Indoor Scene Classification Algorithm Based on an Object Vector for Robot Applications.
International Conference on Artificial Intelligence in Electronics Engineering (AIEE)(2022)
摘要
At present, many indoor scene classification algorithms are based on abstract feature descriptions and lack semantic interpretation for classification results. For robot applications, these classification methods fail to reuse prior knowledge of environmental objects, resulting in a waste of computing resources, and are not conducive to interaction. In addition, a large number of existing methods have not been verified for robot applications because training and test are performed on the same dataset. Here, intermediate semantic structure, called object vector, is proposed to describe the scene. The recognition confidence, category, and quantity of typical objects, which are related to scene concepts, can be captured by this structure. Based on the object vector, the semantic prototype models of scene categories are obtained using the prototype learning method, and the corresponding prototype confidence vector is generated for scene prediction. The object vector and prototype confidence vector are concatenated to form a complete descriptor. Applying sparse random forest learning, scene conceptual models are formed and used in real scene classification. In the experiment, the training and test sets were selected from sets with different styles. The average accuracy on the MIT Indoor 67, VPC, and Tsotsos_home datasets is higher than that of fine-tuned VGG. In particular, the accuracy on the VPC dataset improved by more than 5%. Moreover, the algorithm performance is evaluated on an actual robot platform.
更多查看译文
AI 理解论文
溯源树
样例
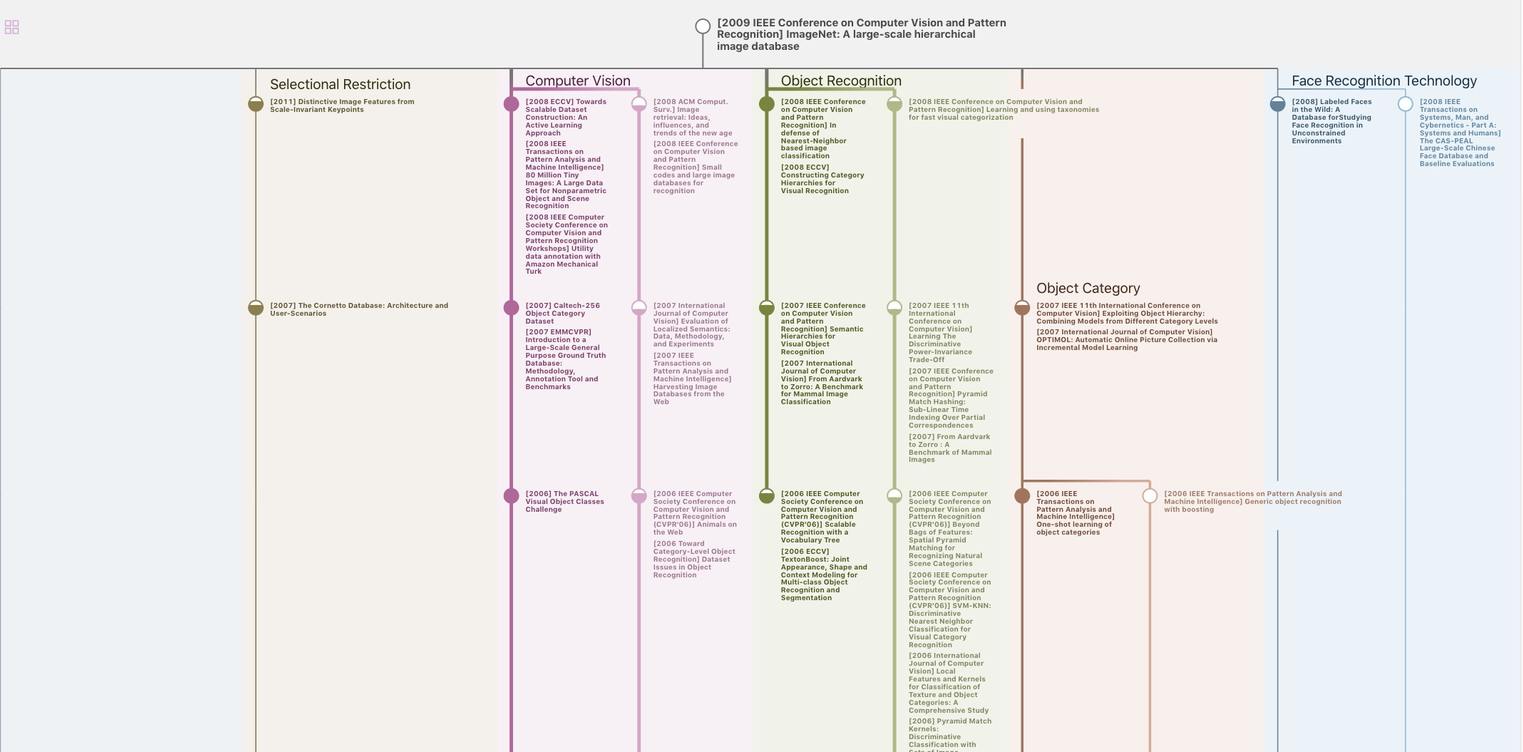
生成溯源树,研究论文发展脉络
Chat Paper
正在生成论文摘要