Bridging Automated to Autonomous Cyber Defense: Foundational Analysis of Tabular Q-Learning.
Workshop on Security and Artificial Intelligence (AISec)(2022)
摘要
Leveraging security automation and orchestration technologies enables security analysts to respond more quickly and accurately to threats. However, current tooling is limited to automating very finely scoped and hand-coded situations, such as quarantining known malware and blocking traffic from known malicious domains. Recent research has sought to bridge the gap between this kind of automated security and autonomous cyber defense, leveraging reinforcement learning (RL) on top of basic automation to enable intelligent response. This paper provides foundational analysis of autonomous agents trained with Tabular Q-Learning through a series of experiments examining a range of network scenarios. Our results demonstrate that off-the-shelf Tabular Q-Learning does not offer a single, superior solution across all scenarios. However, we also find that modifying the underlying state encoding and update function can influence the robustness of the defensive agent to generalize to unseen evaluation environments without a significant loss in accuracy. These results highlight potential optimizations for more advanced RL techniques as well as provide a baseline for others leveraging RL for defensive cyber automation.
更多查看译文
AI 理解论文
溯源树
样例
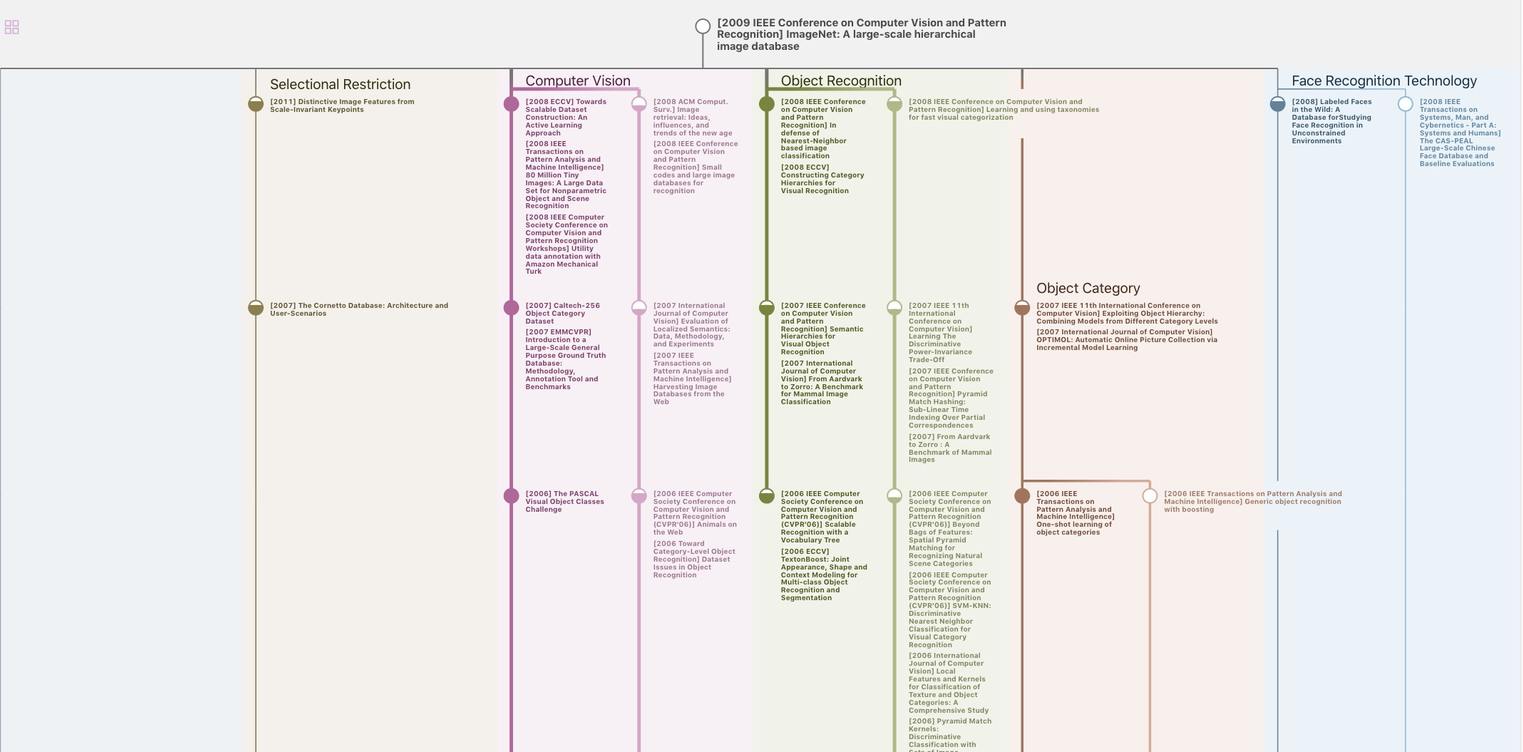
生成溯源树,研究论文发展脉络
Chat Paper
正在生成论文摘要