Evolutionary approach to model calibration with uncertainty: an application to breast cancer growth model.
Annual Conference on Genetic and Evolutionary Computation (GECCO)(2022)
Abstract
Dynamical systems in most scientific areas can be modelled using ordinary differential equations or difference equations. However, when analysing and simulating real-world phenomena, model and data uncertainty arises, mainly due to the exclusion from the model of minor aspects of the phenomenon or experimental measurement error in the data. Therefore, more and more models take uncertainty and its quantification into account. One type of such models are random models, in which their parameters are assumed to be random variables. However, this approach to capturing the uncertainty of phenomena has so far been little employed in real-world problems, both because of its inherent difficulty and the lack of data to support the correct choice of model parameter distributions. This problem can be addressed by designing probabilistic calibration algorithms capable of finding distributions of model parameters that explain/capture data uncertainty. In the latter sense, here we propose a technique based on the multi-objective particle swarm optimisation (MOPSO) algorithm to find the parameter probability distributions of a random model that capture the uncertainty of the data. To illustrate the method in a real application, a complete analysis is performed on a simple first-order difference model for breast cancer tumour growth.
MoreTranslated text
Key words
Uncertainty quantification, Random models, Probabilistic calibration, Multi-Objective Particle Swarm Optimization (MOPSO), Breast cancer growth
AI Read Science
Must-Reading Tree
Example
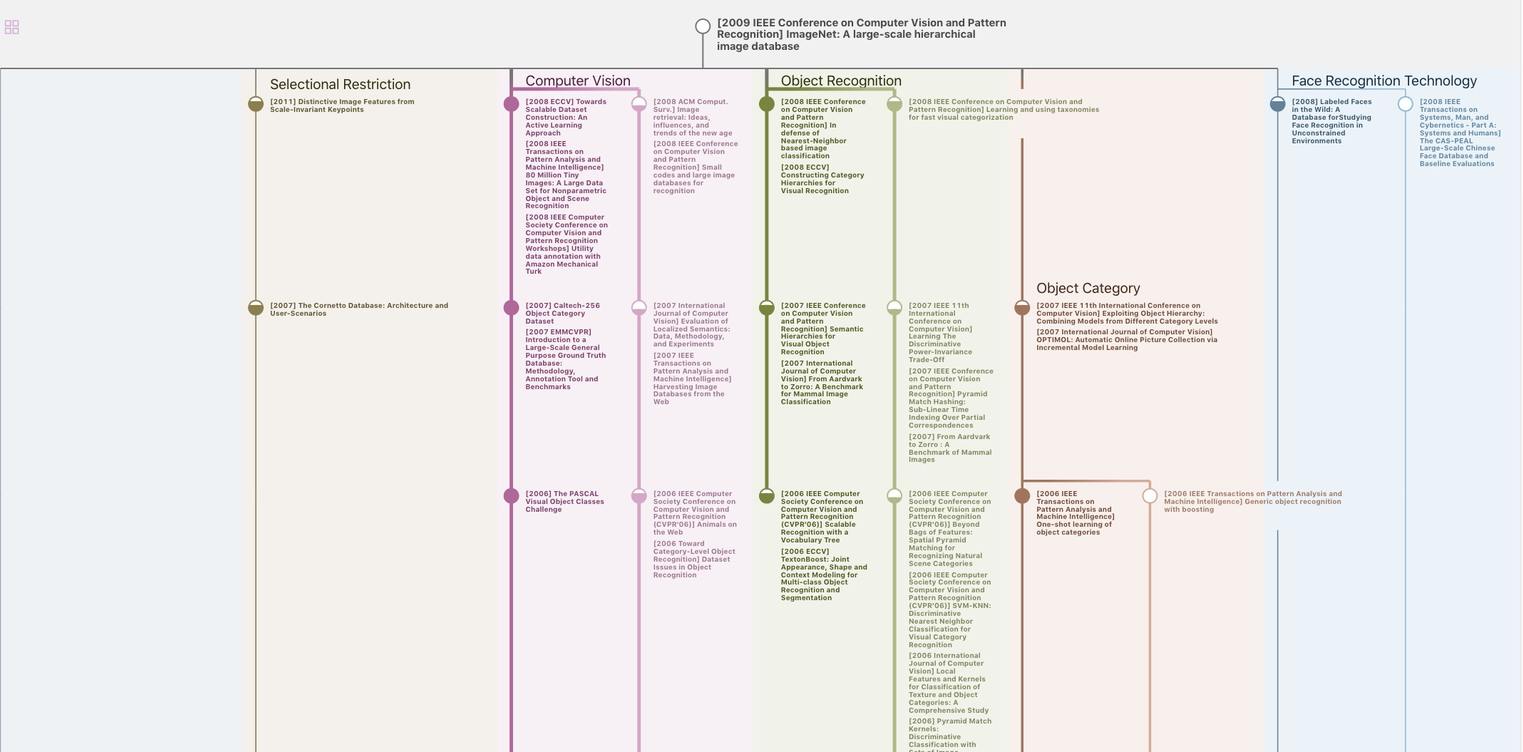
Generate MRT to find the research sequence of this paper
Chat Paper
Summary is being generated by the instructions you defined