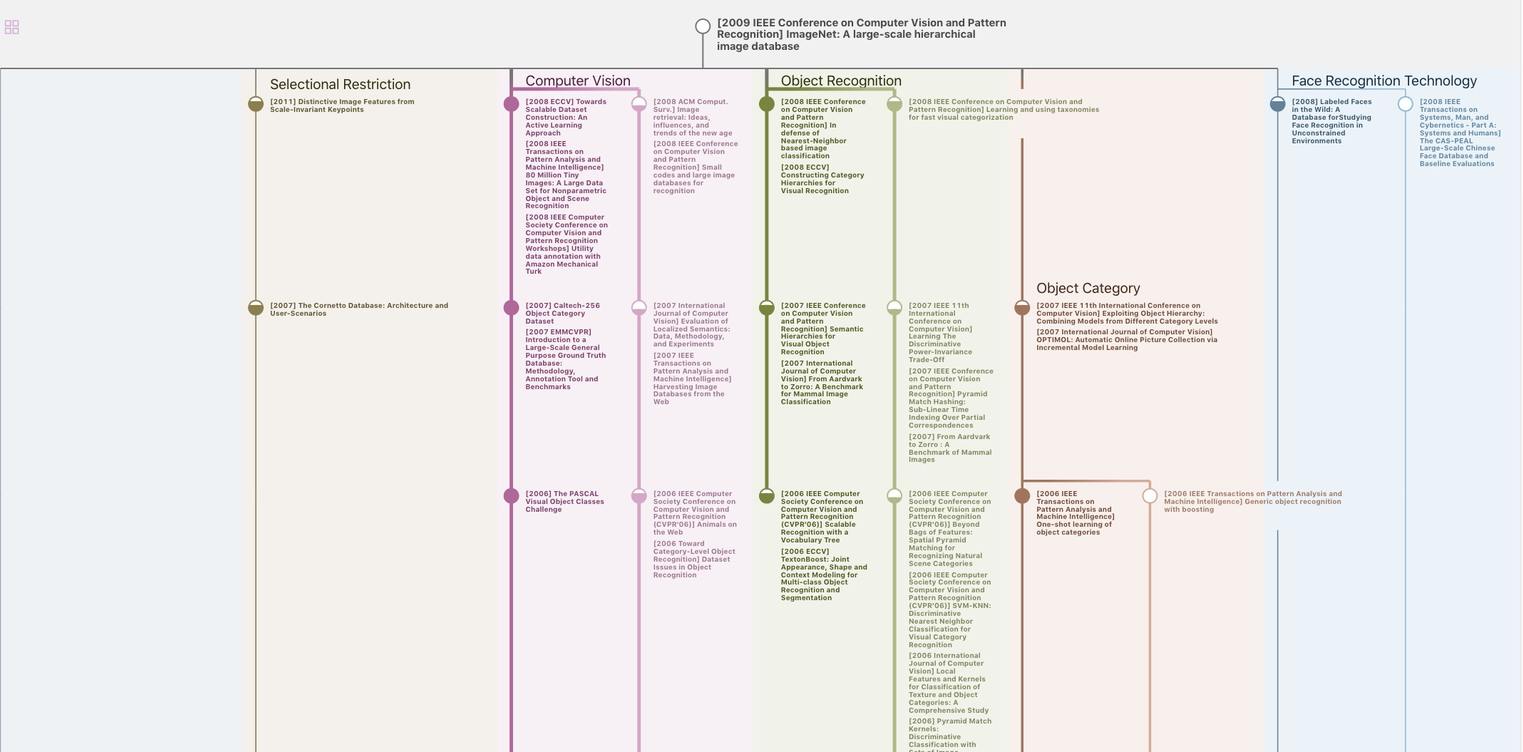
生成溯源树,研究论文发展脉络
Chat Paper
正在生成论文摘要