Variable size segmentation for efficient representation and querying of non-uniform time series datasets.
ACM Symposium on Applied Computing (SAC)(2022)
Abstract
Existing approaches for time series similarity computing are the core of many data analytics tasks. Given the considered data volumes, or simply the need for fast response times, they often rely on shorter representations, usually with information loss. This incurs approximate comparisons where precision is a major issue. We present and experimentally evaluate ASAX, a new approach for segmenting time series before their transformation into symbolic representations. ASAX reduces significantly the information loss incurred by possible splittings at different steps of the representation calculation, particularly for datasets with unbalanced (nonuniform) distributions. We provide theoretical guarantees on the lower bound of similarity measures, and our experiments illustrate that our method outperforms the state of the art, with significant gain in precision for datasets with unbalanced distributions.
MoreTranslated text
Key words
Time Series, Representations, Information Retrieval
AI Read Science
Must-Reading Tree
Example
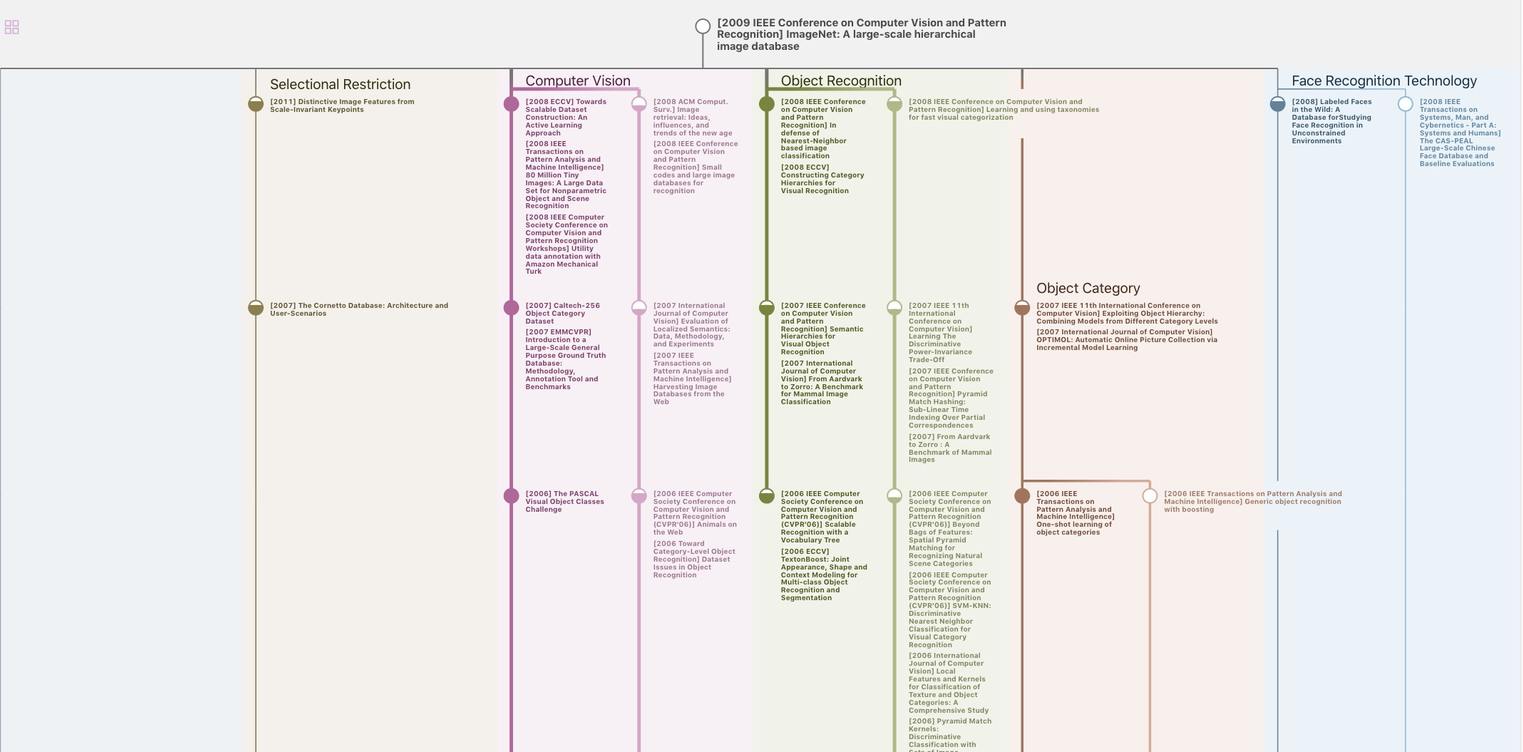
Generate MRT to find the research sequence of this paper
Chat Paper
Summary is being generated by the instructions you defined