Empirical linkage learning for non-binary discrete search spaces in the optimization of a large-scale real-world problem.
Annual Conference on Genetic and Evolutionary Computation (GECCO)(2022)
摘要
Empirical linkage learning (ELL) is a new type of problem decomposition techniques proposed recently for Black-box optimization. ELL techniques are proven never to report false gene dependencies (denoted as false linkage ), which is their main advantage. The linkage learning based on local optimization (3LO) (the first ELL technique proposed) was shown to bring a significant potential of reaching high-quality results. However, 3LO applies only to binary search spaces, this paper examines the 3LO for discrete non-binary search spaces (3LO-nb). 3LO-nb applies to any problem using a discrete encoding. On the base of NP-hard, large-scale, real-world problem, we show that using 3LO-nb may lead to outstanding results.
更多查看译文
关键词
Genetic Algorithms, Linkage learning, Empirical linkage learning, Large-scale global optimization
AI 理解论文
溯源树
样例
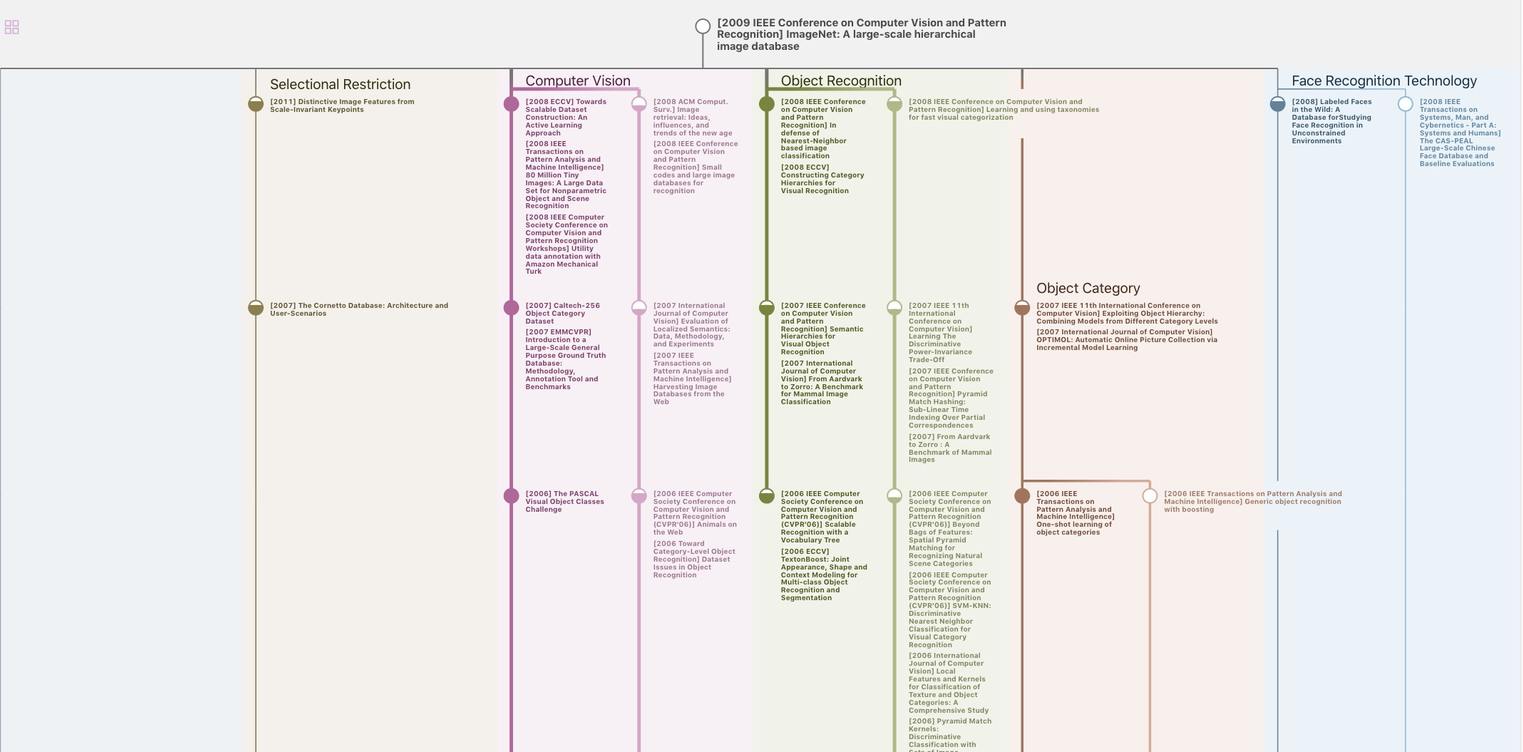
生成溯源树,研究论文发展脉络
Chat Paper
正在生成论文摘要